Multiple conformational states in retrospective virtual screening – homology models vs. crystal structures: beta-2 adrenergic receptor case study
Journal of Cheminformatics(2015)
摘要
Background Distinguishing active from inactive compounds is one of the crucial problems of molecular docking, especially in the context of virtual screening experiments. The randomization of poses and the natural flexibility of the protein make this discrimination even harder. Some of the recent approaches to post-docking analysis use an ensemble of receptor models to mimic this naturally occurring conformational diversity. However, the optimal number of receptor conformations is yet to be determined. In this study, we compare the results of a retrospective screening of beta-2 adrenergic receptor ligands performed on both the ensemble of receptor conformations extracted from ten available crystal structures and an equal number of homology models. Additional analysis was also performed for homology models with up to 20 receptor conformations considered. Results The docking results were encoded into the Structural Interaction Fingerprints and were automatically analyzed by support vector machine. The use of homology models in such virtual screening application was proved to be superior in comparison to crystal structures. Additionally, increasing the number of receptor conformational states led to enhanced effectiveness of active vs. inactive compounds discrimination. Conclusions For virtual screening purposes, the use of homology models was found to be most beneficial, even in the presence of crystallographic data regarding the conformational space of the receptor. The results also showed that increasing the number of receptors considered improves the effectiveness of identifying active compounds by machine learning methods. Graphical abstract Comparison of machine learning results obtained for various number of beta-2 AR homology models and crystal structures.
更多查看译文
关键词
Crystal structures,Homology models,Virtual screening,Structural Interaction Fingerprints,Ensemble of receptors
AI 理解论文
溯源树
样例
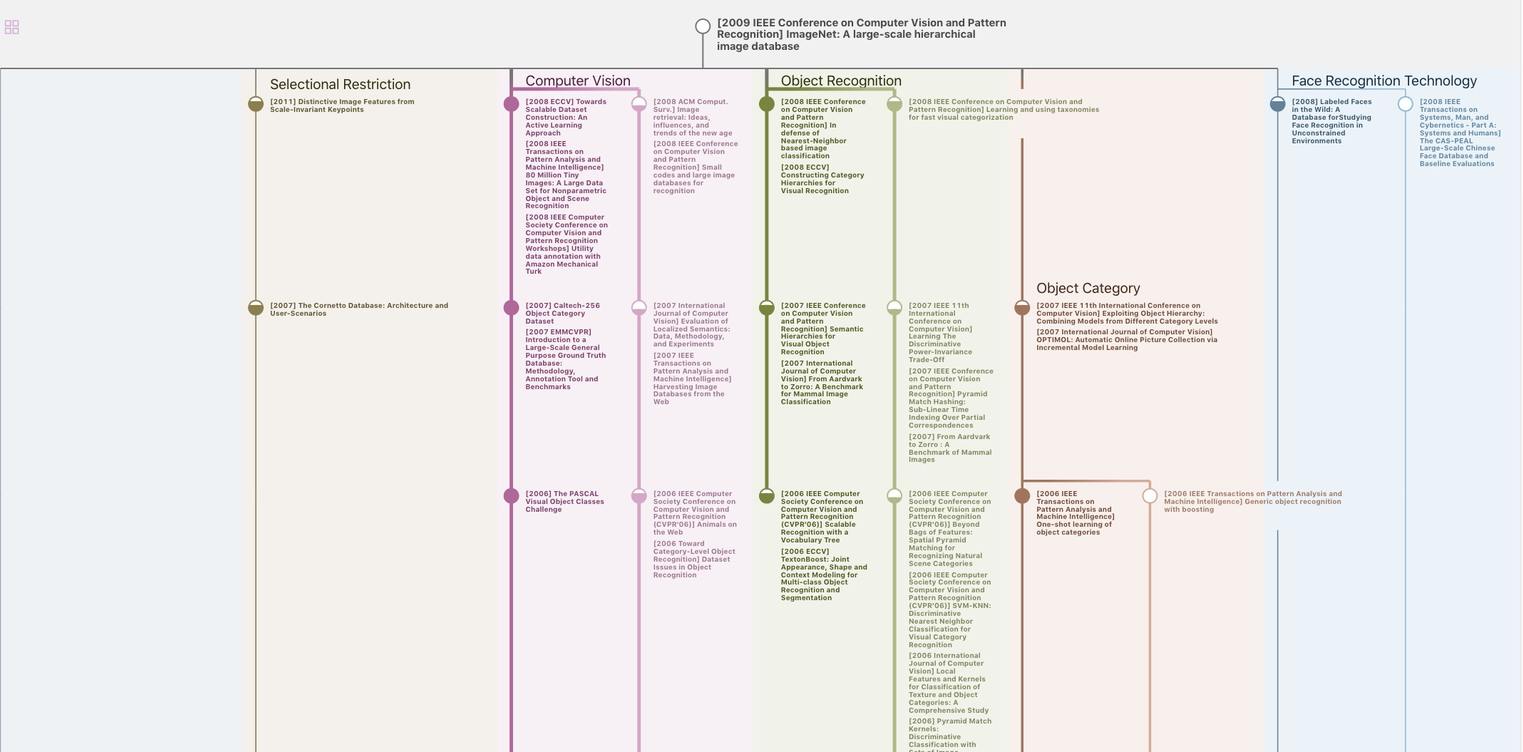
生成溯源树,研究论文发展脉络
Chat Paper
正在生成论文摘要