Simple Regret Optimization in Online Planning for Markov Decision Processes.
J. Artif. Intell. Res. (JAIR)(2014)
摘要
We consider online planning in Markov decision processes (MDPs). In online planning, the agent focuses on its current state only, deliberates about the set of possible policies from that state onwards and, when interrupted, uses the outcome of that exploratory deliberation to choose what action to perform next. Formally, the performance of algorithms for online planning is assessed in terms of simple regret, the agent's expected performance loss when the chosen action, rather than an optimal one, is followed. To date, state-of-the-art algorithms for online planning in general MDPs are either best effort, or guarantee only polynomial-rate reduction of simple regret over time. Here we introduce a new Monte-Carlo tree search algorithm, BRUE, that guarantees exponential- rate and smooth reduction of simple regret. At a high level, BRUE is based on a simple yet non-standard state-space sampling scheme, MCTS2e, in which different parts of each sample are dedicated to different exploratory objectives. We further extend BRUE with a variant of \"learning by forgetting.\" The resulting parametrized algorithm, BRUE(α), exhibits even more attractive formal guarantees than BRUE. Our empirical evaluation shows that both BRUE and its generalization, BRUE(α), are also very effective in practice and compare favorably to the state-of-the-art.
更多查看译文
AI 理解论文
溯源树
样例
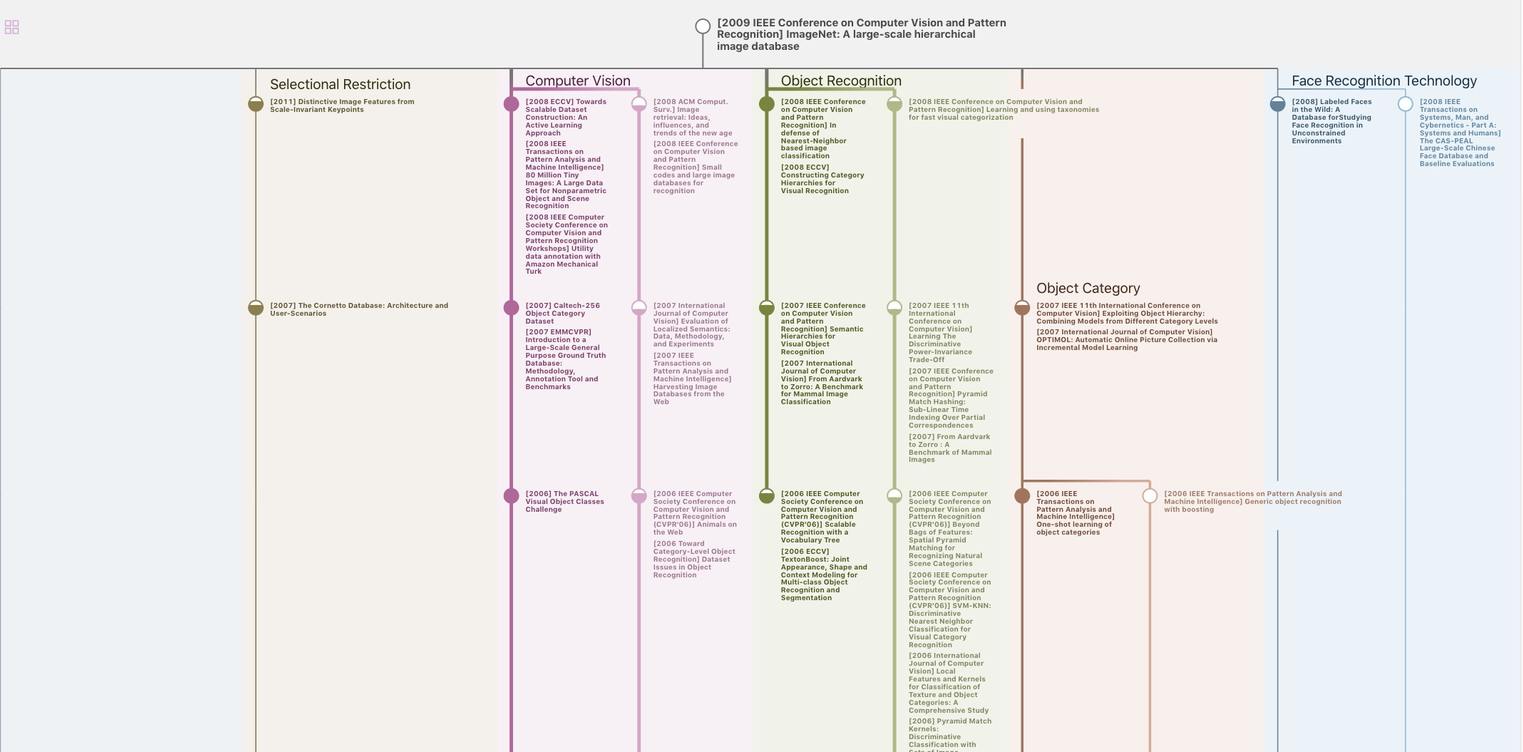
生成溯源树,研究论文发展脉络
Chat Paper
正在生成论文摘要