Smoothing Method For Improved Minimum Phone Error Linear Regression
IEICE TRANSACTIONS ON INFORMATION AND SYSTEMS(2014)
摘要
A smoothing method for minimum phone error linear regression (MPELR) is proposed in this paper. We show that the objective function for minimum phone error (MPE) can be combined with a prior mean distribution. When the prior mean distribution is based on maximum likelihood (ML) estimates, the proposed method is the same as the previous smoothing technique for MPELR. Instead of ML estimates, maximum a posteriori (MAP) parameter estimate is used to define the mode of prior mean distribution to improve the performance of MPELR. Experiments on a large vocabulary speech recognition task show that the proposed method can obtain 8.4% relative reduction in word error rate when the amount of data is limited, while retaining the same asymptotic performance as conventional MPELR. When compared with discriminative maximum a posteriori linear regression (DMAPLR), the proposed method shows improvement except for the case of limited adaptation data for supervised adaptation.
更多查看译文
关键词
speaker adaptation (SA), maximum likelihood linear regression (MLLR), maximum a posteriori linear regression (MAPLR), minimum phone error linear regression (MPELR), discriminative maximum a posteriori linear regression (DMAPLR)
AI 理解论文
溯源树
样例
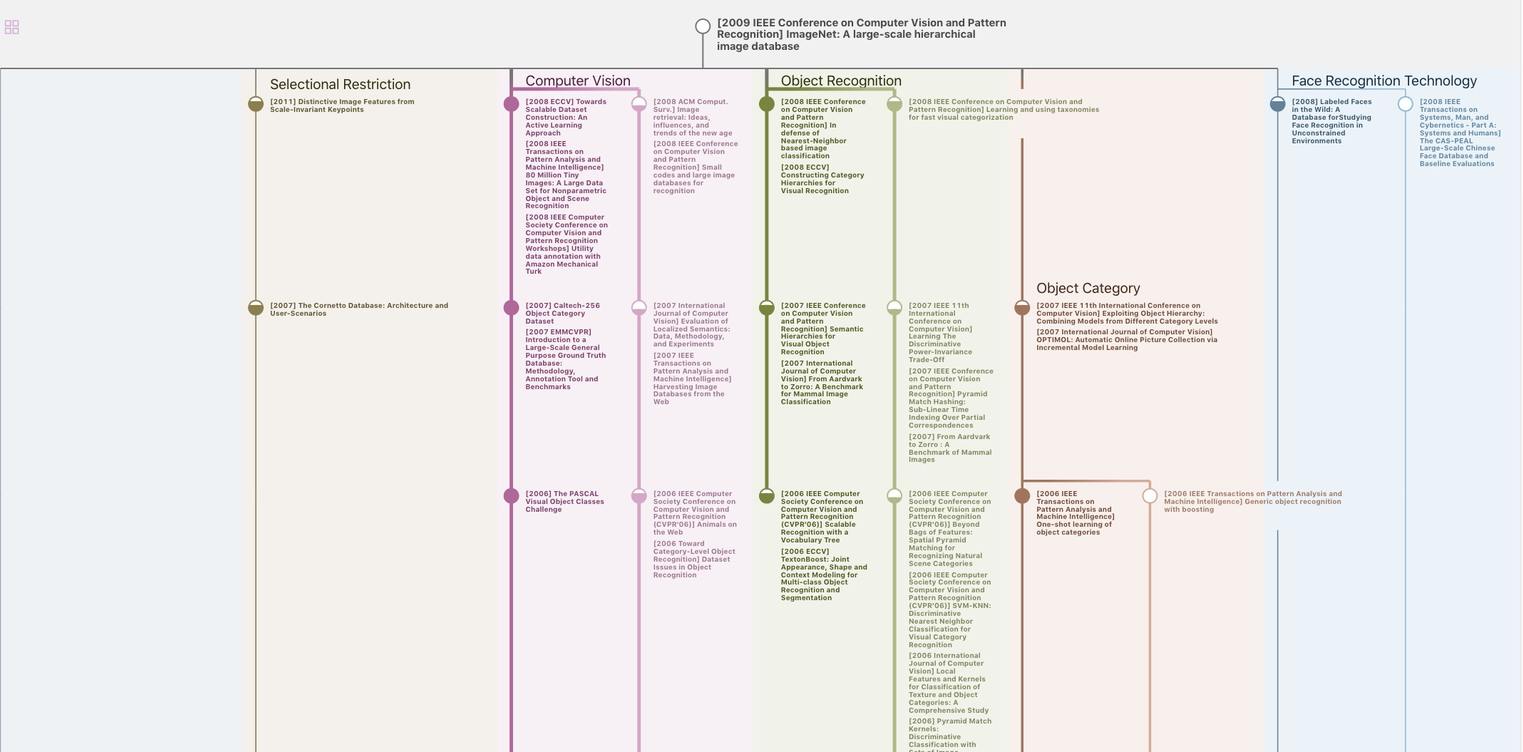
生成溯源树,研究论文发展脉络
Chat Paper
正在生成论文摘要