Fisher's linear discriminant embedded metric learning
Neurocomputing(2014)
摘要
In this paper, we propose a novel distance metric learning model, which embeds [email protected]?s linear discriminant into the classical maximum margin criterion. Specifically, given pairs of similar samples and pairs of dissimilar samples, our metric learning model aims to maximize the margin between these two kinds of sample pairs while maintaining a large mean squared distance ratio. In this way, the learned model benefits from exploiting the distributions of sample pairs and thus becomes more reliable and effective. Furthermore, the optimization problem of our model can be solved efficiently by a proposed generic iterative approximate method. The effectiveness of our model is demonstrated on various datasets including a challenging face verification dataset called Labeled Faces in the Wild.
更多查看译文
关键词
fisher's linear discriminant,machine learning,metric learning,semidefinite optimization
AI 理解论文
溯源树
样例
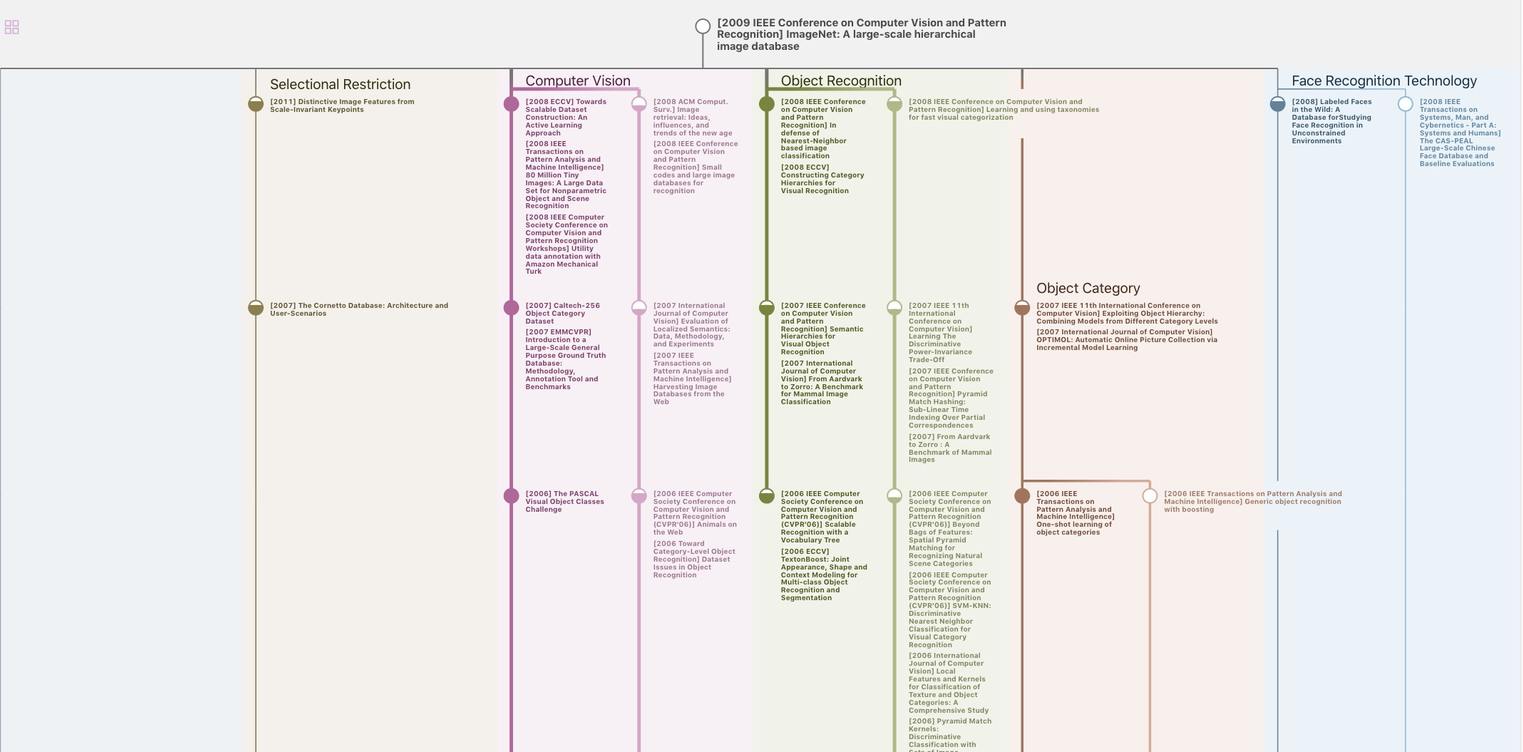
生成溯源树,研究论文发展脉络
Chat Paper
正在生成论文摘要