Graph-based sensor fusion for classification of transient acoustic signals.
IEEE transactions on cybernetics(2015)
摘要
Advances in acoustic sensing have enabled the simultaneous acquisition of multiple measurements of the same physical event via co-located acoustic sensors. We exploit the inherent correlation among such multiple measurements for acoustic signal classification, to identify the launch/impact of munition (i.e., rockets, mortars). Specifically, we propose a probabilistic graphical model framework that can explicitly learn the class conditional correlations between the cepstral features extracted from these different measurements. Additionally, we employ symbolic dynamic filtering-based features, which offer improvements over the traditional cepstral features in terms of robustness to signal distortions. Experiments on real acoustic data sets show that our proposed algorithm outperforms conventional classifiers as well as the recently proposed joint sparsity models for multisensor acoustic classification. Additionally our proposed algorithm is less sensitive to insufficiency in training samples compared to competing approaches.
更多查看译文
关键词
symbolic dynamic filtering-based features,discriminative graphs,graph-based sensor fusion,signal distortions,acoustic signal classification,acoustic signal processing,co-located acoustic sensors,symbolic features,signal classification,transient acoustic signal classification,filtering theory,graph theory,cepstral feature extraction,multiple measurements,probabilistic graphical model framework,sensor fusion,probability,feature extraction,graphical models,boosting,correlation
AI 理解论文
溯源树
样例
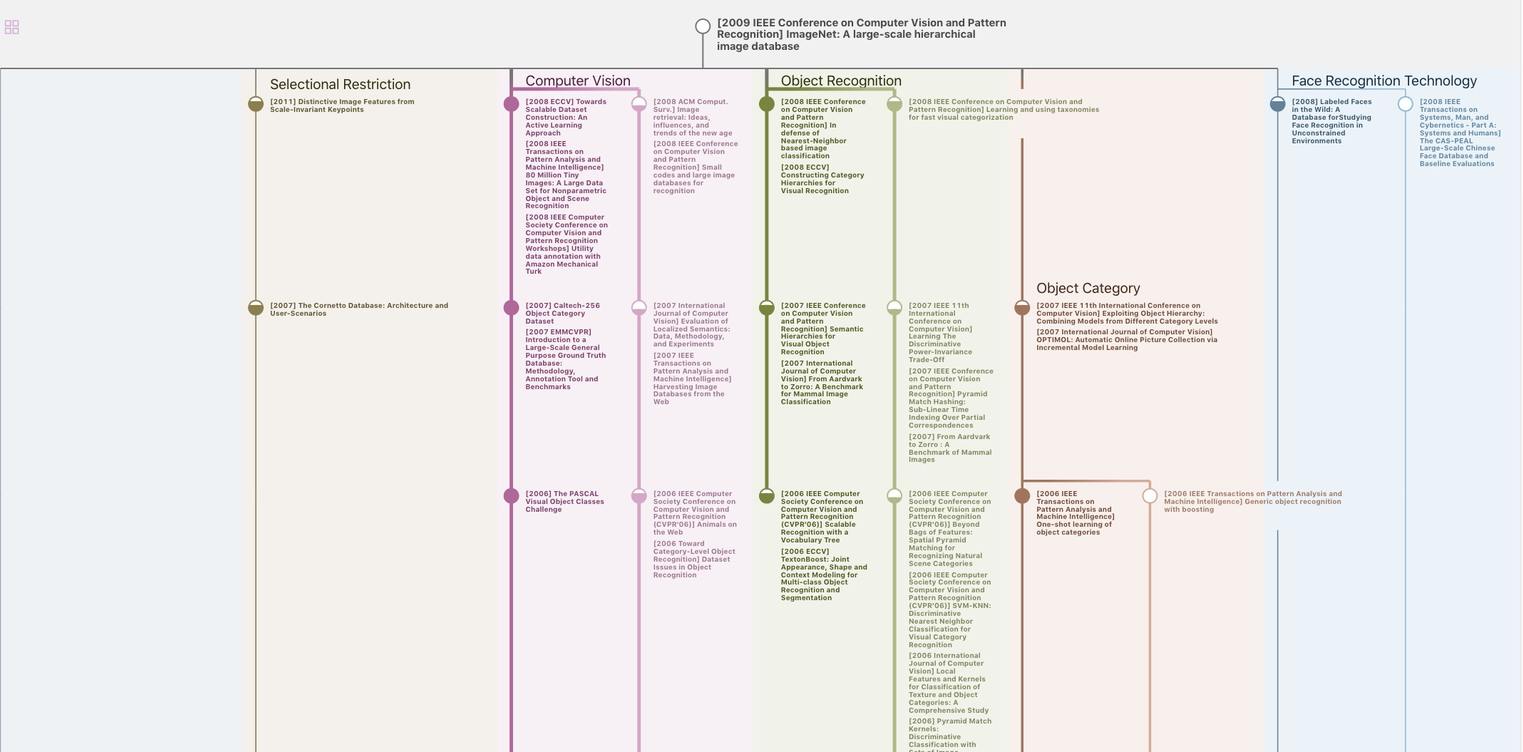
生成溯源树,研究论文发展脉络
Chat Paper
正在生成论文摘要