A 1 TOPS/W Analog Deep Machine-Learning Engine With Floating-Gate Storage in 0.13 µm CMOS
J. Solid-State Circuits(2015)
摘要
An analog implementation of a deep machine-learning system for efficient feature extraction is presented in this work. It features online unsupervised trainability and non-volatile floating-gate analog storage. It utilizes a massively parallel reconfigurable current-mode analog architecture to realize efficient computation, and leverages algorithm-level feedback to provide robustness to circuit imperfections in analog signal processing. A 3-layer, 7-node analog deep machine-learning engine was fabricated in a 0.13 μm standard CMOS process, occupying 0.36 mm 2 active area. At a processing speed of 8300 input vectors per second, it consumes 11.4 μW from the 3 V supply, achieving 1×10 12 operation per second per Watt of peak energy efficiency. Measurement demonstrates real-time cluster analysis, and feature extraction for pattern recognition with 8-fold dimension reduction with an accuracy comparable to the floating-point software simulation baseline.
更多查看译文
关键词
deep machine-learning engine,deep machine learning,power 11.4 muw,pattern clustering,current mode arithmetic,real-time cluster analysis,translinear circuits,pattern recognition,voltage 3 v,power 11.4 muW,random-access storage,Analog signal processing,standard CMOS process,cmos digital integrated circuits,algorithm-level feedback,voltage 3 V,8-fold dimension reduction,standard cmos process,analog signal processing,nonvolatile floating-gate analog storage,feature extraction,floating-point software simulation baseline,neuromorphic engineering,CMOS digital integrated circuits,massively parallel reconfigurable current-mode analog architecture,floating gate,size 0.13 mum,unsupervised learning,real-time systems,online unsupervised trainability
AI 理解论文
溯源树
样例
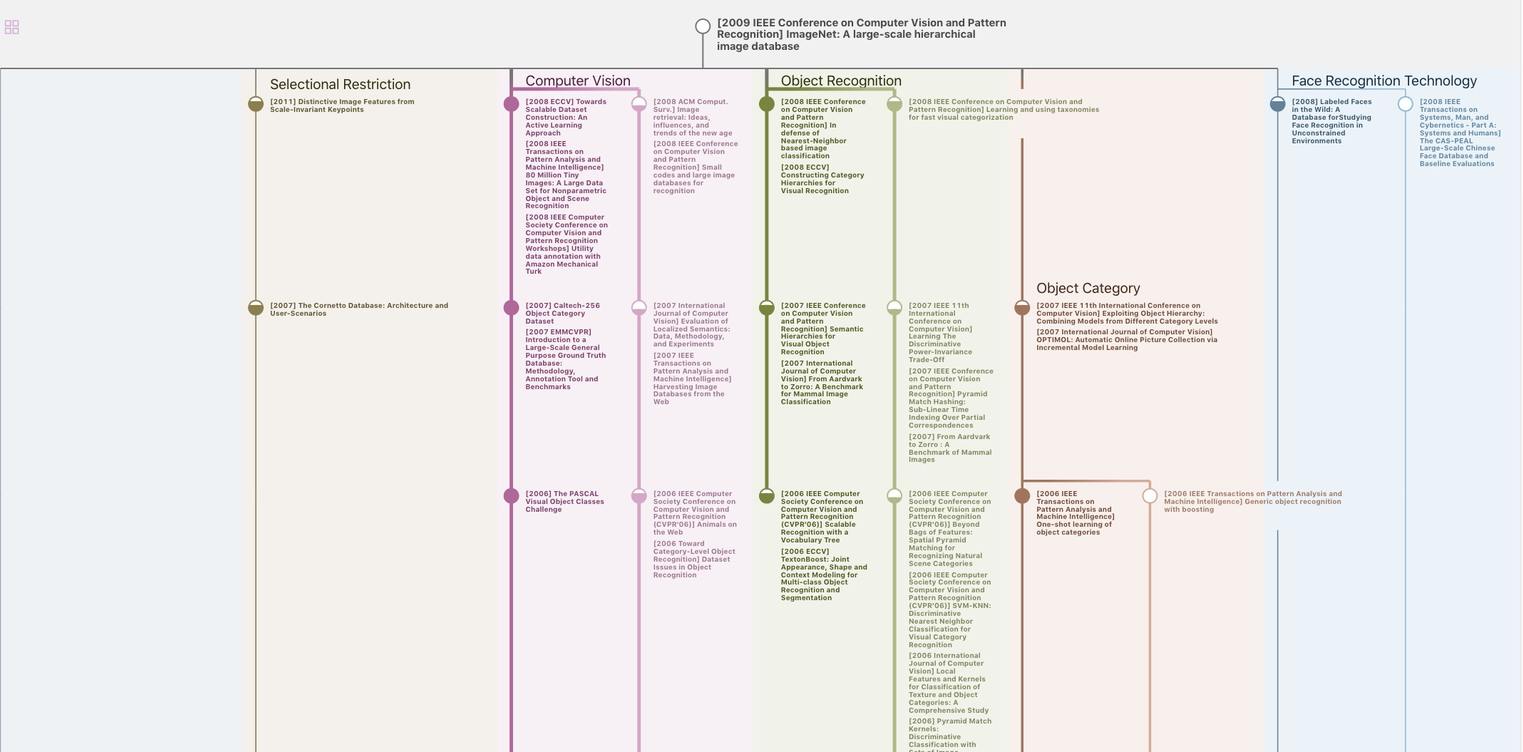
生成溯源树,研究论文发展脉络
Chat Paper
正在生成论文摘要