Exploiting the Use of Convolutional Neural Networks for Localization in Indoor Environments.
Applied Artificial Intelligence(2017)
摘要
Indoor localization has been an active research area for the last two decades. A great number of sensors have been applied in the task of localization—some with high computational and energy demands e.g. laser beams, or with issues related to the coverage area, for example, by making use of images obtained by a network of cameras. A different approach, which presents less energy demands and a wide area of coverage, can be created by means of the signal strength of wireless networks. The open issue with signal strength is its high instability due to interferences, attenuation and fading, which, in general, makes the localization systems to present less than desired accuracy. In this article, we exploit the use of Convolutional Neural Networks ConvNets in the task of localization. The main motivation behind the employment of ConvNets is its inherent ability of feature extraction, which we believe can deal better with the noise without a filtering step. We evaluate how ConvNets can be employed and identify the best topologies that lead to the lowest errors.
Indoor localization has been an active research area for the last two decades. A great number of sensors have been applied in the task of localization—some with high computational and energy demands e.g. laser beams, or with issues related to the coverage area, for example, by making use of images obtained by a network of cameras. A different approach, which presents less energy demands and a wide area of coverage, can be created by means of the signal strength of wireless networks. The open issue with signal strength is its high instability due to interferences, attenuation and fading, which, in general, makes the localization systems to present less than desired accuracy. In this article, we exploit the use of Convolutional Neural Networks ConvNets in the task of localization. The main motivation behind the employment of ConvNets is its inherent ability of feature extraction, which we believe can deal better with the noise without a filtering step. We evaluate how ConvNets can be employed and identify the best topologies that lead to the lowest errors.
更多查看译文
关键词
convolutional neural networks,localization,neural networks
AI 理解论文
溯源树
样例
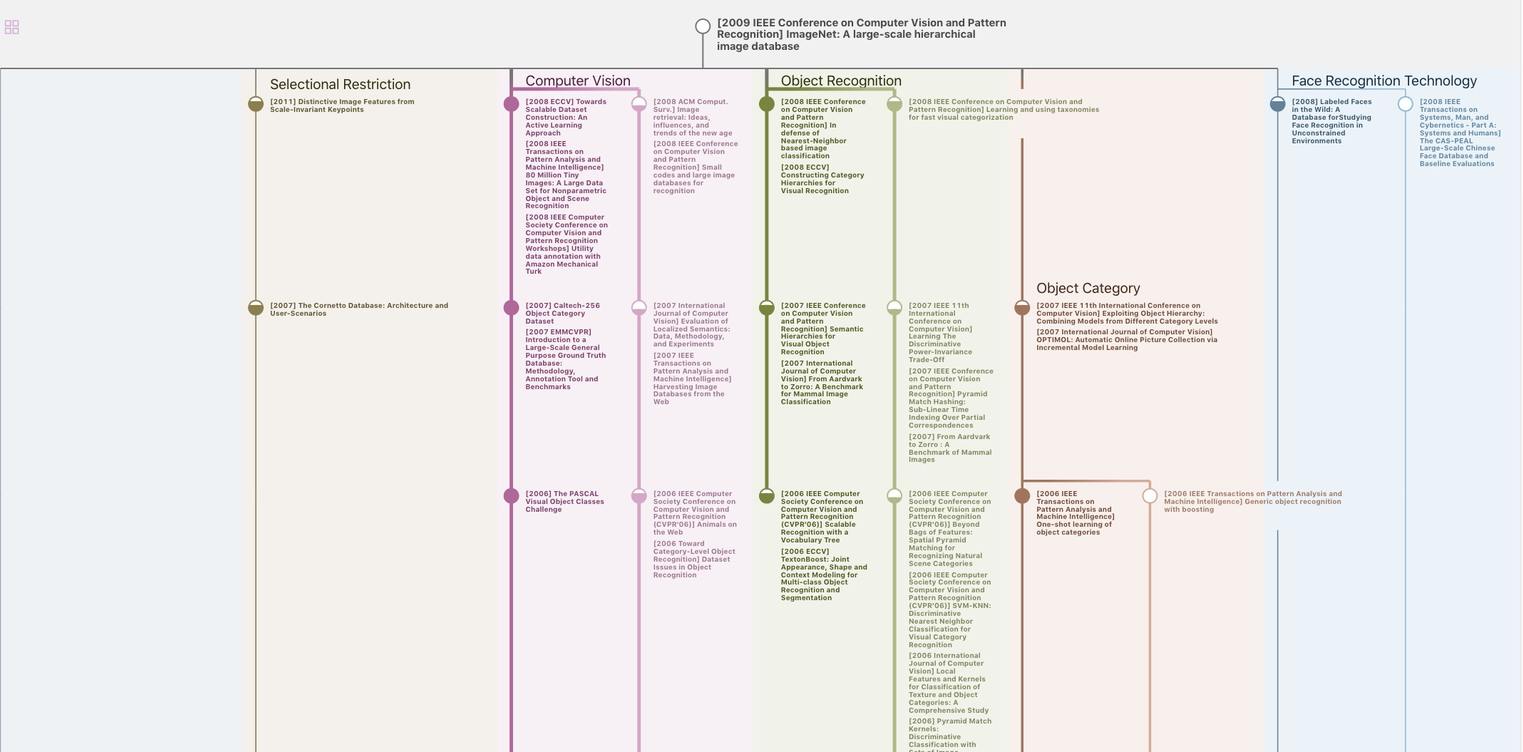
生成溯源树,研究论文发展脉络
Chat Paper
正在生成论文摘要