Learning rates of multi-kernel regression by orthogonal greedy algorithm
Journal of Statistical Planning and Inference(2013)
摘要
We investigate the problem of regression from multiple reproducing kernel Hilbert spaces by means of orthogonal greedy algorithm. The greedy algorithm is appealing as it uses a small portion of candidate kernels to represent the approximation of regression function, and can greatly reduce the computational burden of traditional multi-kernel learning. Satisfied learning rates are obtained based on the Rademacher chaos complexity and data dependent hypothesis spaces.
更多查看译文
关键词
Sparse,Multi-kernel learning,Orthogonal greedy algorithm,Data dependent hypothesis space,Rademacher chaos complexity,Learning rate
AI 理解论文
溯源树
样例
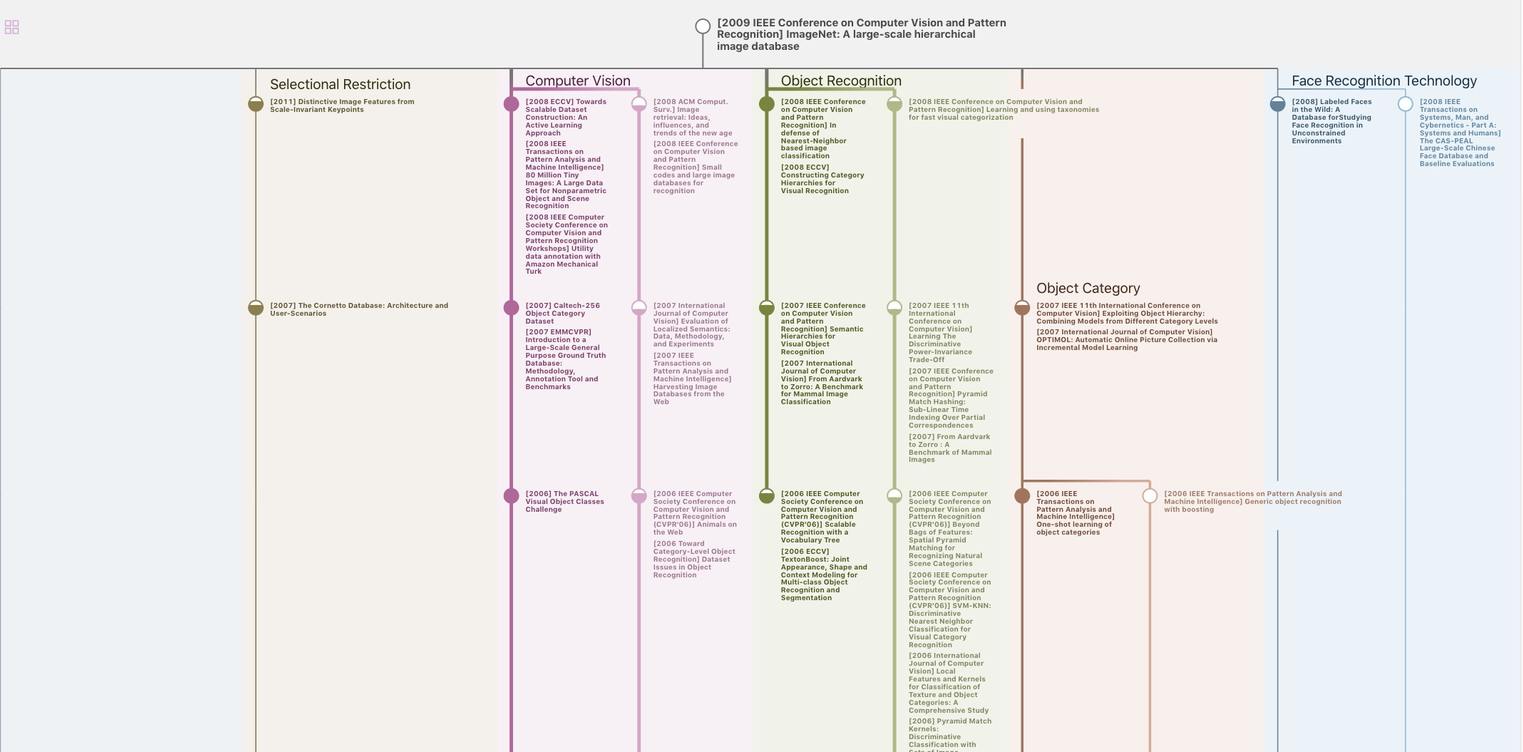
生成溯源树,研究论文发展脉络
Chat Paper
正在生成论文摘要