FBPFormer: Dynamic Convolutional Transformer for Global-Local-Contexual Facial Beauty Prediction
ARTIFICIAL NEURAL NETWORKS AND MACHINE LEARNING, ICANN 2023, PART X(2023)
Abstract
Facial Beauty Prediction (FBP) is subjective and varies from person to person, which makes it difficult to obtain a unified and objective evaluation. Previous efforts adopt conventional convolution neural networks to extract local facial features and calculate corresponding facial attractiveness scores, ignoring the global facial features. To address this issue, we propose a dynamic convolution vision transformer named FBPFormer which aims to focus on both local facial features and the global facial information of the human face. Specifically, we first build a lightweight convolution network to produce pseudo facial attribute embedding. To inject the global facial information into the transformer, the parameters of encoders are dynamically generated by the embedding of each instance. Therefore, these dynamic encoders can fuse and further fuse local facial features and global facial information while encoding query, key, and value vectors. Furthermore, we design an instance-level dynamic exponential loss to dynamically adjust the optimization objectives of the model. Extensive experiments show our method achieves competitive performance, demonstrating its effectiveness in the FBP task.
MoreTranslated text
Key words
Face beauty prediction,Vision transformer,Dynamic convolution
AI Read Science
Must-Reading Tree
Example
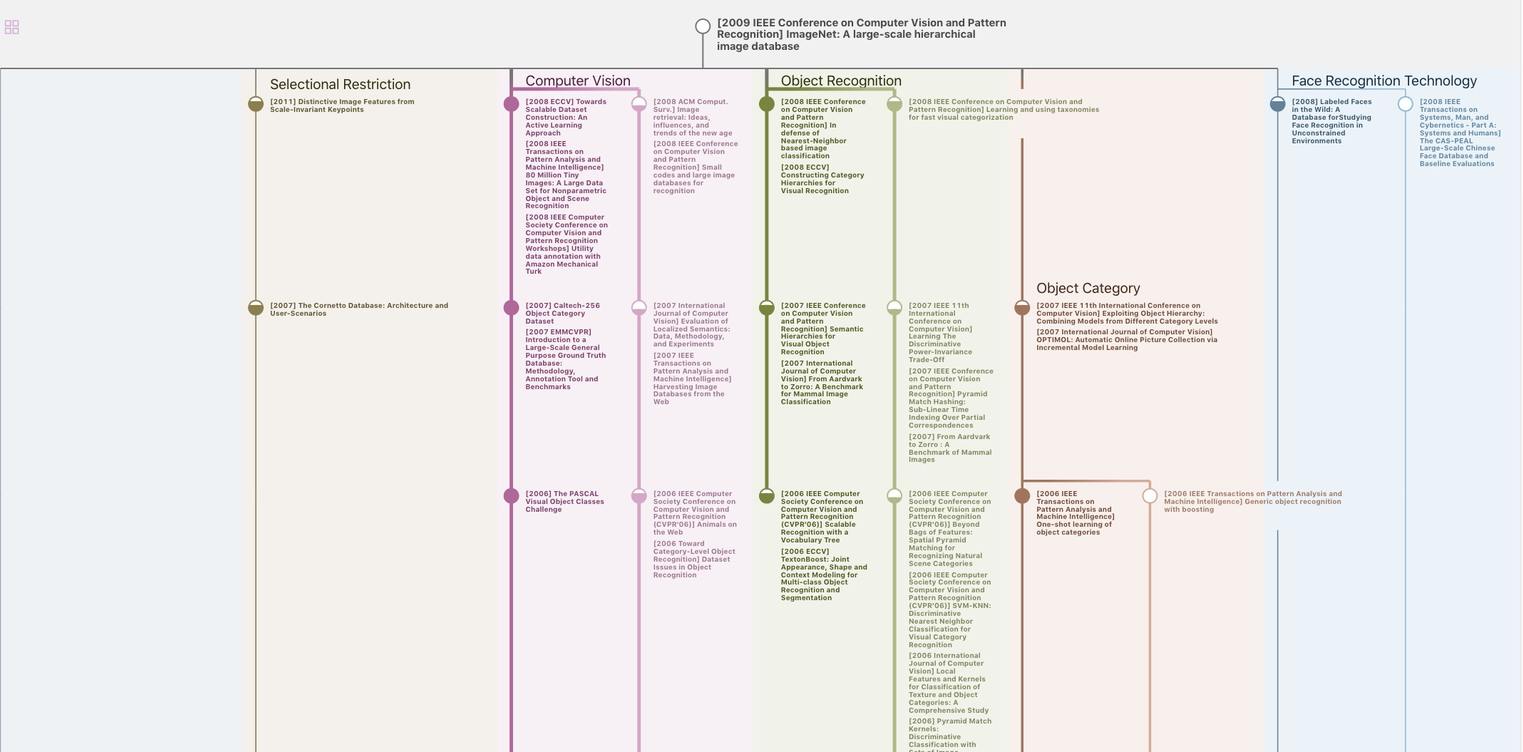
Generate MRT to find the research sequence of this paper
Chat Paper
Summary is being generated by the instructions you defined