Spectral embedding of graphs
semanticscholar(2019)
摘要
These lecture notes introduce the spectral embedding of graphs, where each node is represented by a vector of low dimension using the spectral decomposition of the Laplacian matrix. This embedding can in turn be used to apply classical learning techniques, either semi-supervised (with labels attached to some nodes) or unsupervised (like ranking or clustering nodes). We shall see that the spectral embedding of graphs is closely related to random walks in the graph. The analogy with various fields of physics, like thermodynamics, mechanics and electricity, will also emerge naturally. These notes are mainly based on [1, 2, 3, 5]. We also refer the reader to [4] for an overview on spectral clustering.
更多查看译文
AI 理解论文
溯源树
样例
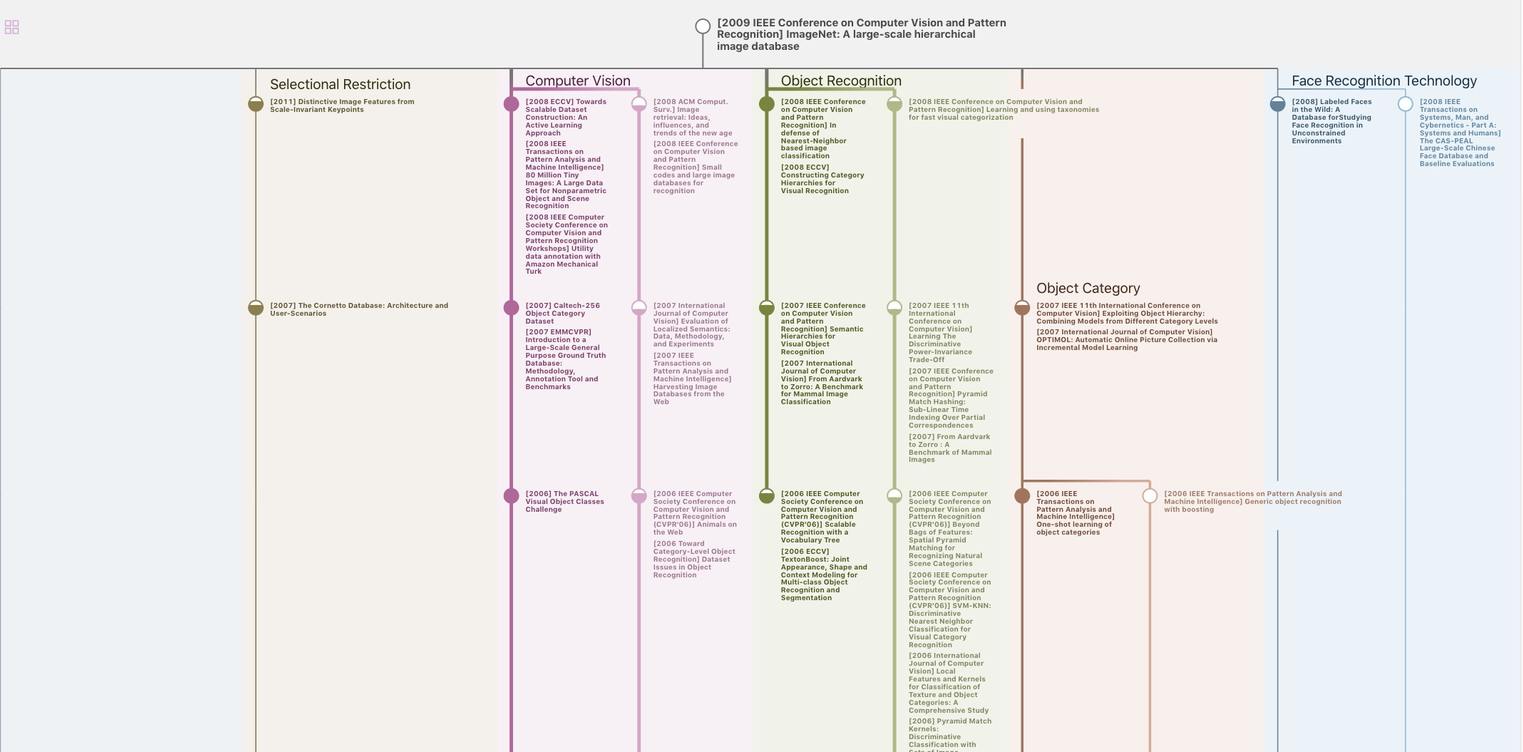
生成溯源树,研究论文发展脉络
Chat Paper
正在生成论文摘要