Sparse multivariate gaussian mixture regression.
IEEE transactions on neural networks and learning systems(2015)
摘要
Fitting a multivariate Gaussian mixture to data represents an attractive, as well as challenging problem, in especial when sparsity in the solution is demanded. Achieving this objective requires the concurrent update of all parameters (weight, centers, and precisions) of all multivariate Gaussian functions during the learning process. Such is the focus of this paper, which presents a novel method founded on the minimization of the error of the generalized logarithmic utility function (GLUF). This choice, which allows us to move smoothly from the mean square error (MSE) criterion to the one based on the logarithmic error, yields an optimization problem that resembles a locally convex problem and can be solved with a quasi-Newton method. The GLUF framework also facilitates the comparative study between both extremes, concluding that the classical MSE optimization is not the most adequate for the task. The performance of the proposed novel technique is demonstrated on simulated as well as realistic scenarios.
更多查看译文
关键词
sparsity.,logarithmic utility function,function approximation,regression,sparsity,gaussian function mixture (gfm),mixture models,kernel,minimization,quasi newton method,cost function,symmetric matrices,optimization problem,sparse matrices,regression analysis,gaussian processes,vectors
AI 理解论文
溯源树
样例
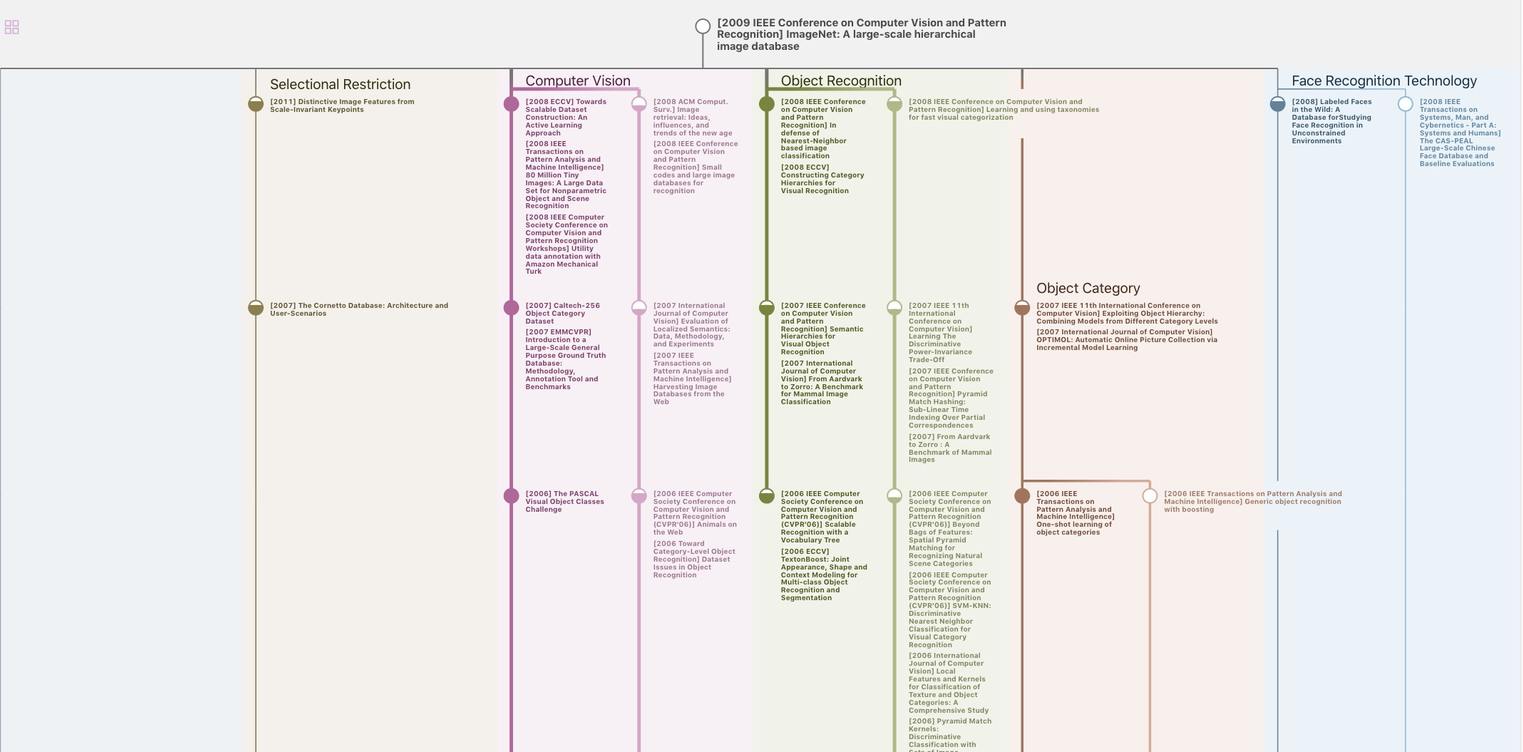
生成溯源树,研究论文发展脉络
Chat Paper
正在生成论文摘要