Leave-one-out cross-validation is risk consistent for lasso
Machine Learning(2014)
摘要
The lasso procedure pervades the statistical and signal processing literature, and as such, is the target of substantial theoretical and applied research. While much of this research focuses on the desirable properties that lasso possesses—predictive risk consistency, sign consistency, correct model selection—these results assume that the tuning parameter is chosen in an oracle fashion. Yet, this is impossible in practice. Instead, data analysts must use the data twice, once to choose the tuning parameter and again to estimate the model. But only heuristics have ever justified such a procedure. To this end, we give the first definitive answer about the risk consistency of lasso when the smoothing parameter is chosen via cross-validation. We show that under some restrictions on the design matrix, the lasso estimator is still risk consistent with an empirically chosen tuning parameter.
更多查看译文
关键词
Stochastic equicontinuity,Uniform convergence,Persistence
AI 理解论文
溯源树
样例
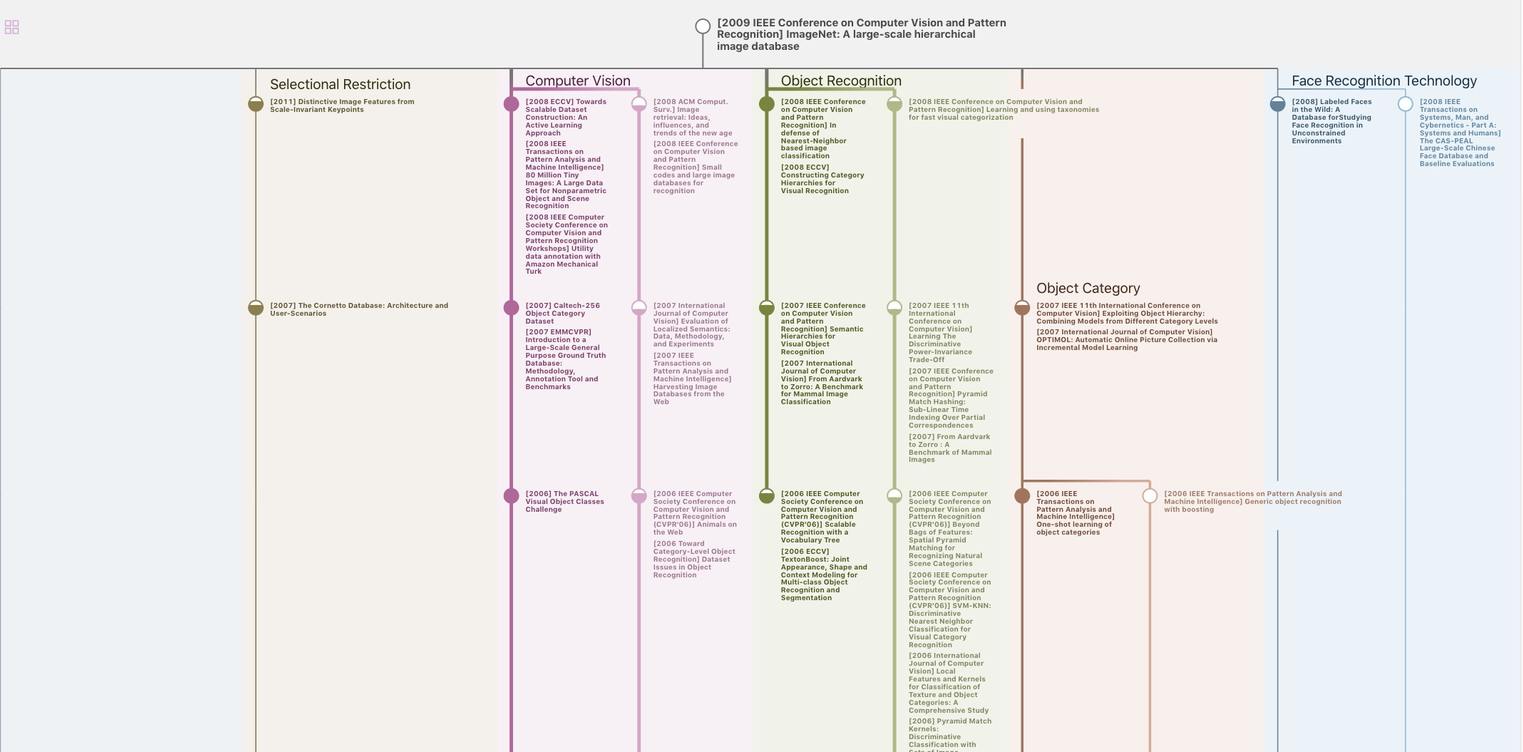
生成溯源树,研究论文发展脉络
Chat Paper
正在生成论文摘要