Robust Topological Inference: Distance To a Measure and Kernel Distance.
JOURNAL OF MACHINE LEARNING RESEARCH(2017)
摘要
Let P be a distribution with support S. The salient features of S can be quantified with persistent homology, which summarizes topological features of the sublevel sets of the distance function (the distance of any point x to S). Given a sample from P we can infer the persistent homology using an empirical version of the distance function. However, the empirical distance function is highly non-robust to noise and outliers. Even one outlier is deadly. The distance-to-a-measure (DTM), introduced by Chazal et al. (2011), and the kernel distance, introduced by Phillips et al. (2014), are smooth functions that provide useful topological information but are robust to noise and outliers. Chazal et al. (2015) derived concentration bounds for DTM. Building on these results, we derive limiting distributions and confidence sets, and we propose a method for choosing tuning parameters.
更多查看译文
关键词
Topological data analysis,persistent homology,RKHS
AI 理解论文
溯源树
样例
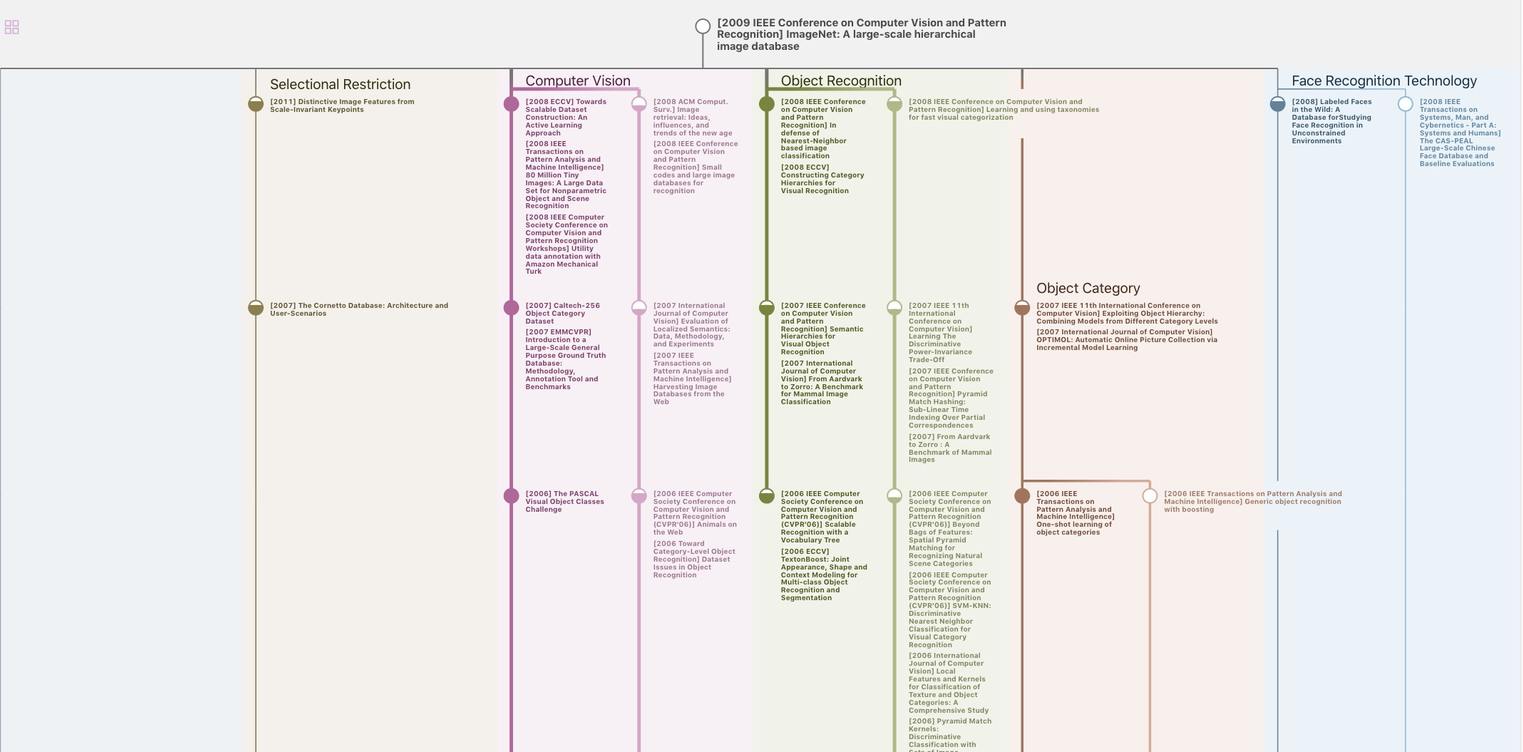
生成溯源树,研究论文发展脉络
Chat Paper
正在生成论文摘要