OMP-ELM: Orthogonal Matching Pursuit-Based Extreme Learning Machine for Regression.
JOURNAL OF INTELLIGENT SYSTEMS(2015)
摘要
Extreme learning machine (ELM) is a recent scheme for single hidden layer feed forward networks (SLFNs). It has attracted much interest in the machine intelligence and pattern recognition fields with numerous real-world applications. The ELM structure has several advantages, such as its adaptability to various problems with a rapid learning rate and low computational cost. However, it has shortcomings in the following aspects. First, it suffers from the irrelevant variables in the input data set. Second, choosing the optimal number of neurons in the hidden layer is not well defined. In case the hidden nodes are greater than the training data, the ELM may encounter the singularity problem, and its solution may become unstable. To overcome these limitations, several methods have been proposed within the regularization framework. In this article, we considered a greedy method for sparse approximation of the output weight vector of the ELM network. More specifically, the orthogonal matching pursuit (OMP) algorithm is embedded to the ELM. This new technique is named OMP-ELM. OMP-ELM has several advantages over regularized ELM methods, such as lower complexity and immunity to the singularity problem. Experimental works on nine commonly used regression problems indicate that the investigated OMP-ELM method confirms these advantages. Moreover, OMP-ELM is compared with the ELM method, the regularized ELM scheme, and artificial neural networks.
更多查看译文
关键词
ELM,regularized ELM,sparsity,OMP,SLFNs
AI 理解论文
溯源树
样例
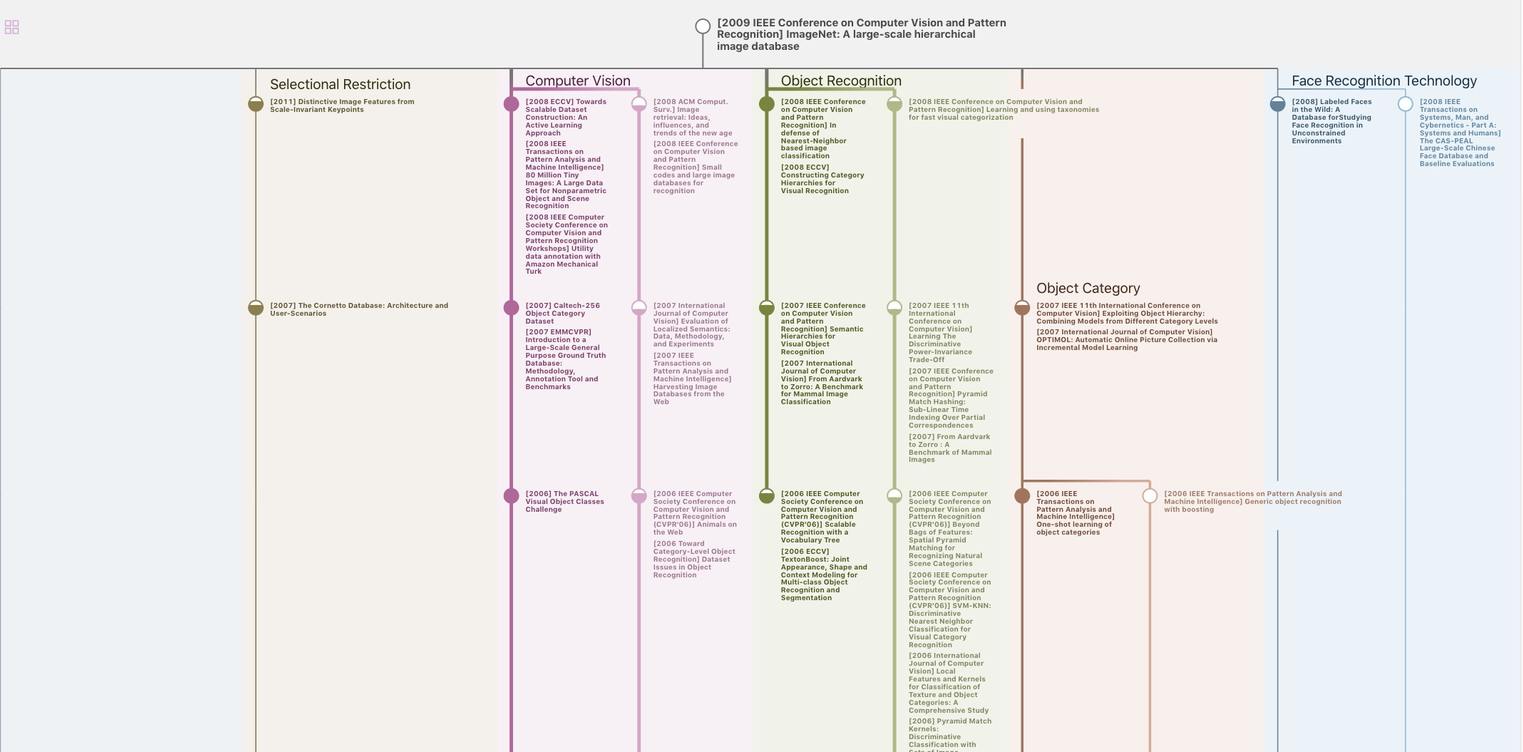
生成溯源树,研究论文发展脉络
Chat Paper
正在生成论文摘要