Convex Point Estimation using Undirected Bayesian Transfer Hierarchies
Uncertainty in Artificial Intelligence(2012)
摘要
When related learning tasks are naturally ar- ranged in a hierarchy, an appealing approach for coping with scarcity of instances is that of trans- fer learning using a hierarchical Bayes frame- work. As fully Bayesian computations can be difficult and computationally demanding, it is of- ten desirable to use posterior point estimates that facilitate (relatively) efficient prediction. How- ever, the hierarchical Bayes framework does not always lend itself naturally to this maximum a- posteriori goal. In this work we propose an undi- rected reformulation of hierarchical Bayes that relies on priors in the form of similarity mea- sures. We introduce the notion of "degree of transfer" weights on components of these sim- ilarity measures, and show how they can be automatically learned within a joint probabilis- tic framework. Importantly, our reformulation results in a convex objective for many learn- ing problems, thus facilitating optimal poste- rior point estimation using standard optimization techniques. In addition, we no longer require proper priors, allowing for flexible and straight- forward specification of joint distributions over transfer hierarchies. We show that our frame- work is effective for learning models that are part of transfer hierarchies for two real-life tasks: ob- ject shape modeling using Gaussian density esti- mation and document classification.
更多查看译文
关键词
relative efficiency,relational learning,point estimation
AI 理解论文
溯源树
样例
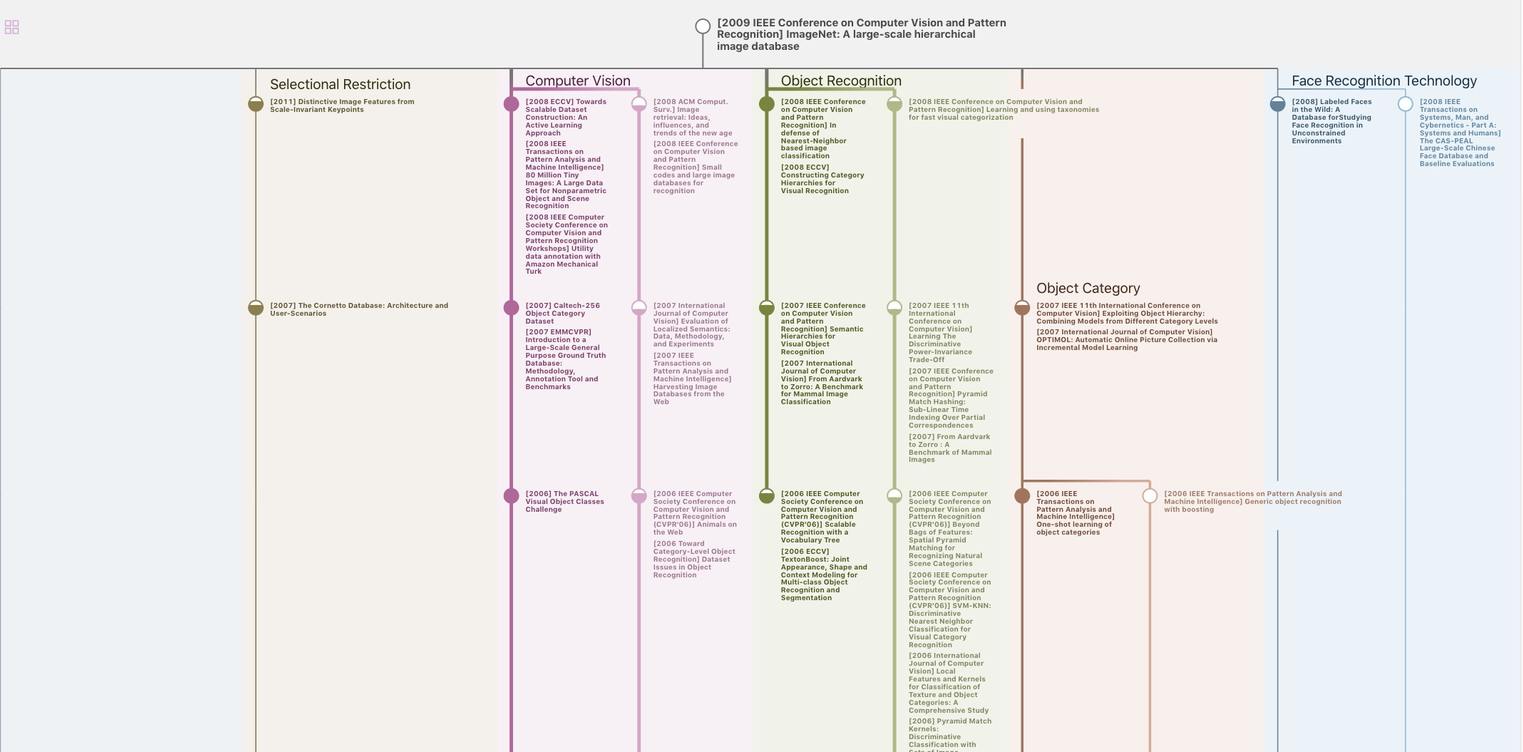
生成溯源树,研究论文发展脉络
Chat Paper
正在生成论文摘要