Enabling Health Monitoring Approach Based on Vibration Data for Accurate Prognostics
Industrial Electronics, IEEE Transactions (2015)
摘要
The performance of data-driven prognostics approaches is closely dependent on the form and trend of extracted features. Indeed, features that clearly reflect the machine degradation should lead to accurate prognostics, which is the global objective of this paper. This paper contributes a new approach for feature extraction/selection: The extraction is based on trigonometric functions and cumulative transformation, and the selection is performed by evaluating feature fitness using monotonicity and trendability characteristics. The proposition is applied to the time-frequency analysis of nonstationary signals using a discrete wavelet transform. The main idea is to map raw vibration data into monotonic features with early trends, which can be easily predicted. To show that, selected features are used to build a model with a data-driven approach, namely, the summation wavelet-extreme learning machine, that enables good balance between model accuracy and complexity. For validation and generalization purposes, the vibration data from two real applications of prognostics and health management challenges are used: (1) cutting tools from a computer numerical control machine (2010); and (2) bearings from the platform PRONOSTIA (2012). The performance of the proposed approach is thoroughly compared with the classical approach by performing feature fitness analysis, cutting-tool wear “estimation”, and bearings' “long-term prediction” tasks, which validates the proposition.
更多查看译文
关键词
condition monitoring,cutting tools,discrete wavelet transforms,feature extraction,feature selection,learning (artificial intelligence),machine bearings,mechanical engineering computing,signal processing,vibrations,wear,PRONOSTIA platform,bearing long-term prediction tasks,computer numerical control machine,cumulative transformation,cutting-tool wear estimation,data-driven prognostics approach performance,discrete wavelet transform,feature extraction,feature fitness analysis,feature fitness evaluation,feature selection,generalization purposes,global objective,health monitoring approach,machine degradation,model accuracy,model complexity,monotonic features,monotonicity characteristics,nonstationary signals,raw vibration data mapping,summation wavelet-extreme learning machine,time-frequency analysis,trendability characteristics,trigonometric functions,validation purposes,Data driven,feature extraction,monitoring,prognostics
AI 理解论文
溯源树
样例
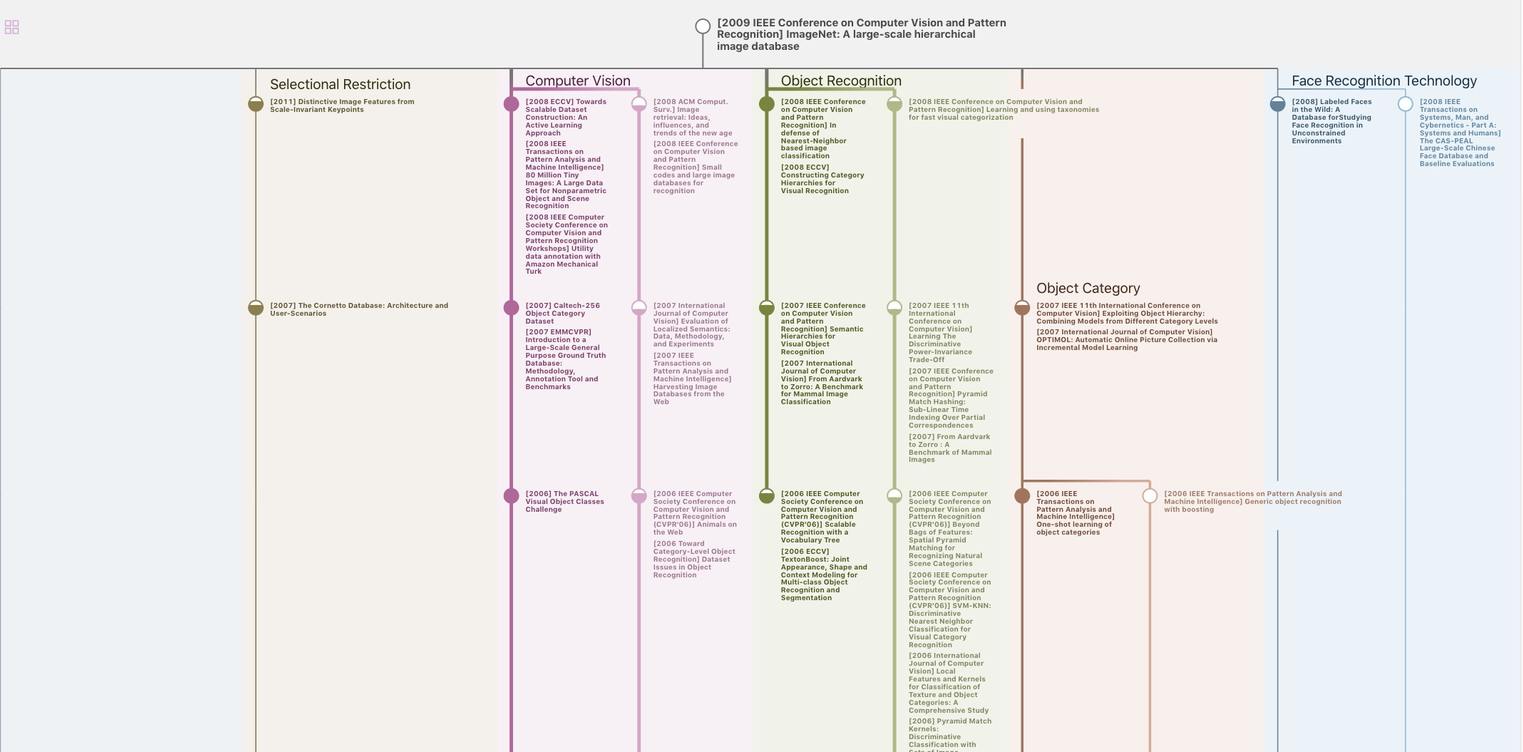
生成溯源树,研究论文发展脉络
Chat Paper
正在生成论文摘要