Construction methods for H-SVMs
Dongnan Daxue Xuebao (Ziran Kexue Ban)/Journal of Southeast University (Natural Science Edition)(2009)
摘要
By deducing the formula for the generalization power of hierarchical support vector machines (H-SVMs), it can be concluded that the generalization power of H-SVMs is related to the count, the distribution, the capacity of the input samples and H-SVMs' structure. And the guarantee of the generalization ability of the SVM node, whose position is located at a high level, can improve the performance of H-SVMs. Then, a novel method for building H-SVMs is put forward. The separation distances of SVMs are regarded as the indices for classifying and clustering. Through the top-down and bottom-up routes, the input samples are classified by maximal separation distances and clustered by minimal separation distance. The approach of classification by maximal separation distance can select the SVM whose separation margin is maximal based on the top-down route, and dichotomize the input samples according to their categories at each node. The approach of clustering by minimal separation distance can select the SVM whose separation margin is minimal based on the bottom-up route, and hierarchically cluster every two input samples according to their categories at each node. Several multiclass data sets from UCI database are selected to test this method. The experimental results show that the method is practicable, and the corresponding H-SVMs structure has a preferable and stable generalization ability.
更多查看译文
关键词
Classification by maximal separation distance,Classification tree,Clustering by minimal separation distance,Hierarchical support vector machines
AI 理解论文
溯源树
样例
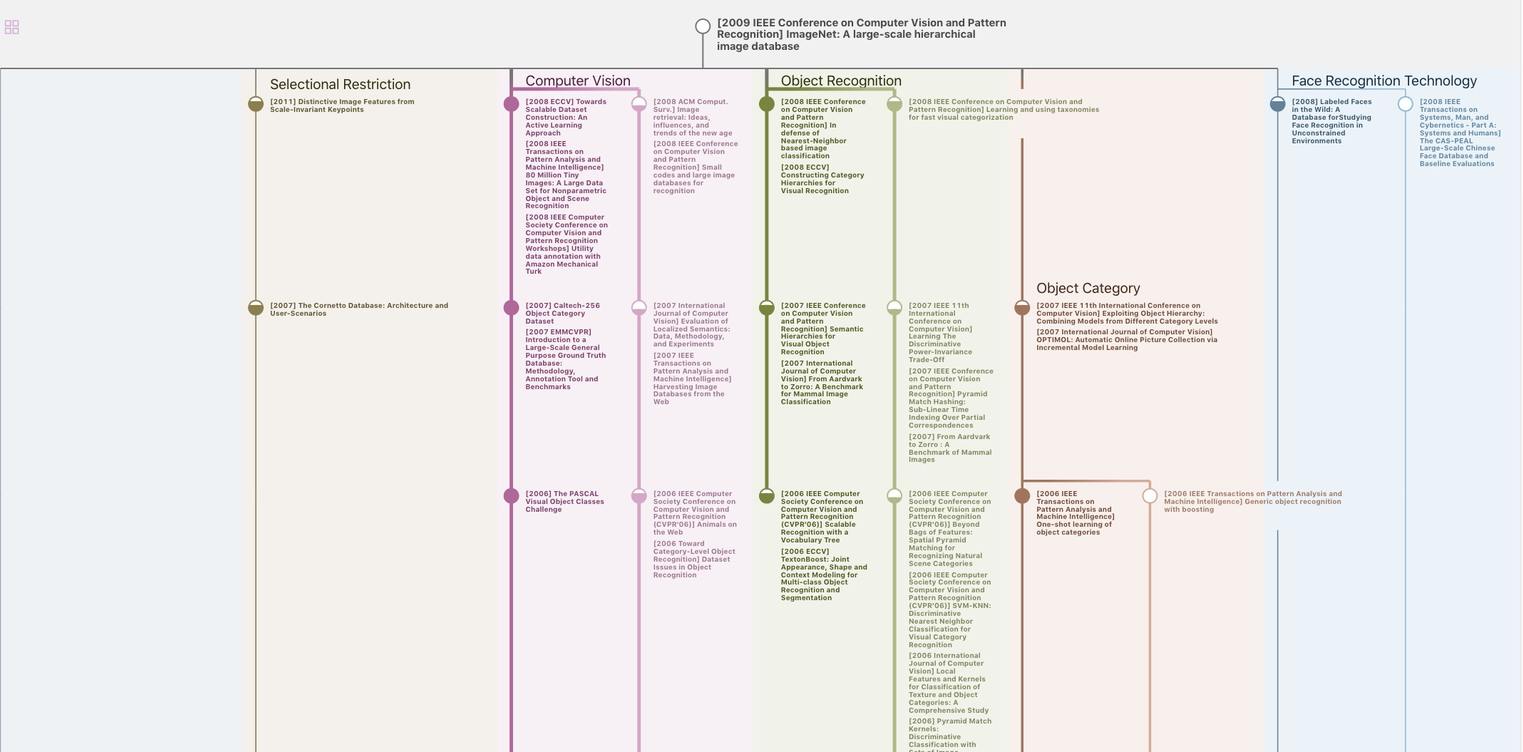
生成溯源树,研究论文发展脉络
Chat Paper
正在生成论文摘要