Detecting False Data Injection Attacks in AC State Estimation
Smart Grid, IEEE Transactions (2015)
摘要
Estimating power system states accurately is crucial to the reliable operation of power grids. Traditional weighted least square (WLS) state estimation methods face the rising threat of cyber-attacks, such as false data injection attacks, which can pass the bad data detection process in WLS state estimation. In this paper, we propose a new detection method to detect false data injection attacks by tracking the dynamics of measurement variations. The {Kullback--Leibler} distance (KLD) is used to calculate the distance between two probability distributions derived from measurement variations. When false data are injected into the power systems, the probability distributions of the measurement variations will deviate from the historical data, thus leading to a larger KLD. The proposed method is tested on IEEE 14 bus system using load data from the New York independent system operator with different attack scenarios. We have also tested our method on false data injection attacks that replace current measurement data with historical measurement data. Test results show that the proposed approach can accurately detect most of the attacks.
更多查看译文
关键词
false data injection,kullback--leibler {distance} (kld),state estimation,histograms,reactive power,indexes
AI 理解论文
溯源树
样例
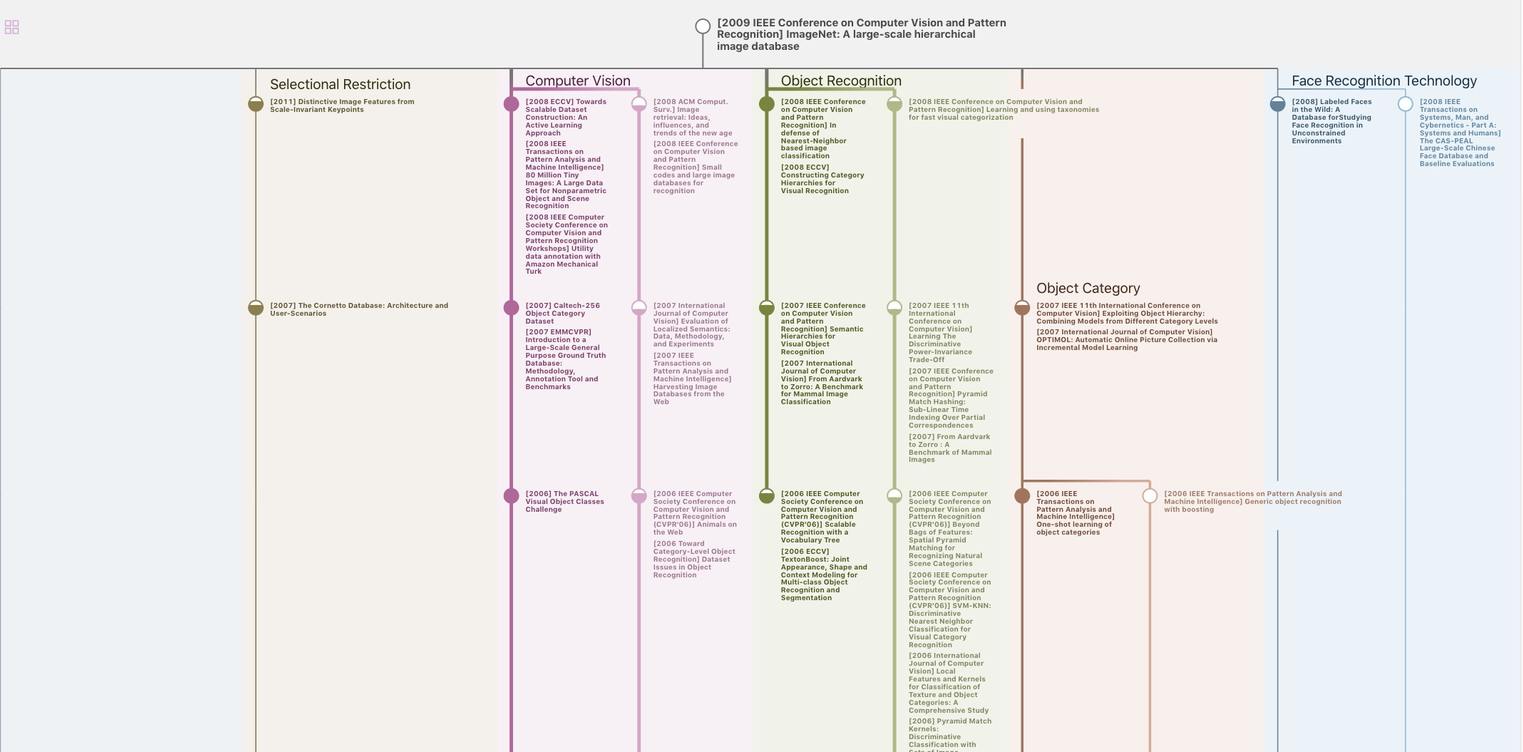
生成溯源树,研究论文发展脉络
Chat Paper
正在生成论文摘要