Support-vector-machine tree-based domain knowledge learning toward automated sports video classification
OPTICAL ENGINEERING(2010)
摘要
We propose a support-vector-machine (SVM) tree to hierarchically learn from domain knowledge represented by low-level features toward automatic classification of sports videos. The proposed SVM tree adopts a binary tree structure to exploit the nature of SVM's binary classification, where each internal node is a single SVM learning unit, and each external node represents the classified output type. Such a SVM tree presents a number of advantages, which include: 1. low computing cost; 2. integrated learning and classification while preserving individual SVM's learning strength; and 3. flexibility in both structure and learning modules, where different numbers of nodes and features can be added to address specific learning requirements, and various learning models can be added as individual nodes, such as neural networks, AdaBoost, hidden Markov models, dynamic Bayesian networks, etc. Experiments support that the proposed SVM tree achieves good performances in sports video classifications. (C) 2010 Society of Photo-Optical Instrumentation Engineers. [DOI: 10.1117/1.3518080]
更多查看译文
关键词
machine learning,sports video classifications,support vector machine
AI 理解论文
溯源树
样例
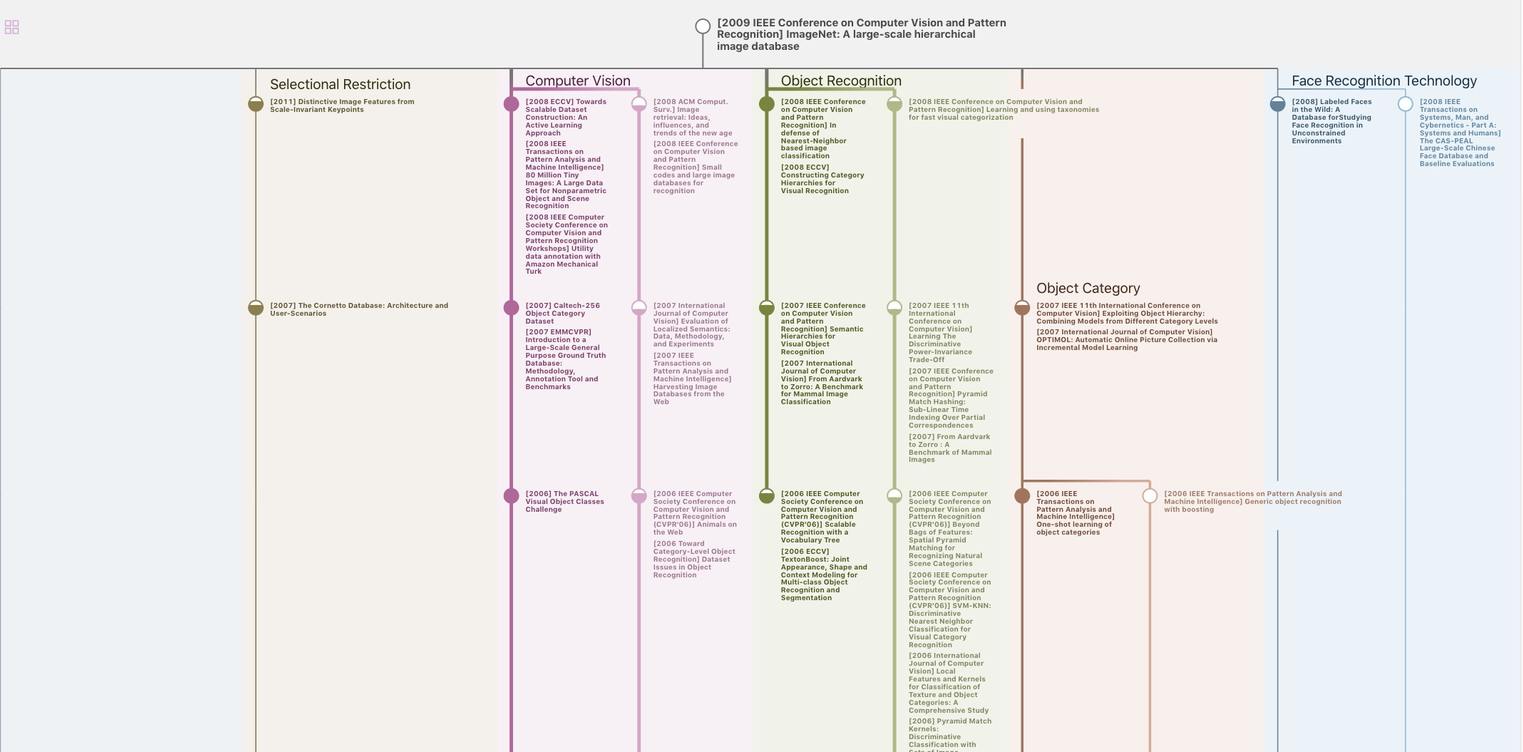
生成溯源树,研究论文发展脉络
Chat Paper
正在生成论文摘要