Phone-to-Audio Alignment without Text: A Semi-Supervised Approach.
IEEE International Conference on Acoustics, Speech, and Signal Processing (ICASSP)(2022)
Abstract
The task of phone-to-audio alignment has many applications in speech research. Here we introduce two Wav2Vec2-based models for both text-dependent and text-independent phone-to-audio alignment. The proposed Wav2Vec2-FS, a semi-supervised model, directly learns phone-to-audio alignment through contrastive learning and a forward sum loss, and can be coupled with a pretrained phone recognizer to achieve text-independent alignment. The other model, Wav2Vec2-FC, is a frame classification model trained on forced aligned labels that can both perform forced alignment and text-independent segmentation. Evaluation results suggest that both proposed methods, even when transcriptions are not available, generate highly close results to existing forced alignment tools. Our work presents a neural pipeline of fully automated phone-to-audio alignment. Code and pretrained models are available at https://github.com/lingjzhu/charsiu.
MoreTranslated text
Key words
forced alignment,phone segmentation,deep learning,automatic speech recognition
AI Read Science
Must-Reading Tree
Example
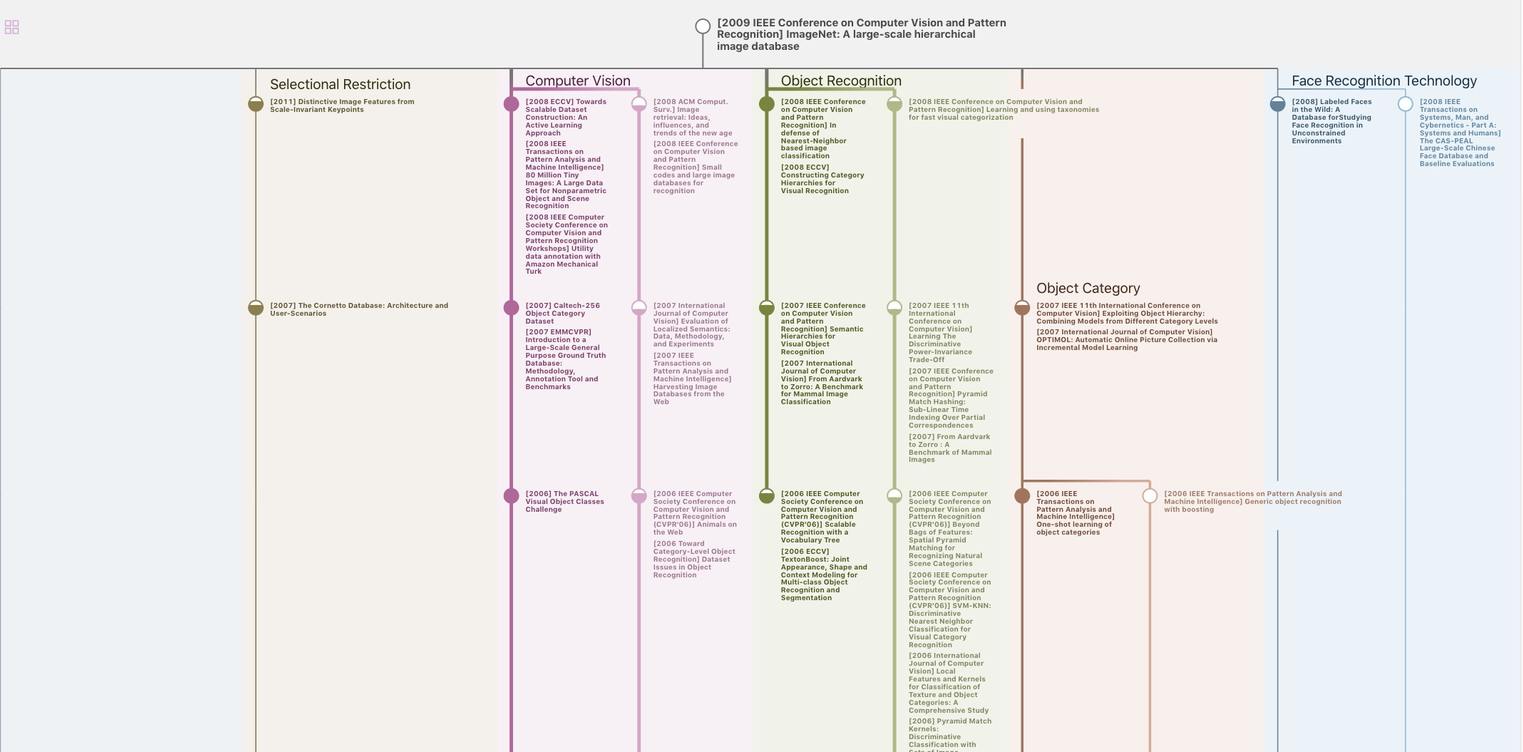
Generate MRT to find the research sequence of this paper
Chat Paper
Summary is being generated by the instructions you defined