An Edge-Assisted Computing and Mask Attention Based Network for Lung Region Segmentation
INTERNATIONAL JOURNAL OF INTELLIGENT SYSTEMS(2023)
摘要
Recent years have witnessed the success of encoder-decoder structure-based approaches in lung region segmentation of chest X-ray (CXR) images. However, accurate lung region segmentation is still challenging due to the following three issues: (1) inaccurate lung region segmentation boundaries, (2) existence of lesion-related artifacts (e.g., opacity and pneumonia), and (3) lack of the ability to utilize multiscale information. To address these issues, we propose an edge-assisted computing and mask attention based network (called EAM-Net), which consists of an encoder-decoder network, an edge-assisted computing module, and multiple mask attention modules. Based on the encoder-decoder structure, an edge-assisted computing module is first proposed, which integrates the feature maps of the shallow encoding layers for edge prediction, and uses the edge evidence map as a strong cue to guide the lung region segmentation, thereby refining the lung region segmentation boundaries. We further design a mask attention module after each decoding layer, which employs a mask attention operation to make the model focus on lung regions while suppressing the lesion-related artifacts. Besides, a multiscale aggregation loss is proposed to optimize EAM-Net. Extensive experiments on the JSRT, Shenzhen, and Montgomery datasets demonstrate that EAM-Net outperforms existing state-of-the-art lung region segmentation methods.
更多查看译文
关键词
mask attention based network,lung,segmentation,edge-assisted
AI 理解论文
溯源树
样例
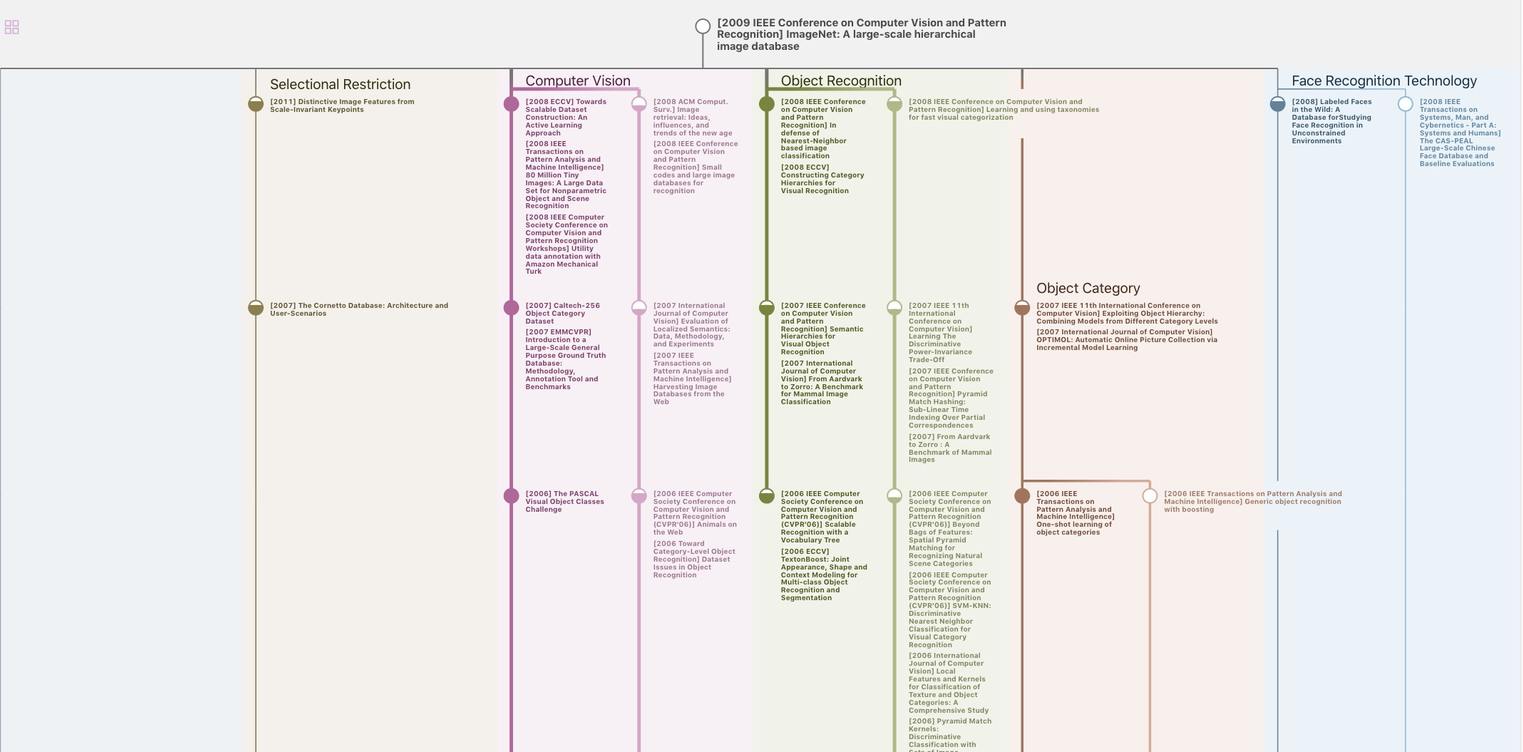
生成溯源树,研究论文发展脉络
Chat Paper
正在生成论文摘要