DAE-GAN: Underwater Image Super-Resolution Based on Degradation-Aware Attention Enhanced Generative Adversarial Network
crossref(2024)
Abstract
Underwater image often exhibit detail blurring and color distortion due to light scattering, impurities, and other influences, obscuring essential textures and details. This presents a challenge for existing super-resolution techniques in identifying and extracting effective features, making high-quality reconstruction difficult. This research aims to innovate underwater image super-resolution technology to tackle this challenge. Initially, an underwater image degradation model was created by integrating random subsampling, Gaussian blur, mixed noise, and suspended particle simulation to generate a highly realistic synthetic dataset, thereby training the network to adapt to various degradation factors. Subsequently, to enhance the network's capability to extract key features, improvements were made based on the symmetrically structured Blind Super-Resolution Generative Adversarial Network (BSRGAN) model architecture. An attention mechanism based on energy functions was introduced within the generator to assess the importance of each pixel, and a weighted fusion strategy of adversarial loss, reconstruction loss, and perceptual loss was utilized to improve the quality of image reconstruction. Experimental results demonstrate that the proposed method achieved significant improvements in Peak Signal-to-Noise Ratio (PSNR) and Underwater Image Quality Measure (UIQM) by 0.85 dB and 0.19, respectively, significantly enhancing the visual perception quality and indicating its feasibility in super-resolution applications.
MoreTranslated text
AI Read Science
Must-Reading Tree
Example
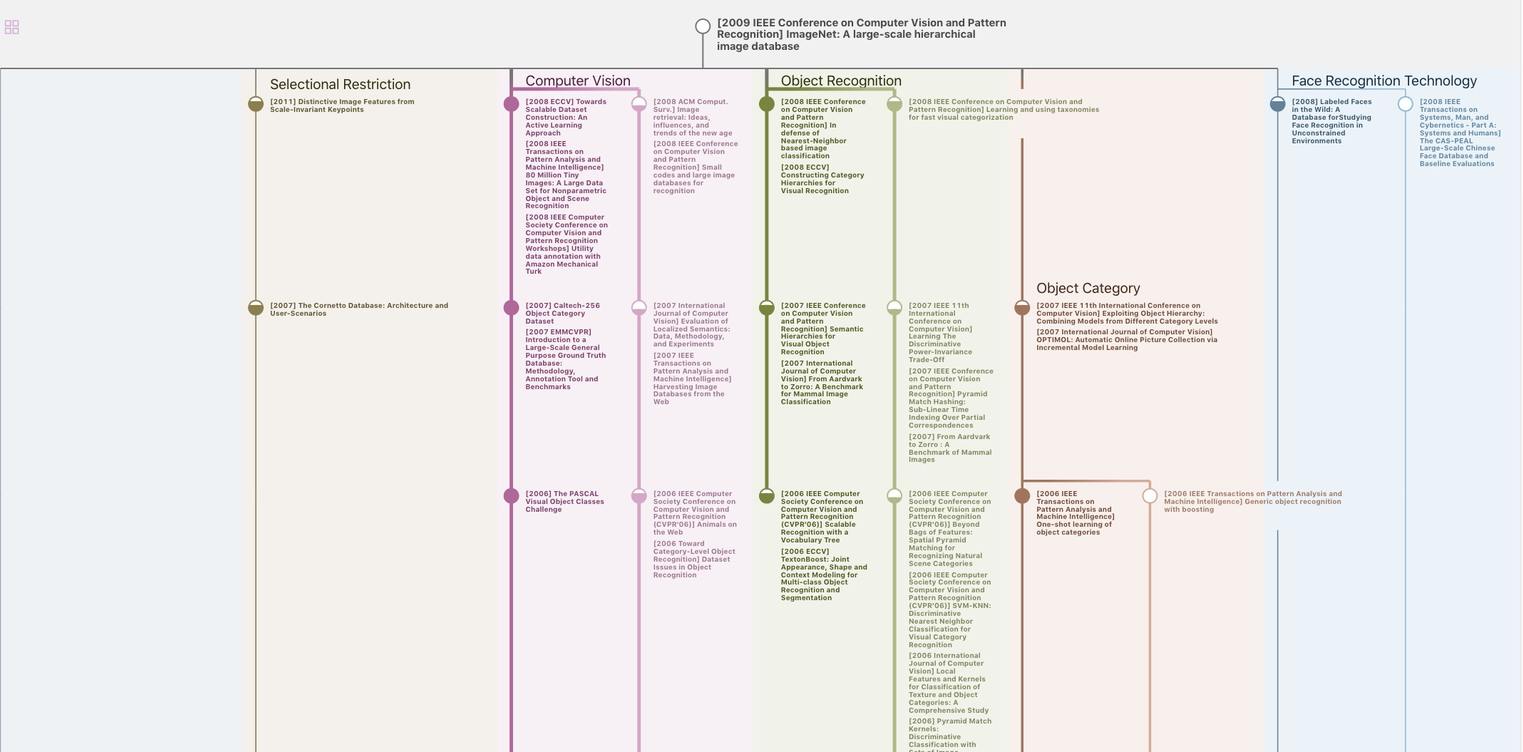
Generate MRT to find the research sequence of this paper
Chat Paper
Summary is being generated by the instructions you defined