Feature selection for linear SVMs under uncertain data: Robust optimization based on difference of convex functions algorithms
Neural Networks(2014)
摘要
In this paper, we consider the problem of feature selection for linear SVMs on uncertain data that is inherently prevalent in almost all datasets. Using principles of Robust Optimization, we propose robust schemes to handle data with ellipsoidal model and box model of uncertainty. The difficulty in treating @?"0-norm in feature selection problem is overcome by using appropriate approximations and Difference of Convex functions (DC) programming and DC Algorithms (DCA). The computational results show that the proposed robust optimization approaches are superior than a traditional approach in immunizing perturbation of the data.
更多查看译文
关键词
feature selection,svm,robust optimization
AI 理解论文
溯源树
样例
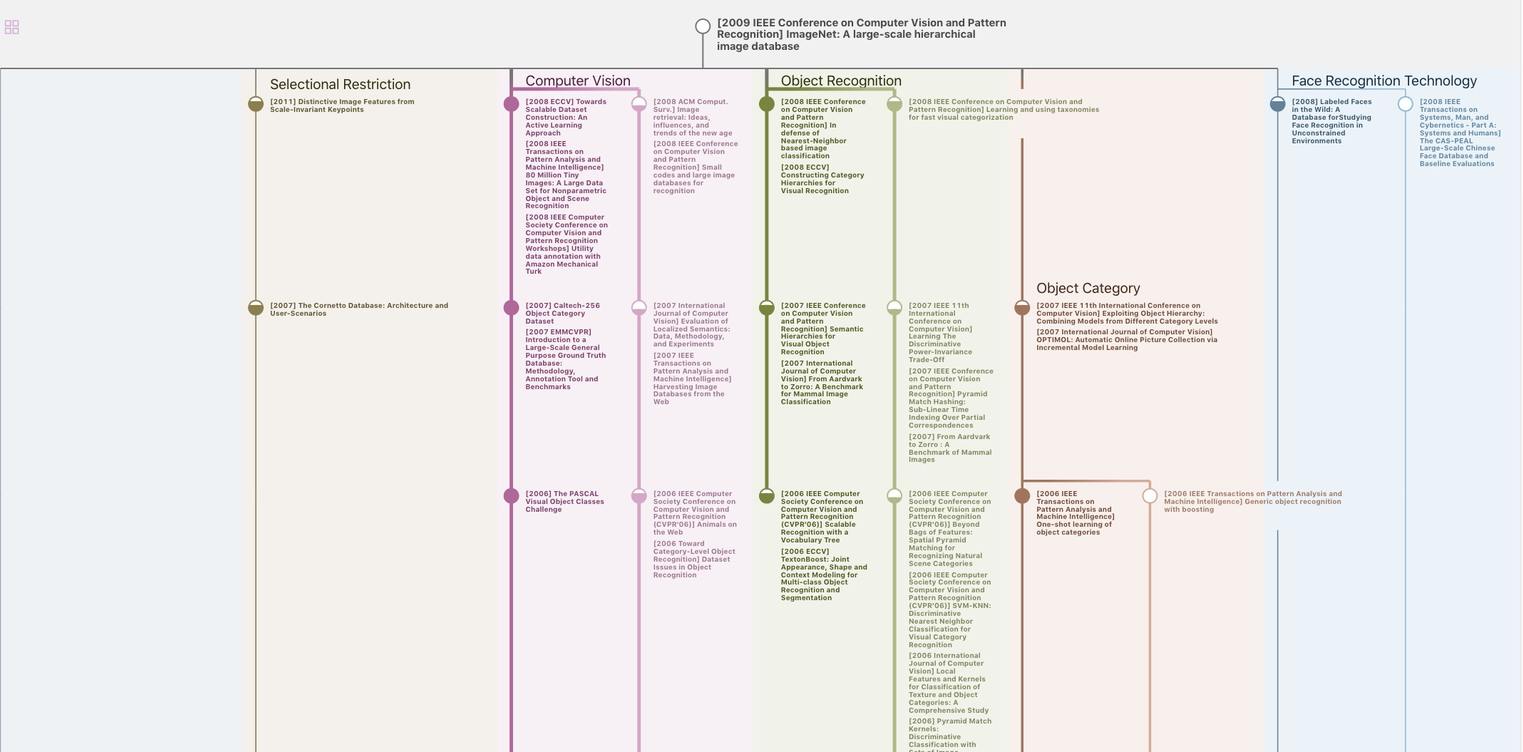
生成溯源树,研究论文发展脉络
Chat Paper
正在生成论文摘要