Image denoising by random walk with restart Kernel and non-subsampled contourlet transform
Signal Processing, IET(2012)
摘要
To address the drawbacks of continuous partial differential equations, a diffusion method based on spectral graph theory and random walk with restart kernel is proposed, which uses non-subsampled contourlet transform to capture the geometric feature of image. Specifically, a new graph weighting function is constructed based on the geometric feature. Moreover, a second-order random walk with restart kernel was generated. The derivation shows that the proposed method is equivalent to the denoising methods based on partial differential equations. The simulation results demonstrate that the proposed method can effectively reduce Gaussian noise and preserve image edge with superior performance compared with other graph-based partial differential equation methods. © 2012 The Institution of Engineering and Technology.
更多查看译文
关键词
random walk,gaussian noise,graph theory,spectral graph theory,partial differential equations
AI 理解论文
溯源树
样例
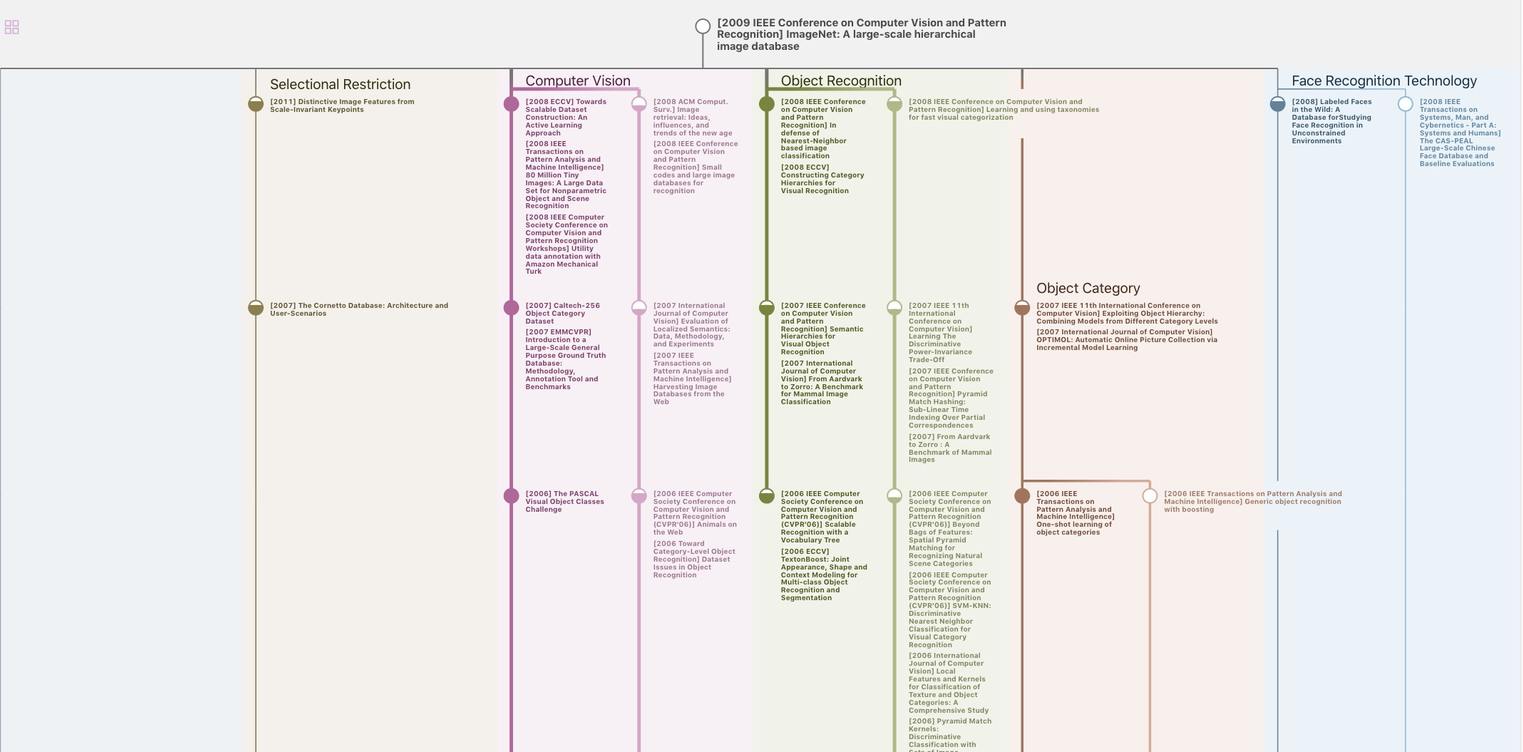
生成溯源树,研究论文发展脉络
Chat Paper
正在生成论文摘要