Support Vector Domain Description with Maximum Between Spheres Separability.
JOURNAL OF INFORMATION SCIENCE AND ENGINEERING(2014)
摘要
Support Vector Domain Description (SVDD) is inspired by the Support Vector Classifier. It obtains a sphere shaped decision boundary with minimal volume around a dataset. This data description can be used for novelty or outlier detection. Our approach is always to minimize the volume of the sphere describing the dataset, while at the same time maximize the separability between the spheres. To build such sphere we only need to solve a convex quadratic optimization problem that can be efficiently solved with the existing software packages, simulation results on seventeen benchmark datasets have successfully validated the effectiveness of the proposed method.
更多查看译文
关键词
support vector domain description,small hypersphere,maximum separability,feature space,convex quadratic programming problem
AI 理解论文
溯源树
样例
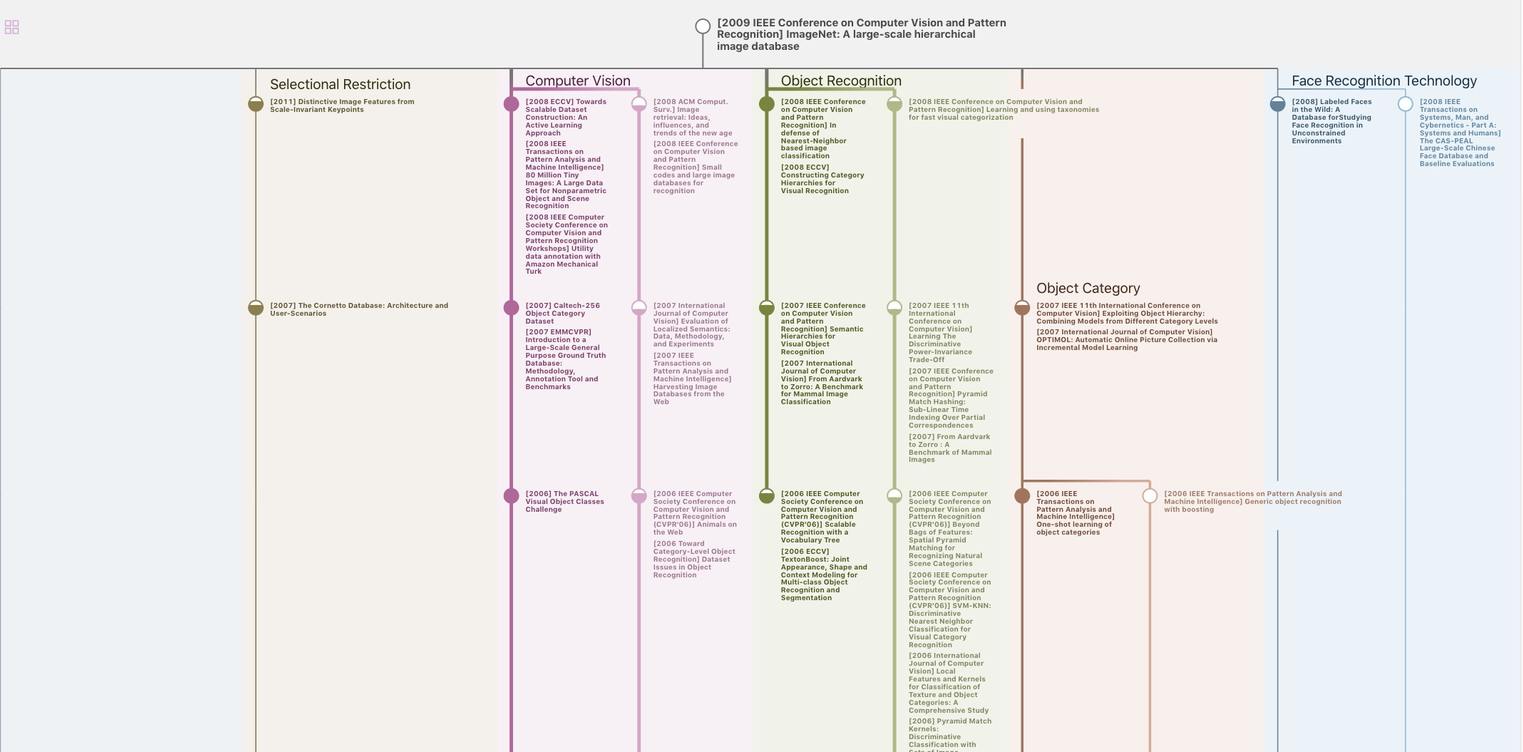
生成溯源树,研究论文发展脉络
Chat Paper
正在生成论文摘要