A Multi-modal Graphical Model for Scene Analysis
Applications of Computer Vision(2015)
Abstract
In this paper, we introduce a multi-modal graphical model to address the problems of semantic segmentation using 2D-3D data exhibiting extensive many-to-one correspondences. Existing methods often impose a hard correspondence between the 2D and 3D data, where the 2D and 3D corresponding regions are forced to receive identical labels. This results in performance degradation due to misalignments, 3D-2D projection errors and occlusions. We address this issue by defining a graph over the entire set of data that models soft correspondences between the two modalities. This graph encourages each region in a modality to leverage the information from its corresponding regions in the other modality to better estimate its class label. We evaluate our method on a publicly available dataset and beat the state-of-the-art. Additionally, to demonstrate the ability of our model to support multiple correspondences for objects in 3D and 2D domains, we introduce a new multi-modal dataset, which is composed of panoramic images and LIDAR data, and features a rich set of many-to-one correspondences.
MoreTranslated text
Key words
graph theory,image classification,image segmentation,natural scenes,2d domains,2d-3d data,2d-3d occlusions,2d-3d projection errors,3d domains,lidar data,class label estimation,identical labels,information leveraging,many-to-one correspondences,modalities,multimodal dataset,multimodal graphical model,panoramic images,performance degradation,publicly available dataset,scene analysis,semantic segmentation problems,soft correspondences,vectors,graphical models,semantics,laser radar,labeling
AI Read Science
Must-Reading Tree
Example
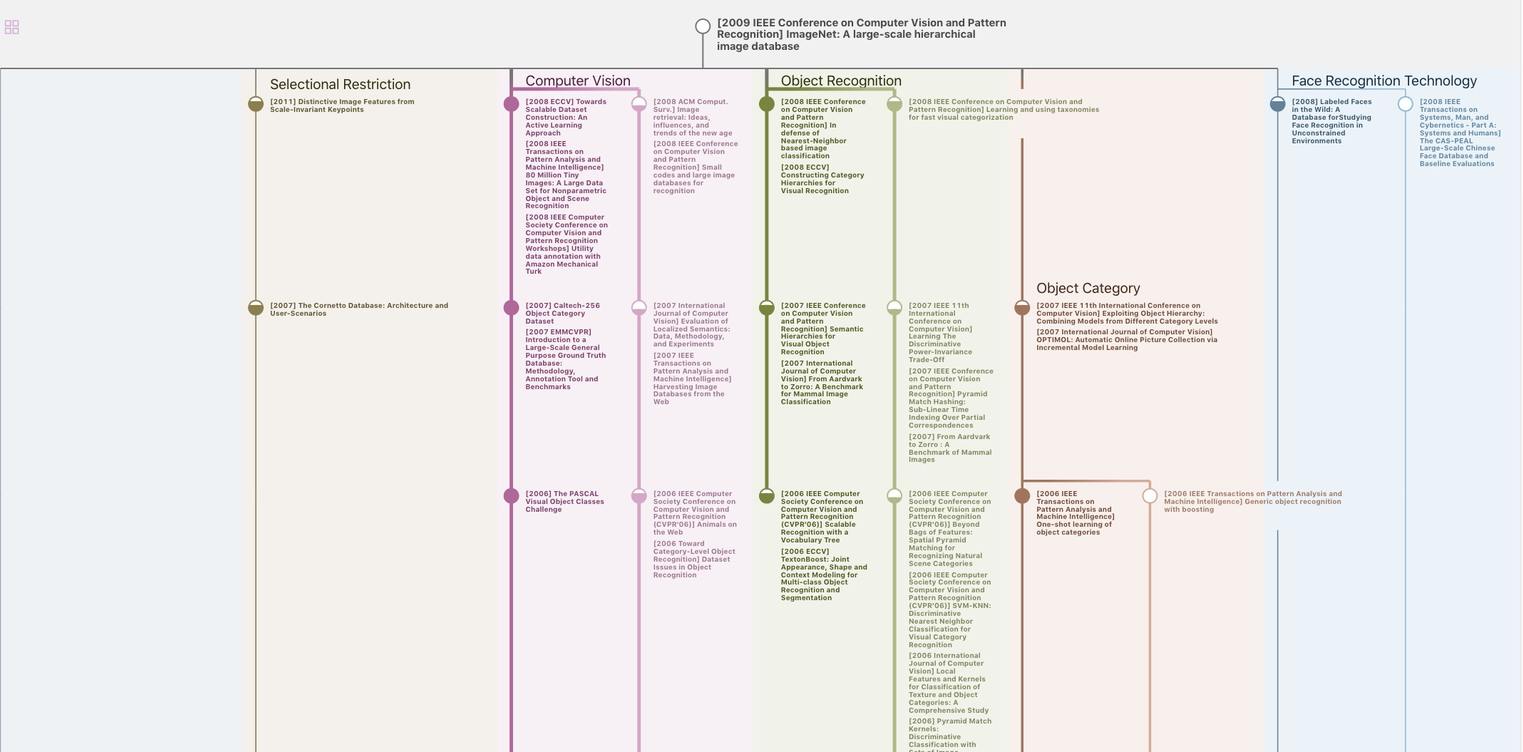
Generate MRT to find the research sequence of this paper
Chat Paper
Summary is being generated by the instructions you defined