FTIR micro-spectroscopic imaging analysis based on PCA and LS-SVM
PIC), 2010 IEEE International Conference(2010)
摘要
FTIR micro-spectroscopic imaging analysis is a new and potential tool for subtle changes detection in biochemical composition. The excellent performance and limitation of FTIR technique are briefly discussed in this paper. In order to explore an automatic and efficacious method for chemical composition distinction, principal component analysis (PCA) and least square support vector machine (LS-SVM) is used. In this work, the unsupervised algorithm PCA is used to reduce dimension, extract linear features of original data set, and speed up the convergence in the training of LS-SVM. Then linear features are sent to LS-SVM classifier to distinguish one ingredient out of blends, in which linear inseparable ingredients can be properly adjusted by changing kernel function and parameters of LS-SVM. The experimental results indicate that this method achieves high classification accuracy and low dependence on the experience and capacity of scientific researchers.
更多查看译文
关键词
biochemical composition,ftir micro-spectoscopic imaging,chemical engineering computing,least square support vector machine (ls-svm),pattern classification,ftir,convergence,least squares approximations,feature extraction,principal analysis(pca),mass spectroscopic chemical analysis,pca,classification,infrared spectroscopy,unsupervised algorithm,least square support vector machine,principal component analysis,ls svm,microspectroscopic imaging,support vector machines,fourier transform spectroscopy,change detection,kernel function,chemical composition,image classification,irrigation,least squares support vector machine,kernel,image analysis,scientific research
AI 理解论文
溯源树
样例
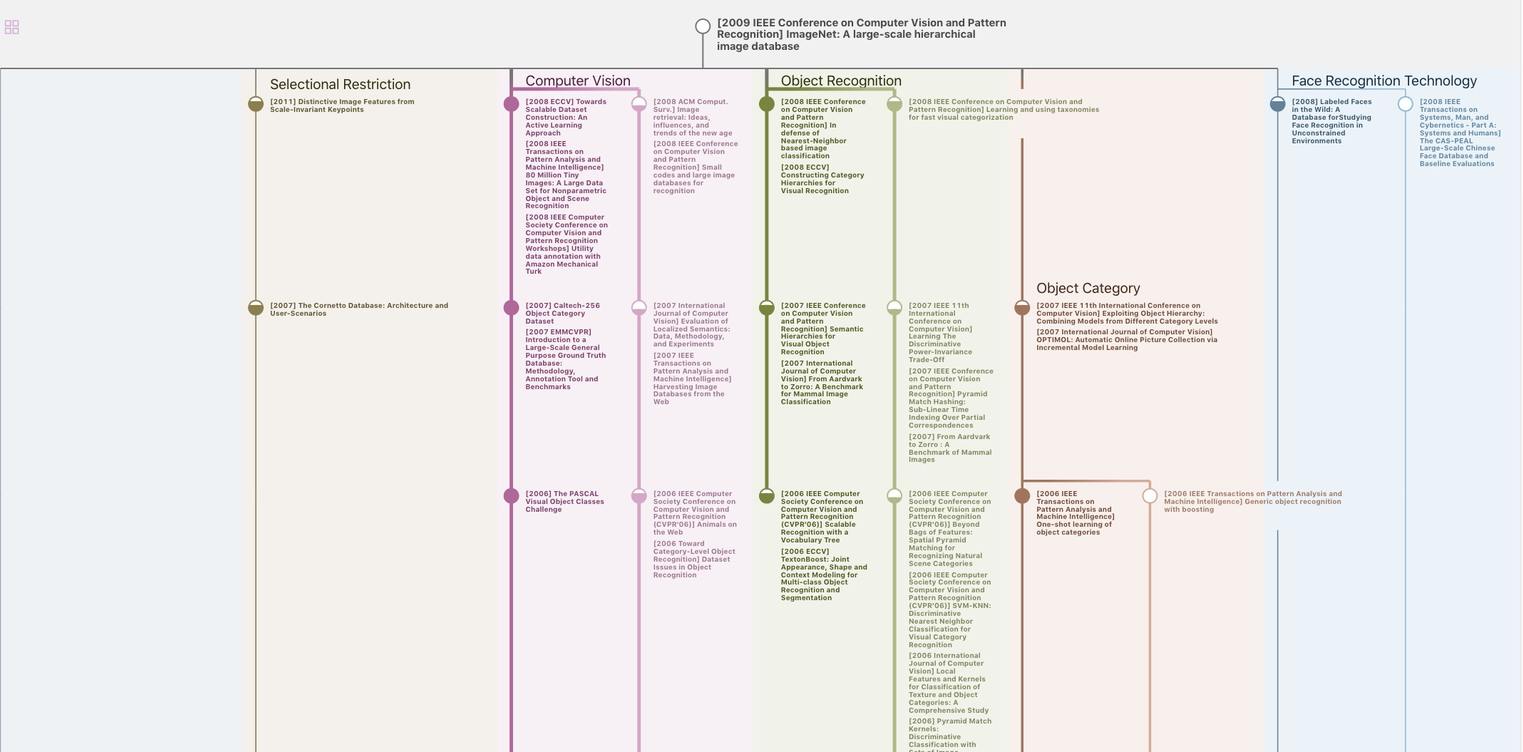
生成溯源树,研究论文发展脉络
Chat Paper
正在生成论文摘要