Facial action unit recognition using multi-class classification.
Neurocomputing(2015)
摘要
Within the context of facial expression classification using the facial action coding system (FACS), we address the problem of detecting facial action units (AUs). Feature extraction is performed by generating a large number of multi-resolution local binary pattern (MLBP) features and then selecting from these using fast correlation-based filtering (FCBF). The need for a classifier per AU is avoided by training a single error-correcting output code (ECOC) multi-class classifier to generate occurrence scores for each of several AU groups. A novel weighted decoding scheme is proposed with the weights computed using first order Walsh coefficients. Platt scaling is used to calibrate the ECOC scores to probabilities and appropriate sums are taken to obtain separate probability estimates for each AU individually. The bias and variance properties of the classifier are measured and we show that both these sources of error can be reduced by enhancing ECOC through bootstrapping and weighted decoding.
更多查看译文
关键词
Facial expression recognition,Local binary patterns,Feature selection,Error-correcting output codes
AI 理解论文
溯源树
样例
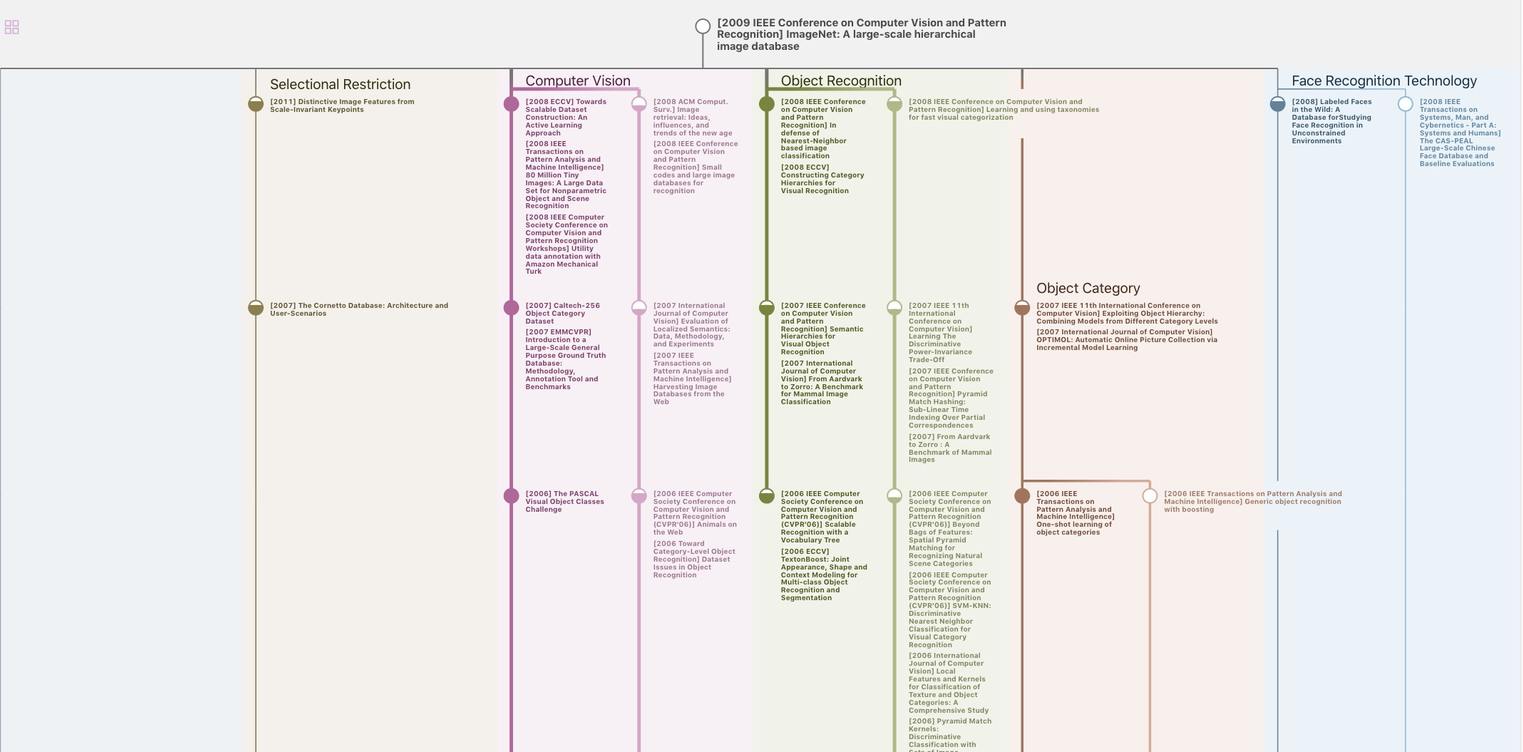
生成溯源树,研究论文发展脉络
Chat Paper
正在生成论文摘要