Estimation for partially nonstationary multivariate autoregressive models with conditional heteroscedasticity
BIOMETRIKA(2001)
摘要
This paper investigates a partially nonstationary multivariate autoregressive model, which allows its innovations to be generated by a multivariate ARCH, autoregressive conditional heteroscedastic, process. Three estimators, including the least squares estimator, a full-rank maximum likelihood estimator and a reduced-rank maximum likelihood estimator, are considered and their asymptotic distributions are derived. When the multivariate ARCH process reduces to the innovation with a constant covariance matrix, these asymptotic distributions are the same as those given by Alin & Reinsel (1990). However, in the presence of multivariate ARCH innovations, the asymptotic distributions of the full-rank maximum likelihood estimator and the reduced-rank maximum likelihood estimator involve two correlated multivariate Brownian motions, which are different from those given by Alin & Reinsel (1990). Simulation results show that the full-rank and reduced-rank maximum likelihood estimator are more efficient than the least squares estimator. An empirical example shows that the two features of multivariate conditional heteroscedasticity and partial nonstationarity may be present simultaneously in a multivariate time series.
更多查看译文
关键词
Brownian motion,cointegration,full-rank and reduced-rank maximum likelihood,estimators,least squares estimator,multivariate ARCH process,partially nonstationary,unit root
AI 理解论文
溯源树
样例
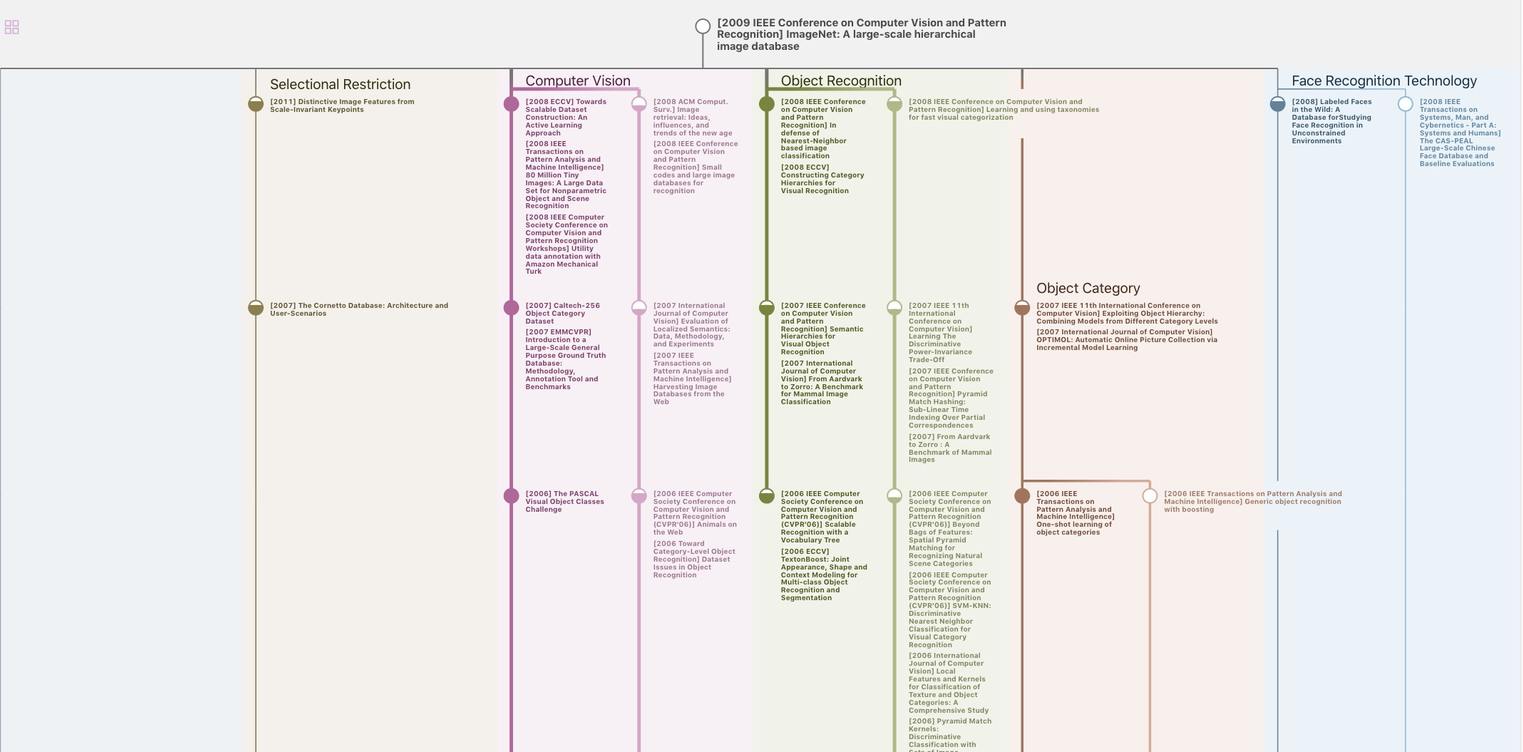
生成溯源树,研究论文发展脉络
Chat Paper
正在生成论文摘要