Multi-task Learning of Deep Neural Networks for Low-resource Speech Recognition
IEEE/ACM Transactions on Audio, Speech and Language Processing (TASLP)(2015)
摘要
We propose a multi-task learning (MTL) approach to improve low-resource automatic speech recognition using deep neural networks (DNNs) without requiring additional language resources. We first demonstrate that the performance of the phone models of a single low-resource language can be improved by training its grapheme models in parallel under the MTL framework. If multiple low-resource languages are trained together, we investigate learning a set of universal phones (UPS) as an additional task again in the MTL framework to improve the performance of the phone models of all the involved languages. In both cases, the heuristic guideline is to select a task that may exploit extra information from the training data of the primary task(s). In the first method, the extra information is the phone-to-grapheme mappings, whereas in the second method, the UPS helps to implicitly map the phones of the multiple languages among each other. In a series of experiments using three low-resource South African languages in the Lwazi corpus, the proposed MTL methods obtain significant word recognition gains when compared with single-task learning (STL) of the corresponding DNNs or ROVER that combines results from several STL-trained DNNs.
更多查看译文
关键词
Multi-task learning,deep neural network,lowresource speech recognition,universal grapheme set,universal phone set
AI 理解论文
溯源树
样例
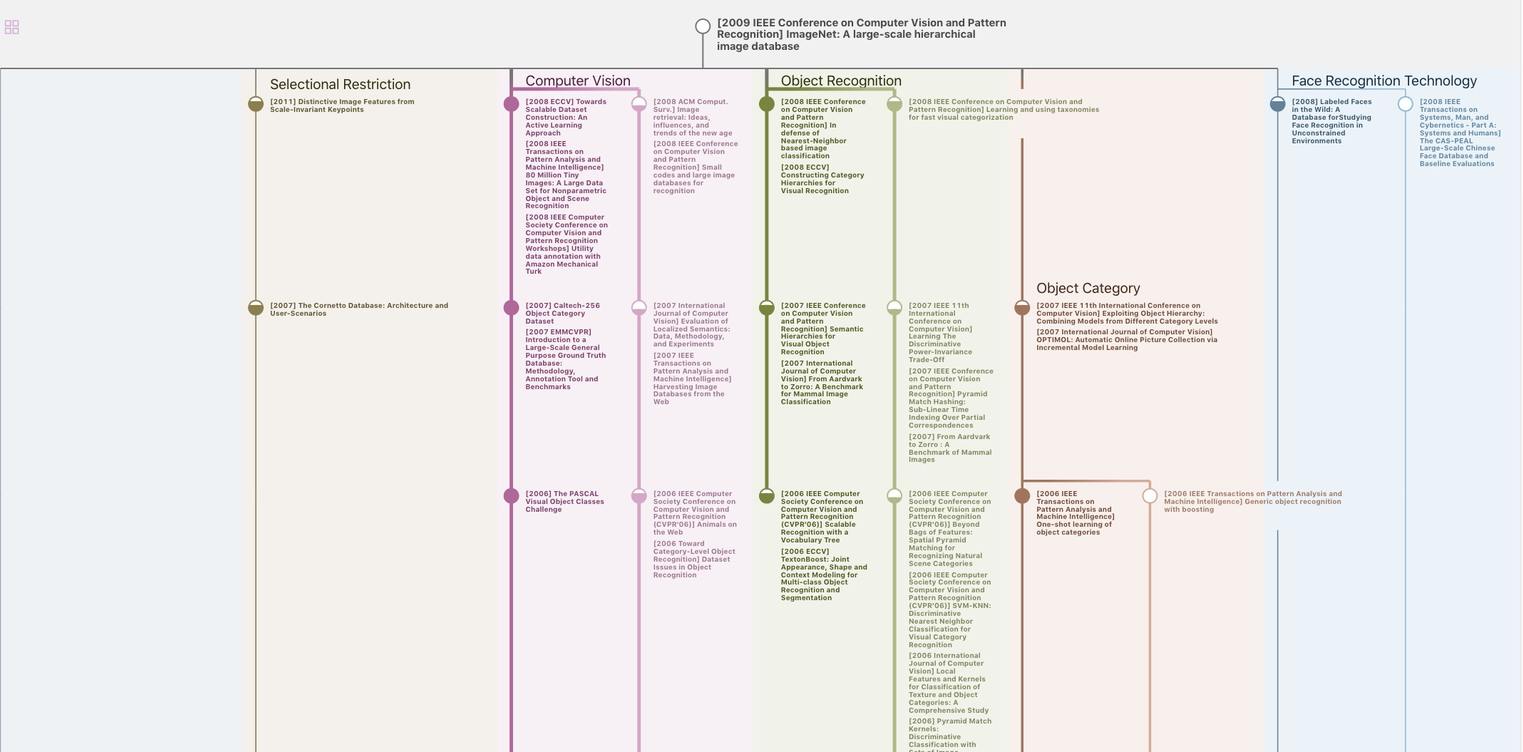
生成溯源树,研究论文发展脉络
Chat Paper
正在生成论文摘要