Towards Convenient Calibration for Cross-Ratio Based Gaze Estimation
Applications of Computer Vision(2015)
摘要
Eye gaze movements are considered as a salient modality for human computer interaction applications. Recently, cross-ratio (CR) based eye tracking methods have attracted increasing interest because they provide remote gaze estimation using a single uncalibrated camera. However, due to the simplification assumptions in CR-based methods, their performance is lower than the model-based approaches [8]. Several efforts have been made to improve the accuracy by compensating for the assumptions with subject specific calibration. This paper presents a CR-based automatic gaze estimation system that accurately works under natural head movements. A subject-specific calibration method based on regularized least-squares regression (LSR) is introduced for achieving higher accuracy compared to other state-of-the-art calibration methods. Experimental results also show that the proposed calibration method generalizes better when fewer calibration points are used. This enables user friendly applications with minimum calibration effort without sacrificing too much accuracy. In addition, we adaptively fuse the estimation of the point of regard (PoR) from both eyes based on the visibility of eye features. The adaptive fusion scheme reduces accuracy error by around 20% and also increases the estimation coverage under natural head movements.
更多查看译文
关键词
calibration,estimation theory,gaze tracking,human computer interaction,image fusion,least squares approximations,regression analysis,cr based eye tracking methods,por estimation,adaptive fusion scheme,cross-ratio based automatic gaze estimation,eye gaze movements,human computer interaction applications,point of regard estimation,regularized lsr,regularized least-square regression,subject specific calibration method,uncalibrated camera,cross ratio,accuracy,light emitting diodes,eye tracking,feature extraction,estimation
AI 理解论文
溯源树
样例
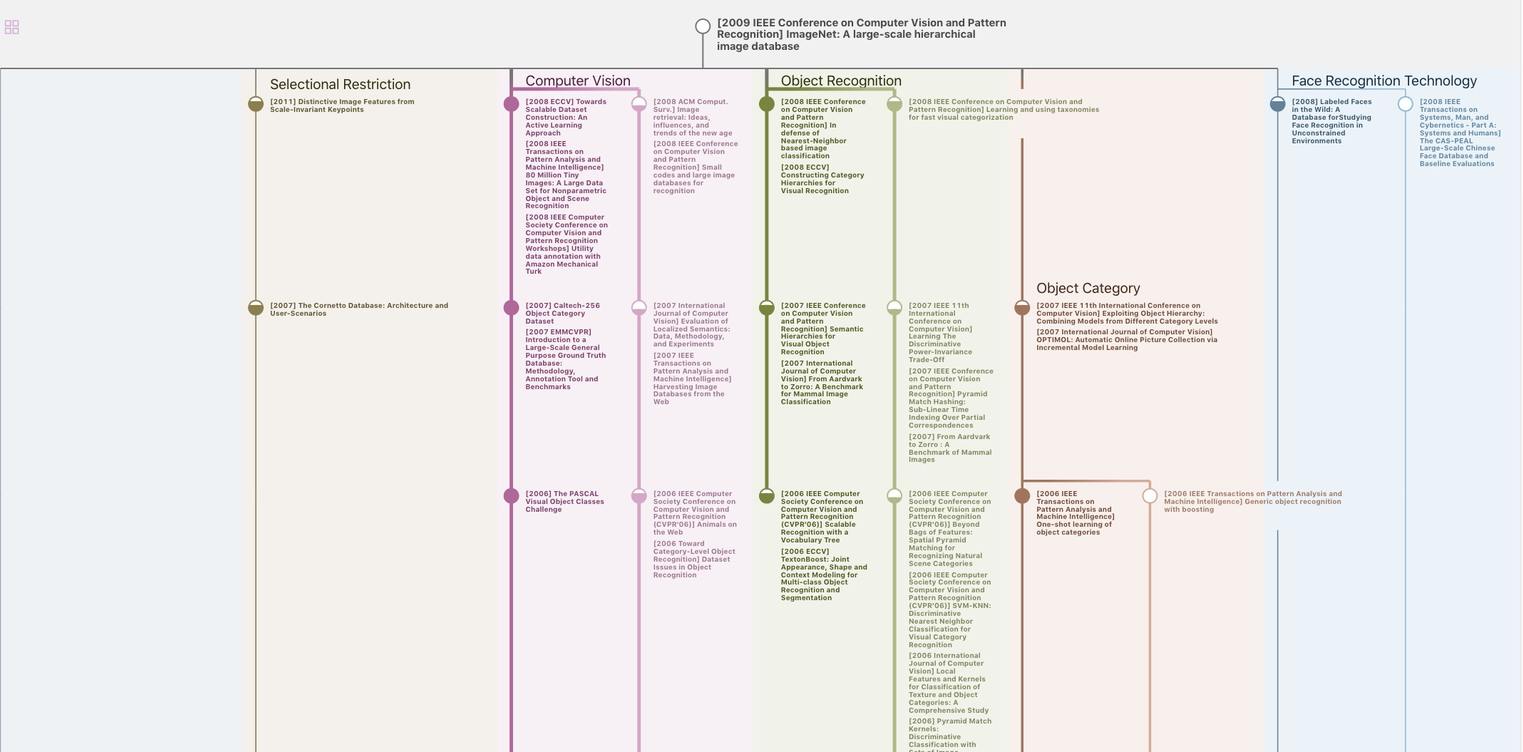
生成溯源树,研究论文发展脉络
Chat Paper
正在生成论文摘要