Contextual Occupancy Maps Incorporating Sensor And Location Uncertainty
Robotics and Automation(2010)
摘要
This paper describes a method of incorporating sensor and localisation uncertainty into contextual occupancy maps to provide for robust mapping. This paper builds on a recently proposed application of the Gaussian process (GP) to occupancy mapping. An extension of GPs is employed which incorporates uncertain inputs into the covariance function. In turn, this allows statistically consistent, multi-resolution maps to be constructed which exploit the spatial inference properties of GPs while correctly accounting for sensor and localisation errors. Experiments are described, with both synthetic and real data, which show the benefits of complete uncertainty modeling and how contextual occupancy maps may be constructed by fusing data from different sensors on different robots in a common probabilistic representation.
更多查看译文
关键词
Gaussian processes,covariance analysis,inference mechanisms,mobile robots,sensor fusion,uncertainty handling,Gaussian process,contextual occupancy map,covariance function,data fusion,multiresolution map,robot,robust mapping,sensor,spatial inference properties
AI 理解论文
溯源树
样例
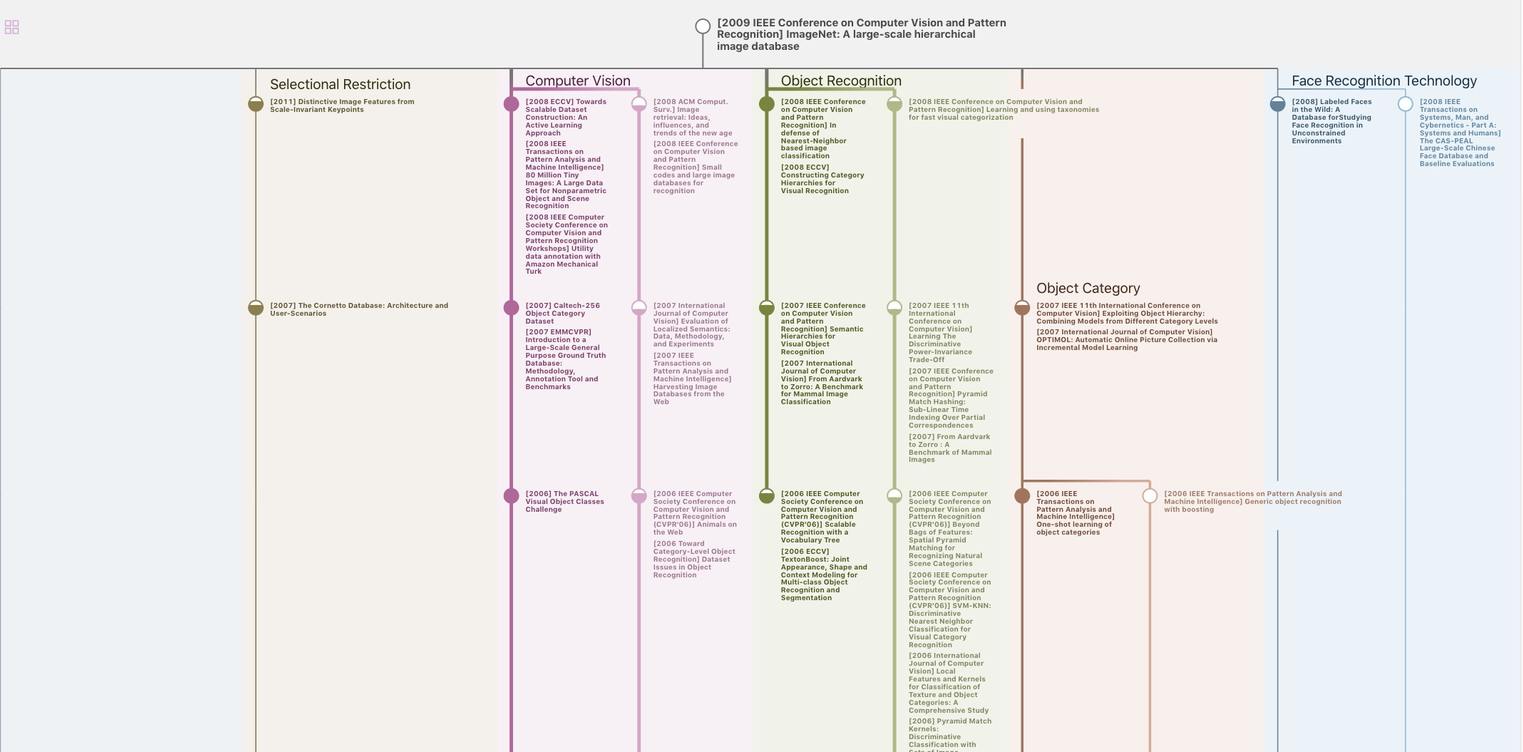
生成溯源树,研究论文发展脉络
Chat Paper
正在生成论文摘要