A transductive graphical model for single image super-resolution.
Neurocomputing(2015)
摘要
The image super-resolution technique plays a critical role in many applications, such as digital entertainments and medical diagnosis. Recently, the super-resolution method has been focused on the neighbor embedding techniques. However, these neighbor embedding based methods cannot produce sparse neighbor weights. Furthermore, these methods would not reach minor reconstructing errors only based on low-resolution patch information, which will result in high computational complexity and large construction errors. This paper presents a novel super-resolution method that incorporates iterative adaptation into neighbor selection and optimizes the model with high-resolution patches. In particular, the proposed model establishes a transductive probabilistic graphical model in light of both the low-resolution and high-resolution patches. The weights of the low-resolution neighbor patches can be treated as priori information of the construction weights for the target high-resolution image. The quality of the desired image is greatly improved in the proposed super-resolution method. Finally, the effectiveness of the proposed algorithm is demonstrated with a variety of experiment results.
更多查看译文
关键词
Super-resolution,Iterative neighbor selection,Probabilistic graph model,Bayesian theorem
AI 理解论文
溯源树
样例
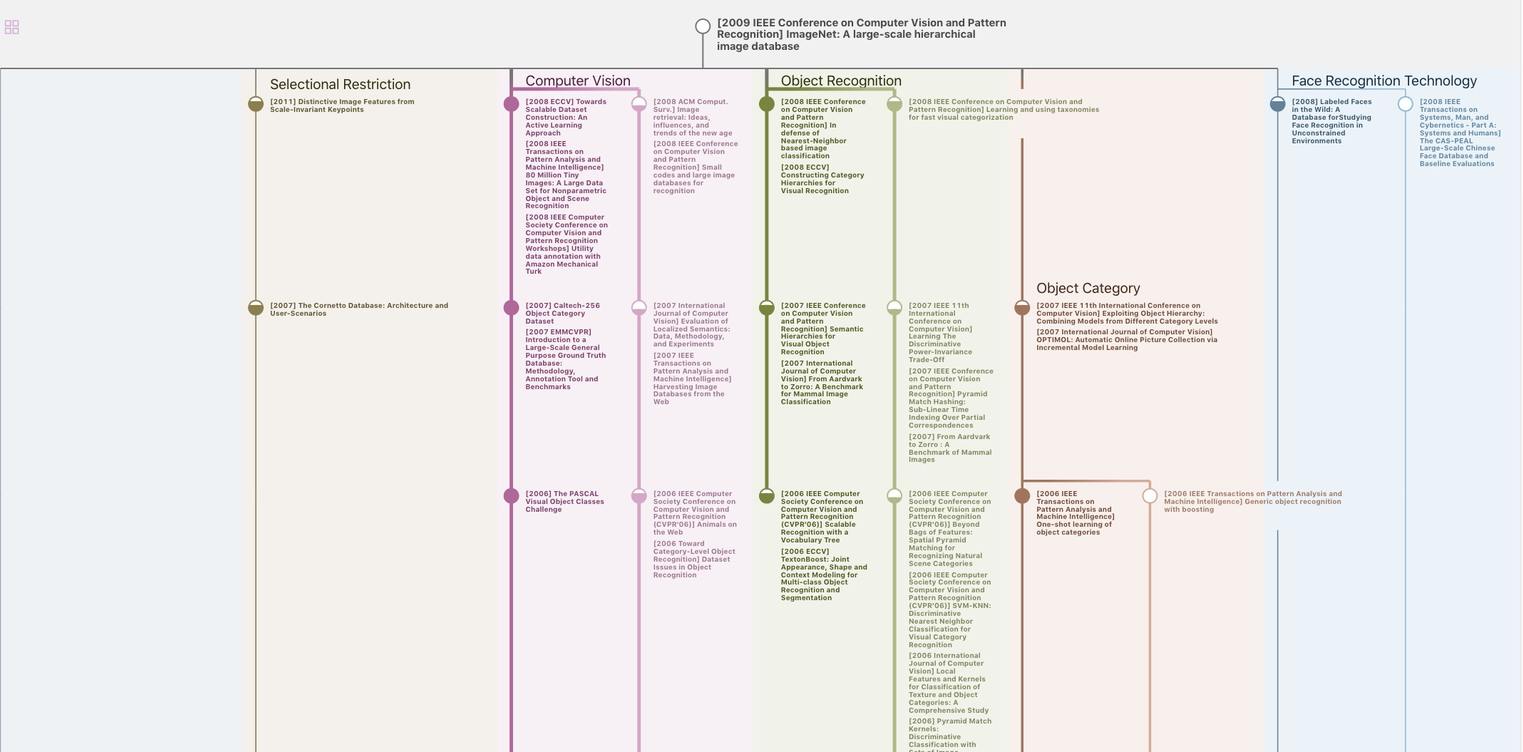
生成溯源树,研究论文发展脉络
Chat Paper
正在生成论文摘要