KW-ATTN - Knowledge Infused Attention for Accurate and Interpretable Text Classification.
DeeLIO@NAACL-HLT(2021)
Abstract
Text classification has wide-ranging applications in various domains. While neural network approaches have drastically advanced performance in text classification, they tend to be powered by a large amount of training data, and interpretability is often an issue. As a step towards better accuracy and interpretability especially on small data, in this paper we present a new knowledge-infused attention mechanism, called KW-ATTN (KnoWledge-infused ATTentioN) to incorporate high-level concepts from external knowledge bases into Neural Network models. We show that KW-ATTN outperforms baseline models using only words as well as other approaches using concepts by classification accuracy, which indicates that high-level concepts help model prediction. Furthermore, crowdsourced human evaluation suggests that additional concept information helps interpretability of the model.
MoreTranslated text
Key words
interpretable text classification,knowledge infused attention,kw-attn
AI Read Science
Must-Reading Tree
Example
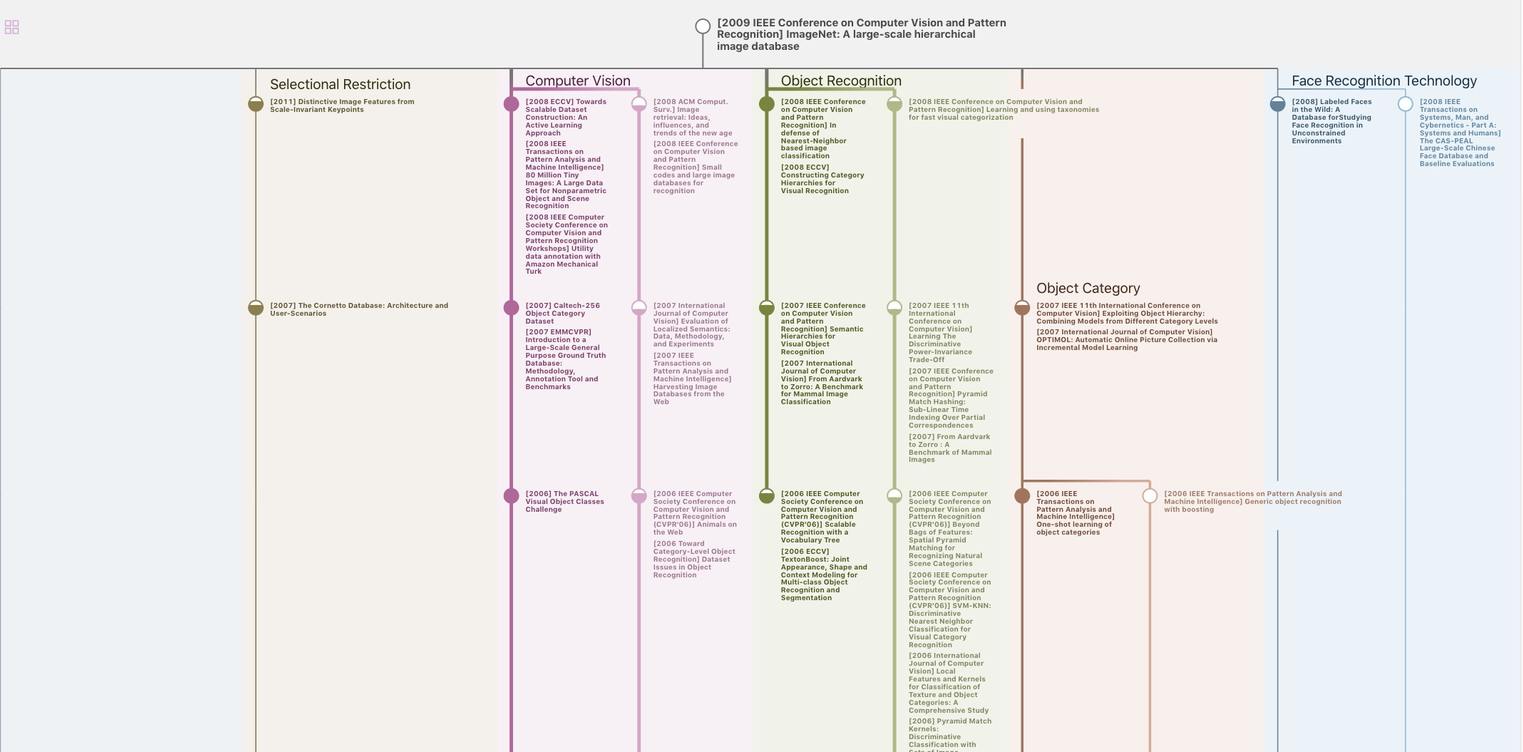
Generate MRT to find the research sequence of this paper
Chat Paper
Summary is being generated by the instructions you defined