Using Correlation-Based Feature Selection for a Diverse Collection of Bioinformatics Datasets.
Bioinformatics and Bioengineering(2014)
摘要
The large number of genes found in most gene micro array datasets demands the use of feature selection techniques to alleviate this problem of high-dimensionality. However, the computational cost of filter-based subset evaluation techniques such as Correlation-Based Feature Selection (CFS) has generally limited the use of these techniques to smaller datasets, or at least smaller collections of gene micro array datasets. No previous work has applied CFS to a large and diverse range of bioinformatics datasets. To address this deficit, we employ nine different micro array datasets exhibiting a wide range of characteristics in terms of dataset balance (fraction of instances found in the minority class) and dataset difficulty of learning (overall difficulty of building effective classification models on raw, pre-feature-selection datasets). We also use five classification learners to discover how these perform in conjunction with CFS, along with five performance metrics to give a broad perspective on our results. The results find that CFS can be used to help build effective models, in particular when used with the 5-Nearest Neighbors learner on data that is Easy or Moderate (in terms of difficulty-of-learning) or Balanced (in terms of class distribution). For other types of data, the optimal learner varies, although in most cases the Logistic Regression learner works worst in conjunction with CFS.
更多查看译文
关键词
correlation,support vector machines,bioinformatics,niobium,cancer,measurement
AI 理解论文
溯源树
样例
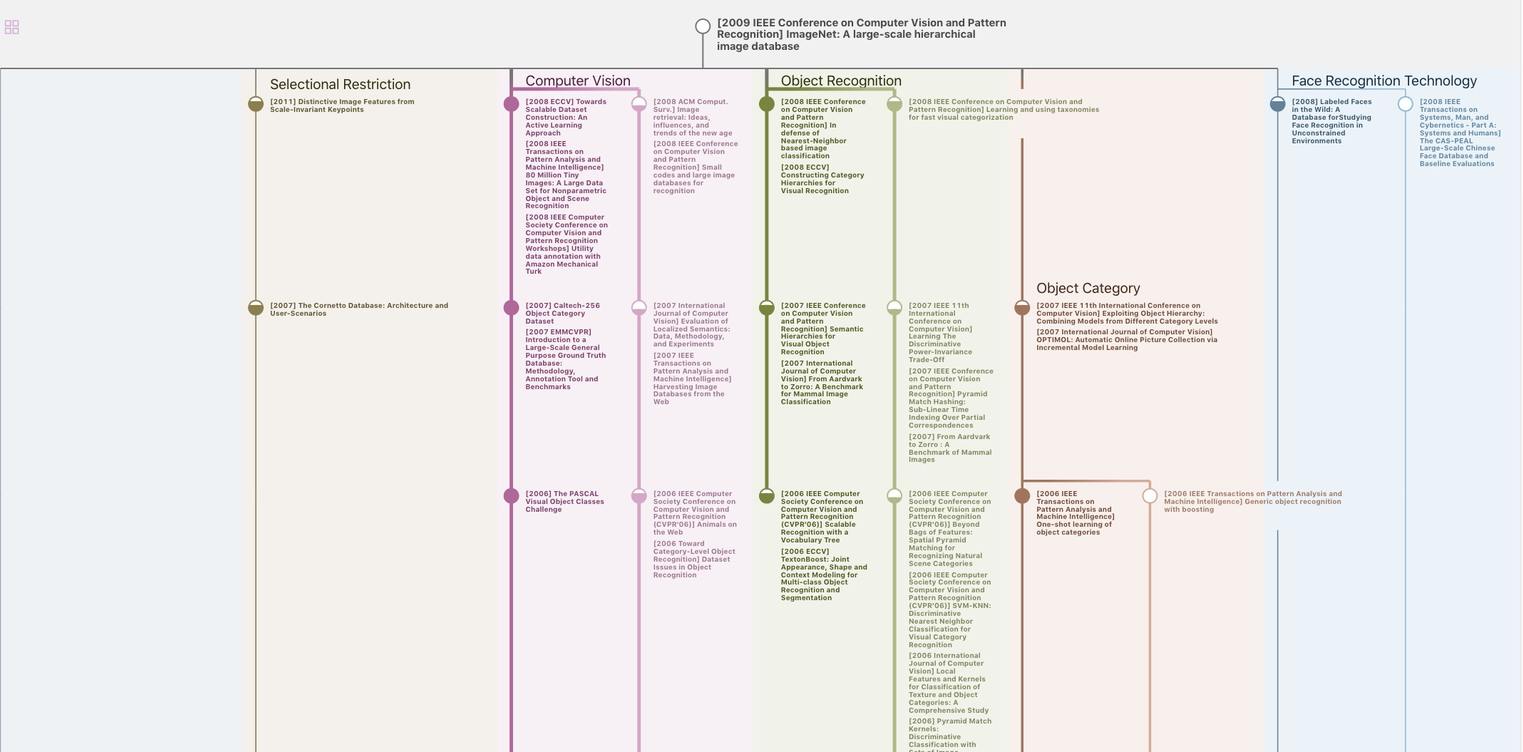
生成溯源树,研究论文发展脉络
Chat Paper
正在生成论文摘要