Feature extraction for classification of proteomic profile
Advanced Materials Research(2013)
摘要
This work studies on feature extraction for classification of proteomic profile. We evaluated four methods, including principal component analysis (PCA), independent component analysis (ICA), locally linear embedding (LLE) and weighted maximum margin criterion (WMMC). PCA, ICA and LLE extract features based on traditional low-dimension map technique. Comparatively, WMMC extracts features according to classification goal. To study classification performance of PCA, ICA, LLE and WMMC in detail, we used two well known classification methods, support vector machine (SVM) and Fisher discriminant analysis (FDA), to classify profiles. The results show WMMC having relatively good performance due to its prediction accuracy, sensitivity and specificity for diagnosis; it can correctly identify features with high discrimination ability from high-dimensional proteomic profile. When feature set size was reduced less than 10, PCA, ICA and LLE lose a lot of classification information, and the prediction accuracies are less than 90%. However, WMMC can extract most classification information. Its prediction accuracies, sensitivities and specificities are more than 95%. Obviously, WMMC is more suitable to proteomic profile classification. For classifier, FDA is sensible to feature extraction. © (2013) Trans Tech Publications, Switzerland.
更多查看译文
关键词
feature extraction,independent component analysis (ica),locally linear embedding (lle),principal component analysis (pca),proteomic profile,weighted maximum margin criterion (wmmc)
AI 理解论文
溯源树
样例
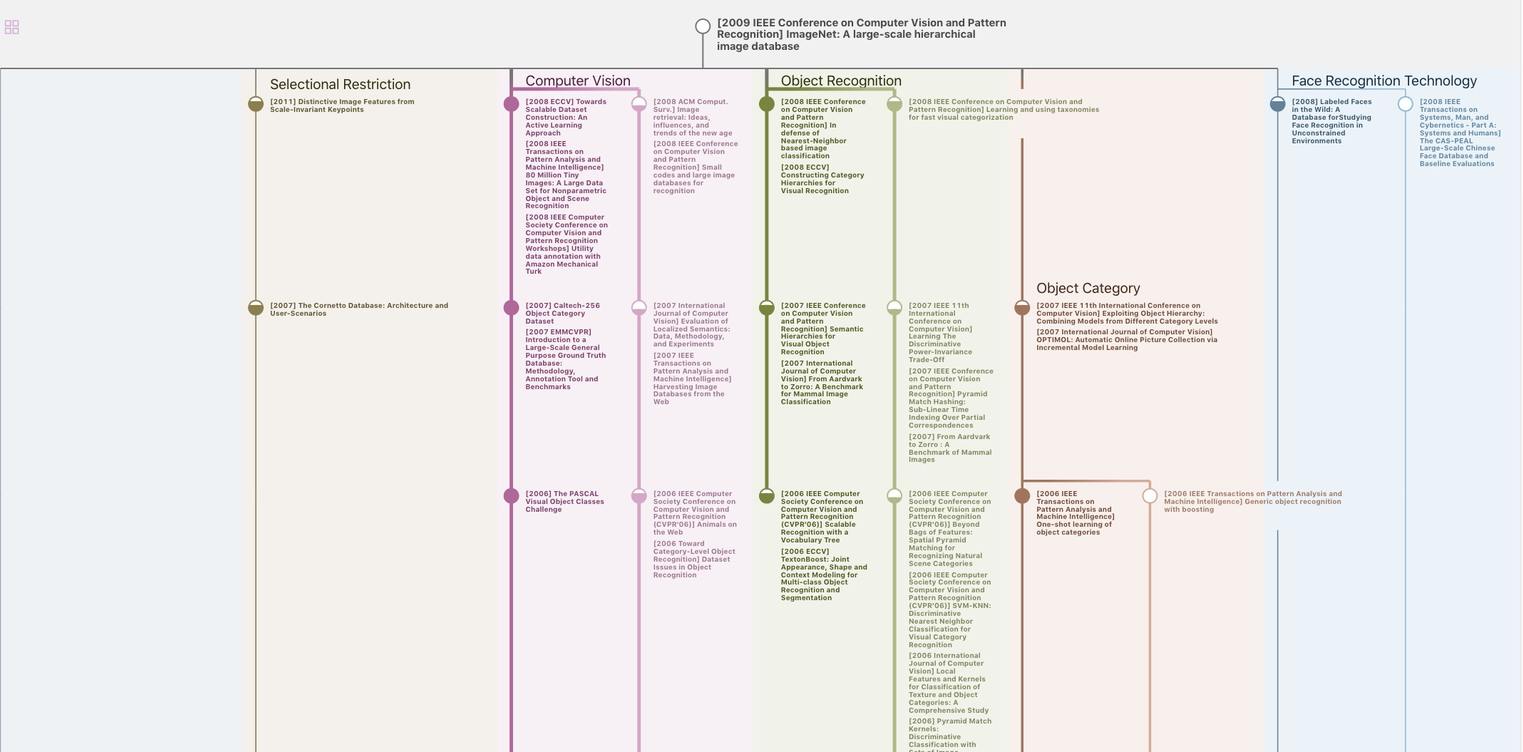
生成溯源树,研究论文发展脉络
Chat Paper
正在生成论文摘要