26. Improving interoperability of therapeutics and their targets for clinical and precision medicine applications
Cancer Genetics(2023)
摘要
Contemporary genomic medicine pipelines are enabled by integration of genomic and therapeutic knowledge bases for clinical decision support systems. Human curators are expected to synthesize these data to predict how patient tumors will respond to potential therapeutics. In some tumors, the presence of a single mutation can be enough to promote sensitivity to a particular treatment. While gene specific drug interaction information is readily available through many resources, therapeutic ontologies and vocabularies supporting these data are not well aligned. In addition, many resources do not have structured concept identifiers associated with therapeutic terms, instead describing them as free-text names. For such resources, the task of linking therapeutic vocabulary to other sources becomes largely reliant on the error-prone process of free text string matching. The same therapeutic concept can hold a handful of valid identifiers across different research domains- from molecular structures to brand names to therapeutic formulations. We have addressed these challenges through development of a Python-based therapy normalization library (TheraPy). We demonstrate how use of this tool enables extraction of relevant therapeutic knowledge from the literature using Natural Language Processing (NLP) techniques. These tools also provide a framework for enriching therapeutic concepts with regulatory approval status from the FDA. We leveraged this tool to harmonize data across 40 resources of the Drug-Gene Interaction database (DGIdb), and demonstrate how this work improves our ability to find and link therapeutic concepts across resources. Contemporary genomic medicine pipelines are enabled by integration of genomic and therapeutic knowledge bases for clinical decision support systems. Human curators are expected to synthesize these data to predict how patient tumors will respond to potential therapeutics. In some tumors, the presence of a single mutation can be enough to promote sensitivity to a particular treatment. While gene specific drug interaction information is readily available through many resources, therapeutic ontologies and vocabularies supporting these data are not well aligned. In addition, many resources do not have structured concept identifiers associated with therapeutic terms, instead describing them as free-text names. For such resources, the task of linking therapeutic vocabulary to other sources becomes largely reliant on the error-prone process of free text string matching. The same therapeutic concept can hold a handful of valid identifiers across different research domains- from molecular structures to brand names to therapeutic formulations. We have addressed these challenges through development of a Python-based therapy normalization library (TheraPy). We demonstrate how use of this tool enables extraction of relevant therapeutic knowledge from the literature using Natural Language Processing (NLP) techniques. These tools also provide a framework for enriching therapeutic concepts with regulatory approval status from the FDA. We leveraged this tool to harmonize data across 40 resources of the Drug-Gene Interaction database (DGIdb), and demonstrate how this work improves our ability to find and link therapeutic concepts across resources.
更多查看译文
关键词
precision medicine applications,interoperability,therapeutics,clinical
AI 理解论文
溯源树
样例
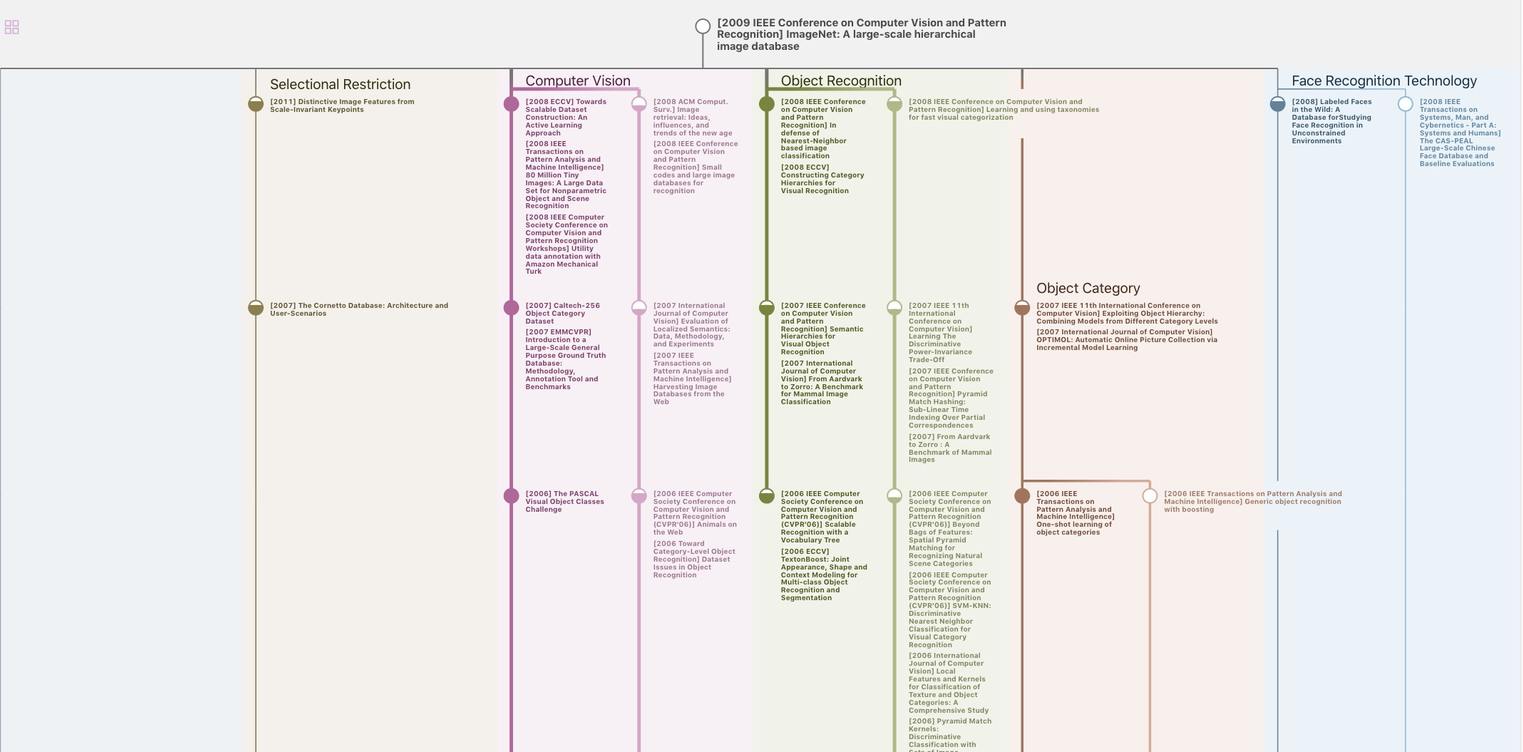
生成溯源树,研究论文发展脉络
Chat Paper
正在生成论文摘要