Probability hypothesis densities for multi-sensor, multi-target tracking with application to acoustic sensors array
Proceedings - 2nd IEEE International Conference on Advanced Computer Control, ICACC 2010(2010)
摘要
Random sets theory offers a uniform framework for the multi-source data fusion, and all problems of the data fusion could be describe, analyzed and solved in this framework. The multi-sensor multi-target tracking problem could be natural represented in the framework. It is of engineering importance to tracking low altitude moving targets with acoustic methods due to the blindness of the traditional radar detecting. In this paper, an algorithm for tracking the low altitude or ground moving targets is put forward based on the Probability Hypothesis Density (PHD) Filter. The PHD Filter based on Finite Set Statistics doesn't need consider data association for multi-target tracking, which propagates the PHD or first moment instead of the full multi-target posterior, and it could estimating the unknown and time-varying number of targets and their states under clutter environment. In the practical, we use the Sequential Monte Carlo (SMC) method to approximate the PHD. The paper presents a novel and fundamentally well-grounded framework for tracking multiple acoustic targets using PHD Filter and passive acoustic localization technique. Simulations are also presented to demonstrate the performance in tracking a randomly varying number of targets in a clutter environment. © 2010 IEEE.
更多查看译文
关键词
data fusion,monte carlo method,multi-target tracking,passive acoustic localization,phd filter,random set,monte carlo methods,radar tracking,sequential monte carlo,acoustics,clutter,set theory,probability,sensor fusion,acoustical engineering,time measurement,sensor array
AI 理解论文
溯源树
样例
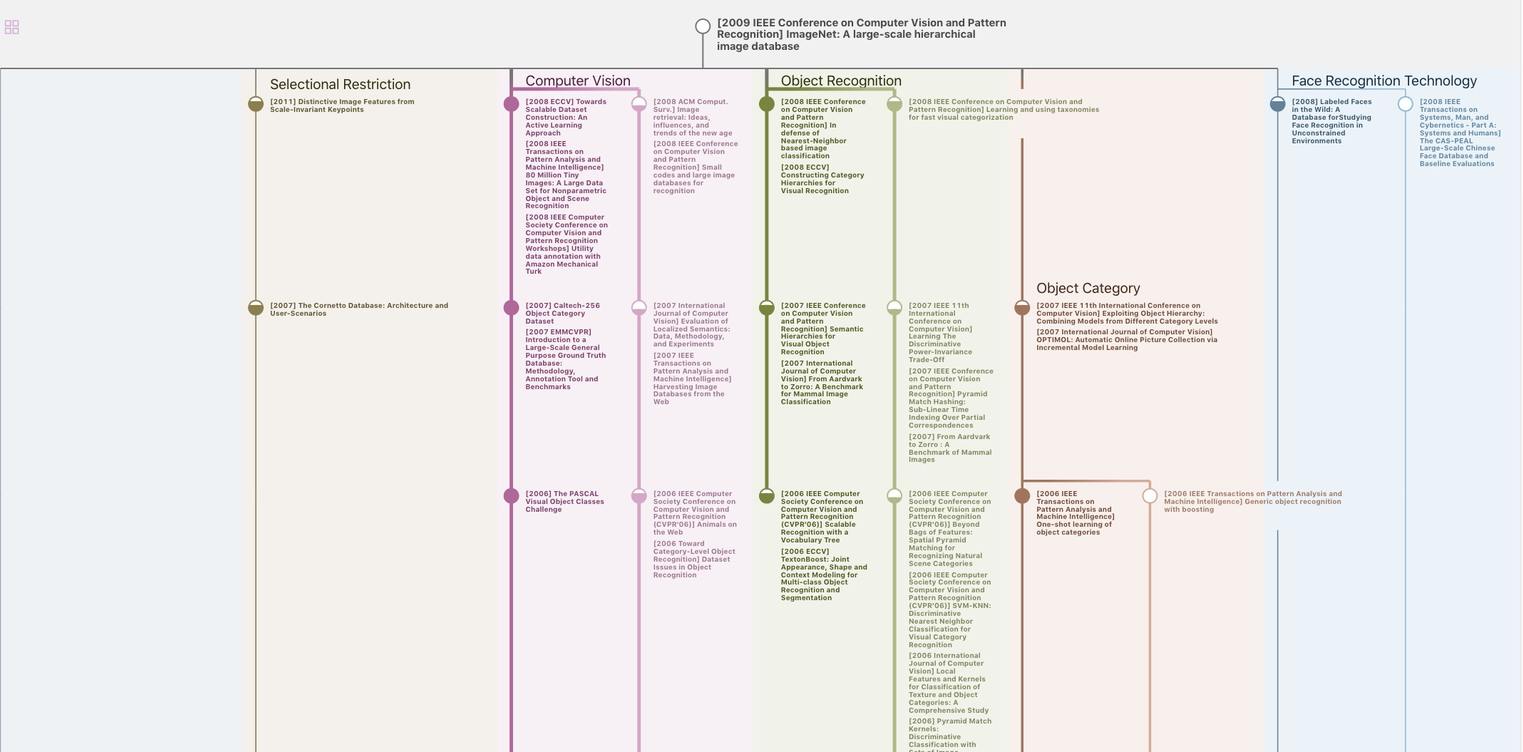
生成溯源树,研究论文发展脉络
Chat Paper
正在生成论文摘要