Online query relaxation via Bayesian causal structures discovery
AAAI(2005)
摘要
We introduce a novel algorithm, TOQR, for relaxing failed queries over databases, that is, over-constrained DNF queries that return an empty result. TOQR uses a small dataset to discover the implicit relationships among the domain attributes, and then it exploits this domain knowledge to relax the failed query. TOQR starts with a relaxed query that does not include any constraint, and it tries to add to it as many as possible of the original constraints or their relaxations. The order in which the constraints are added is derived from the domain's causal structure, which is learned by applying the TAN algorithm to the small training dataset. Our experiments show that TOQR clearly outperforms other approaches: even when trained on a handful of examples, it successfully relaxes more that 97% of the failed queries; furthermore, TOQR'S relaxed queries are highly similar to the original failed query.
更多查看译文
关键词
tan algorithm,online query relaxation,original constraint,original failed query,failed query,bayesian causal structures discovery,small training dataset,domain attribute,over-constrained dnf query,novel algorithm,domain knowledge,small dataset,bayes theorem,data bases,algorithms,experimental design,queueing theory
AI 理解论文
溯源树
样例
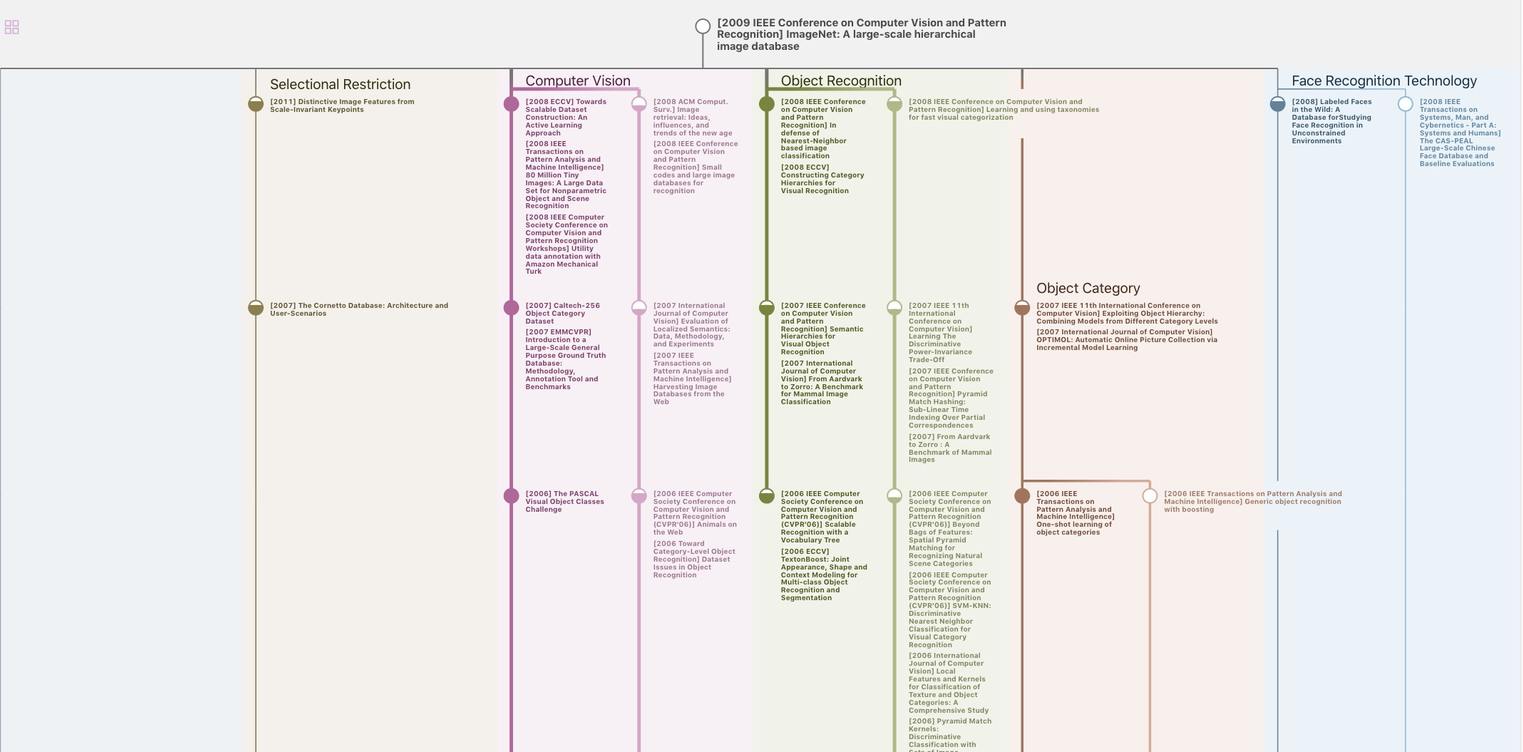
生成溯源树,研究论文发展脉络
Chat Paper
正在生成论文摘要