A Curriculum-Based Meta-Learning Approach for Few-Shot Fault Diagnosis
2023 3rd International Conference on Digital Society and Intelligent Systems (DSInS)(2023)
摘要
Safety-critical systems frequently encounter few-shot fault diagnosis (FSFD) scenarios, where conventional deep learning models may not be as effective. For effective diagnosis of bearings in few-shot scenarios, we present a curriculum-based meta-learning (CBML) approach. In this approach, the task in the meta-training phase is used as a curriculum. The diagnostic knowledge in the task is learned more sufficiently through a curriculum learning strategy. Specifically, a 1DCNN-based backbone network is designed and utilizes an N-way K-shot training mode to extract the diagnostic knowledge from the meta-training tasks. A curriculum metric based on the spectral norm is proposed, which is combined with a curriculum scheduler to implement curriculum learning. The results of the experiments performed on the CWRU and PU datasets demonstrate how effective the curriculum learning strategies are in a variety of FSFD scenarios.
更多查看译文
关键词
curriculum learning,meta-learning,few-shot,fault diagnosis,bearing
AI 理解论文
溯源树
样例
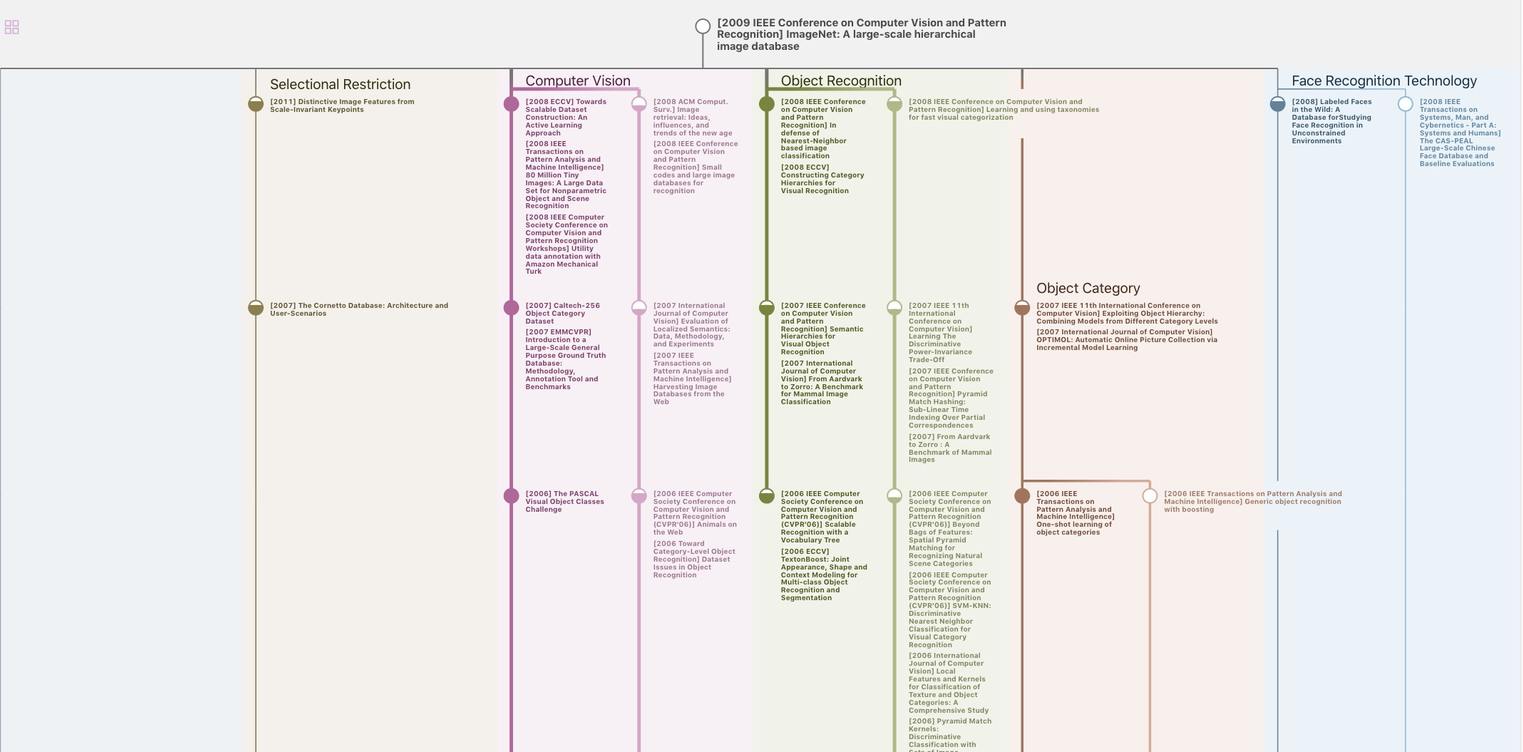
生成溯源树,研究论文发展脉络
Chat Paper
正在生成论文摘要