Lower And Upper Bounds For Approximation Of The Kullback-Leibler Divergence Between Gaussian Mixture Models
ICASSP(2012)
摘要
Many speech technology systems rely on Gaussian Mixture Models (GMMs). The need for a comparison between two GMMs arises in applications such as speaker verification, model selection or parameter estimation. For this purpose, the Kullback-Leibler (KL) divergence is often used. However, since there is no closed form expression to compute it, it can only be approximated. We propose lower and upper bounds for the KL divergence, which lead to a new approximation and interesting insights into previously proposed approximations. An application to the comparison of speaker models also shows how such approximations can be used to validate assumptions on the models.
更多查看译文
关键词
Gaussian Mixture Model (GMM),Kullback-Leibler Divergence,speaker comparison,speech processing
AI 理解论文
溯源树
样例
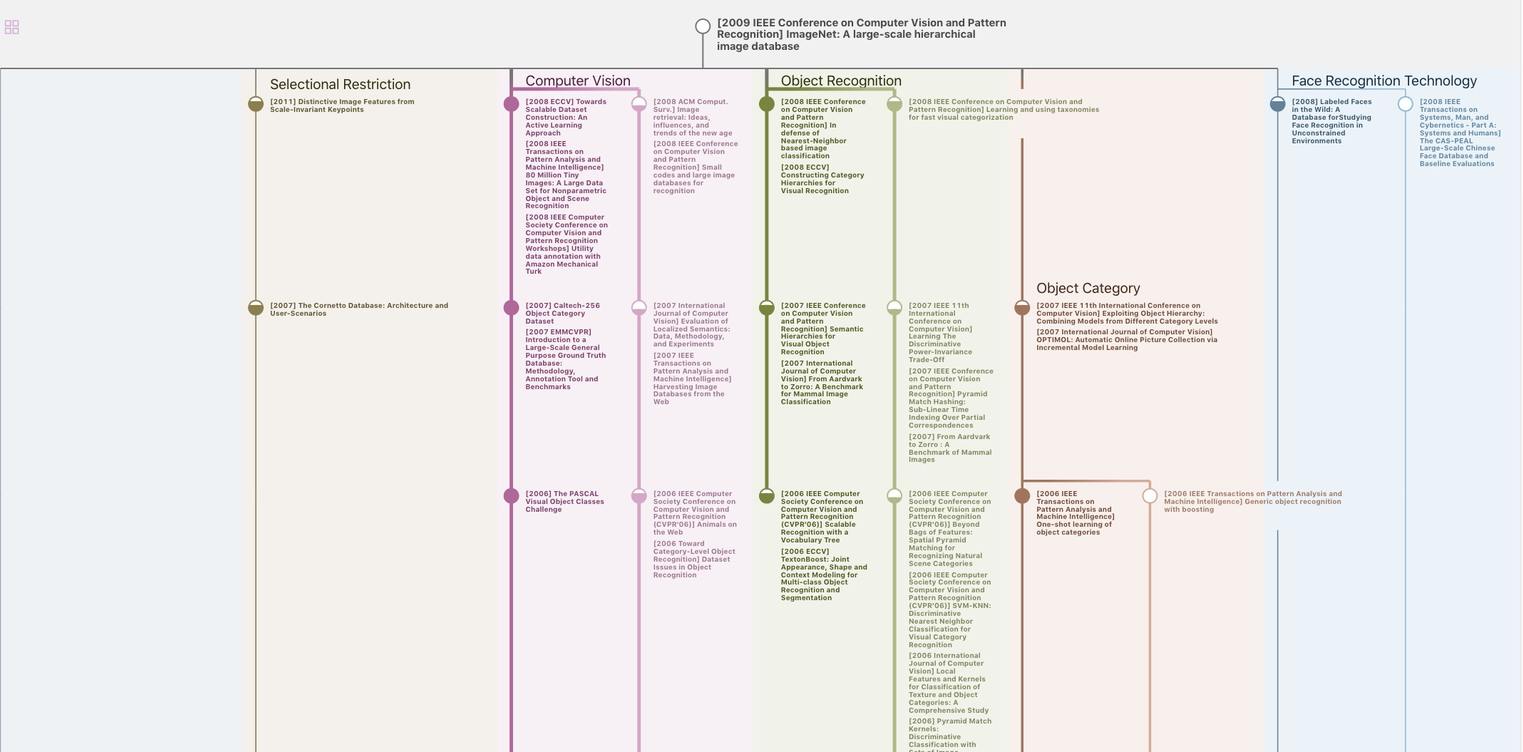
生成溯源树,研究论文发展脉络
Chat Paper
正在生成论文摘要