Shrinkage Linear and Widely Linear Complex-Valued Least Mean Squares Algorithms for Adaptive Beamforming
IEEE Transactions on Signal Processing(2015)
摘要
In this paper, shrinkage linear complex-valued least mean squares (SL-CLMS) and shrinkage widely linear complex-valued least mean squares (SWL-CLMS) algorithms are devised for adaptive beamforming. By exploiting the relationship between the noise-free a posteriori and a priori error signals, the SL-CLMS method is able to provide a variable step size to update the weight vector for the adaptive beamformer, significantly enhancing the convergence speed and decreasing the steady-state misadjustment. On the other hand, besides adopting a variable step size determined by minimizing the square of the augmented noise-free a posteriori errors, the SWL-CLMS approach exploits the noncircular properties of the signal of interest, which considerably improves the steady-state performance. Simulation results are presented to illustrate their superiority over the CLMS, complex-valued normalized LMS, variable step size, recursive least squares (RLS) algorithms and their corresponding widely linear-based schemes. Additionally, our proposed algorithms are more computationally efficient than the RLS solutions though they may have a slightly slower convergence rate.
更多查看译文
关键词
LMS ALGORITHM,NONCIRCULAR INTERFERENCES,SIGNALS,CONVERGENCE
AI 理解论文
溯源树
样例
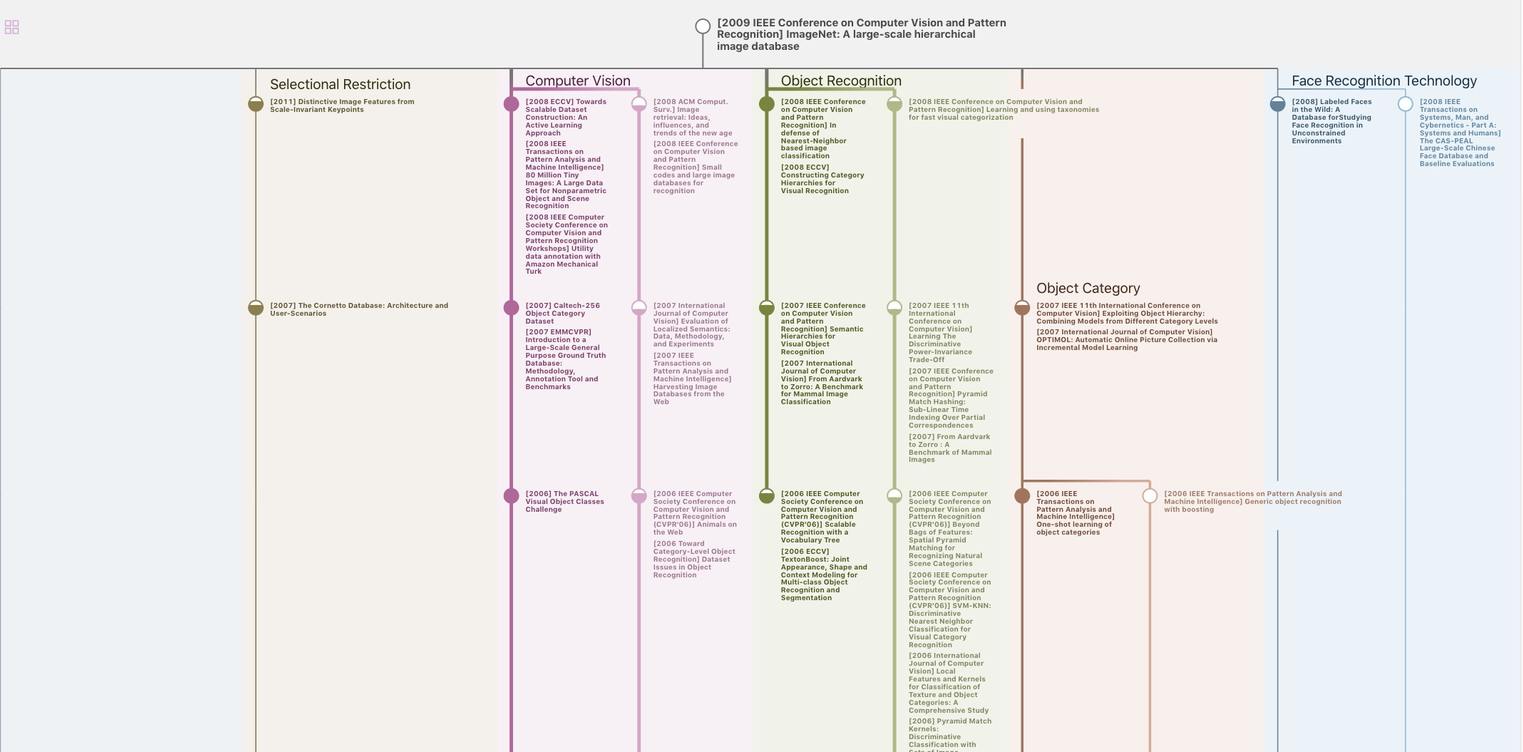
生成溯源树,研究论文发展脉络
Chat Paper
正在生成论文摘要