ε不敏感损失函数支持向量机分类性能研究
Hsi-An Chiao Tung Ta Hsueh/Journal of Xi'an Jiaotong University(2007)
Abstract
将原先用于支持向量回归的ε不敏感损失函数引入到支持向量分类中,提出ε不敏感损失函数支持向量分类算法(ε-SVC).同标准支持向量分类方法(C-SVC)和最小二乘支持向量分类方法(LS-SVC)相比较,试验结果表明:当赋予参数ε一个足够大的接近于1的值时,ε-SVC的分类正确率略低于C-SVC和LS-SVC,但是ε-SVC的训练、测试和参数选择的速度要高于C-SVC和LS-SVC.特别是对于大规模数据集,这种优势将更加明显.另外,通过精确选择参数ε的值,ε-SVC能够获得比C-SVC和LS-SVC更高的分类正确率,但是训练、测试和参数选择的速度却随着ε的减小而降低.
MoreTranslated text
Key words
ε-insensitive loss function,Pattern classification,Support vector classification,Support vector regression
AI Read Science
Must-Reading Tree
Example
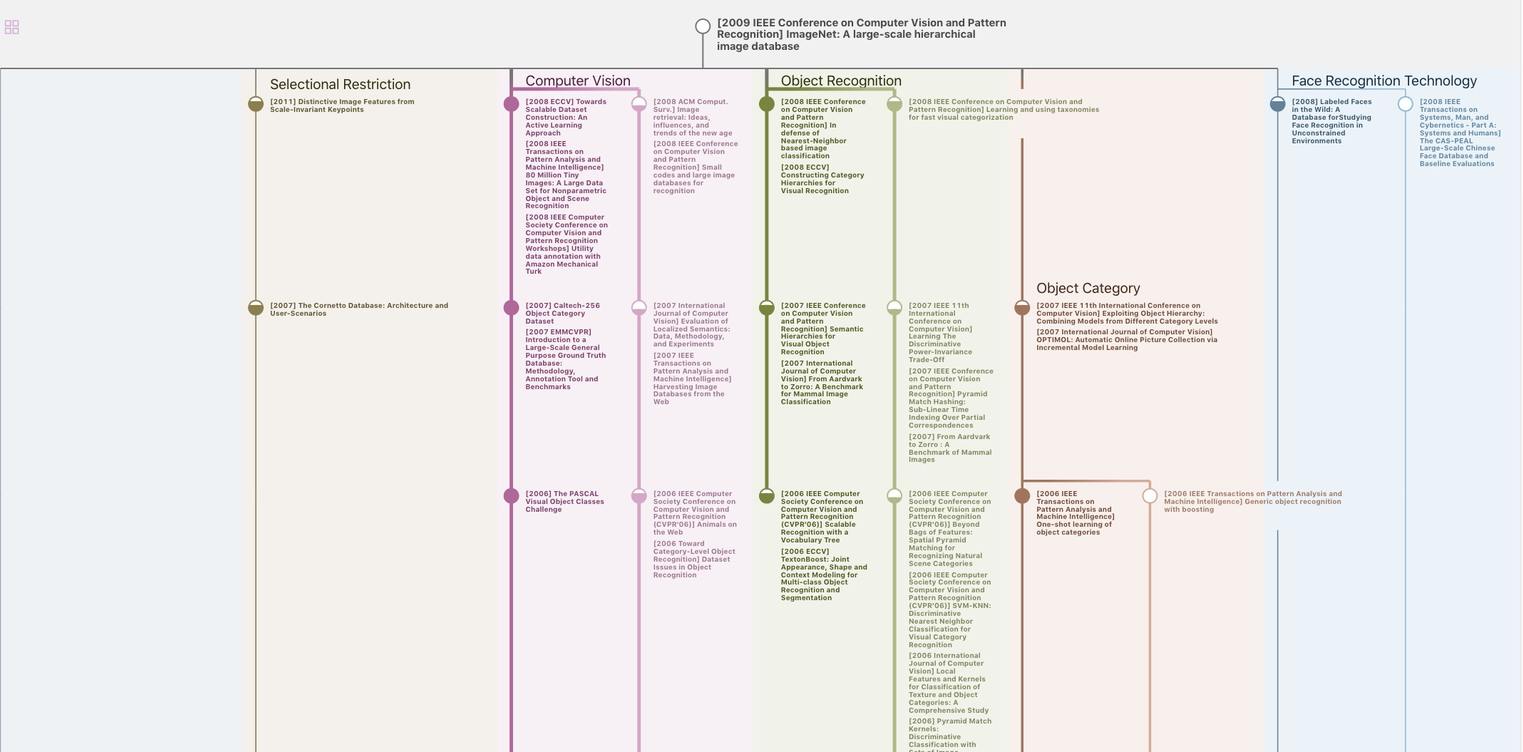
Generate MRT to find the research sequence of this paper
Chat Paper
Summary is being generated by the instructions you defined