On feature extraction for noninvasive kernel estimation of left ventricular chamber function indices from echocardiographic images.
Digital Signal Processing(2015)
摘要
Two reference indices used to characterize left ventricular (LV) global chamber function are end-systolic peak elastance (Emax) and the time-constant of relaxation rate (τ). However, these two indices are very difficult to obtain in the clinical setting as they require invasive high-fidelity catheterization procedures. We have previously demonstrated that it is possible to approximate these indices noninvasively by digital processing color-Doppler M-mode (CDMM) images. The aim of the present study was twofold: (1) to study which feature extraction from linearly reduced input spaces yields the most useful information for the prediction of the haemodynamic variables from CDMM images; (2) to verify whether the use of nonlinear versions of those linear methods actually improves the estimation. We studied the performance and interpretation of different linearly transformed input spaces (raw image, discrete cosine transform (DCT) coefficients, partial least squares, and principal components regression), and we compared whether nonlinear versions of the above methods provided significant improvement in the estimation quality. Our results showed that very few input features suffice for providing a good (medium) quality estimator for Emax (for τ), which can be readily interpreted in terms of the measured flows. Additional covariates should be included to improve the prediction accuracy of both reference indices, but especially in τ models. The use of efficient nonlinear kernel algorithms does improve the estimation quality of LV indices from CDMM images when using DCT input spaces that capture almost all energy.
更多查看译文
关键词
Color Doppler M-mode imaging,Kernel methods,Left ventricular function,Elastance,LV relaxation,Cosine transform
AI 理解论文
溯源树
样例
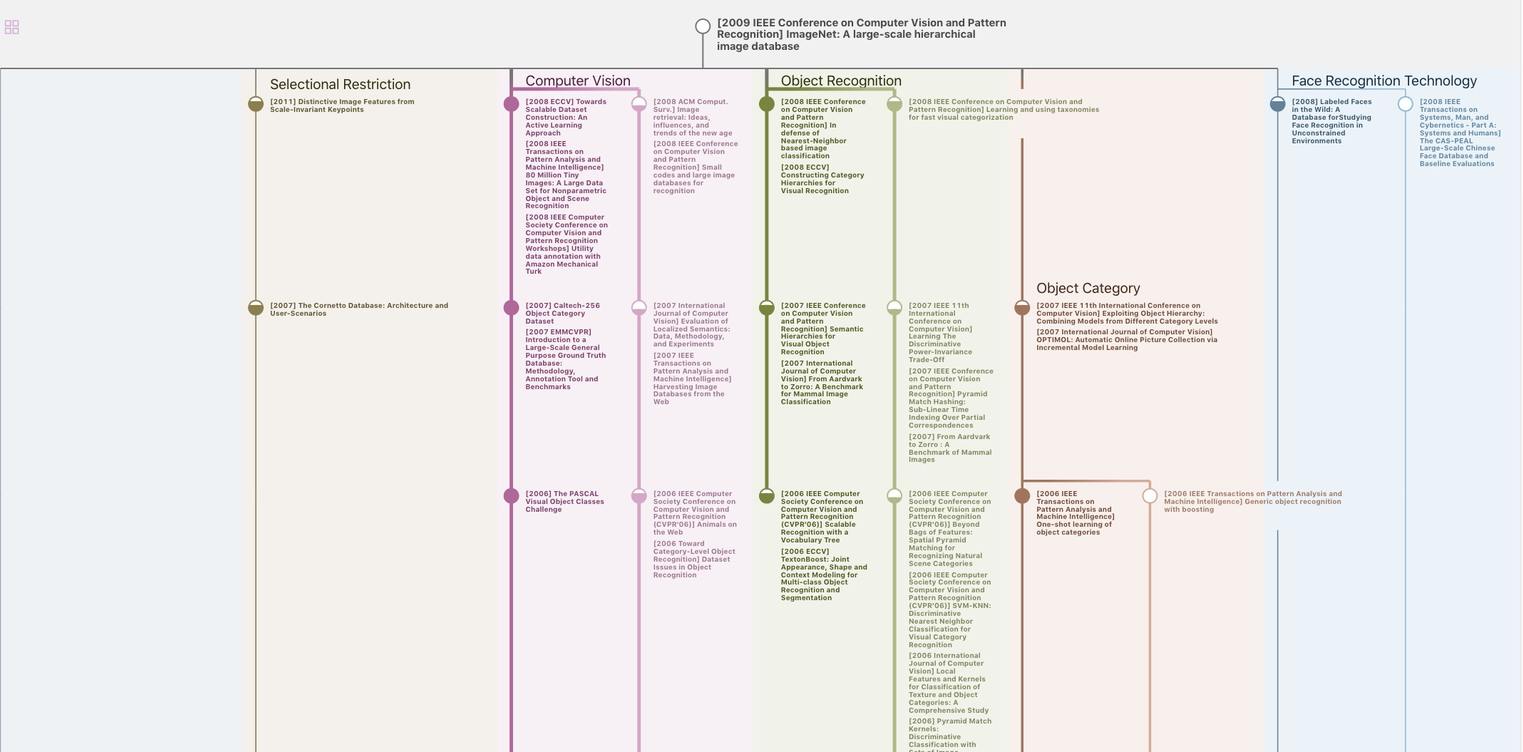
生成溯源树,研究论文发展脉络
Chat Paper
正在生成论文摘要