Trainable TV- L^1 model as recurrent nets for low-level vision
Neural Computing and Applications(2020)
摘要
TV- L^1 is a classical diffusion–reaction model for low-level vision tasks, which can be solved by a duality-based iterative algorithm. Considering the recent success of end-to-end learned representations, we propose a TV-LSTM network to unfold the duality-based iterations of TV- L^1 into long short-term memory (LSTM) cells. In particular, we formulate the iterations as customized layers of a LSTM neural network. Then, the proposed end-to-end trainable TV-LSTMs can be naturally connected with various task-specific networks, e.g., optical flow, image decomposition and event-based optical flow estimation. Extensive experiments on optical flow estimation and structure + texture decomposition have demonstrated the effectiveness and efficiency of the proposed method.
更多查看译文
关键词
Total variation,Optical flow,Recurrent network,Image decomposition
AI 理解论文
溯源树
样例
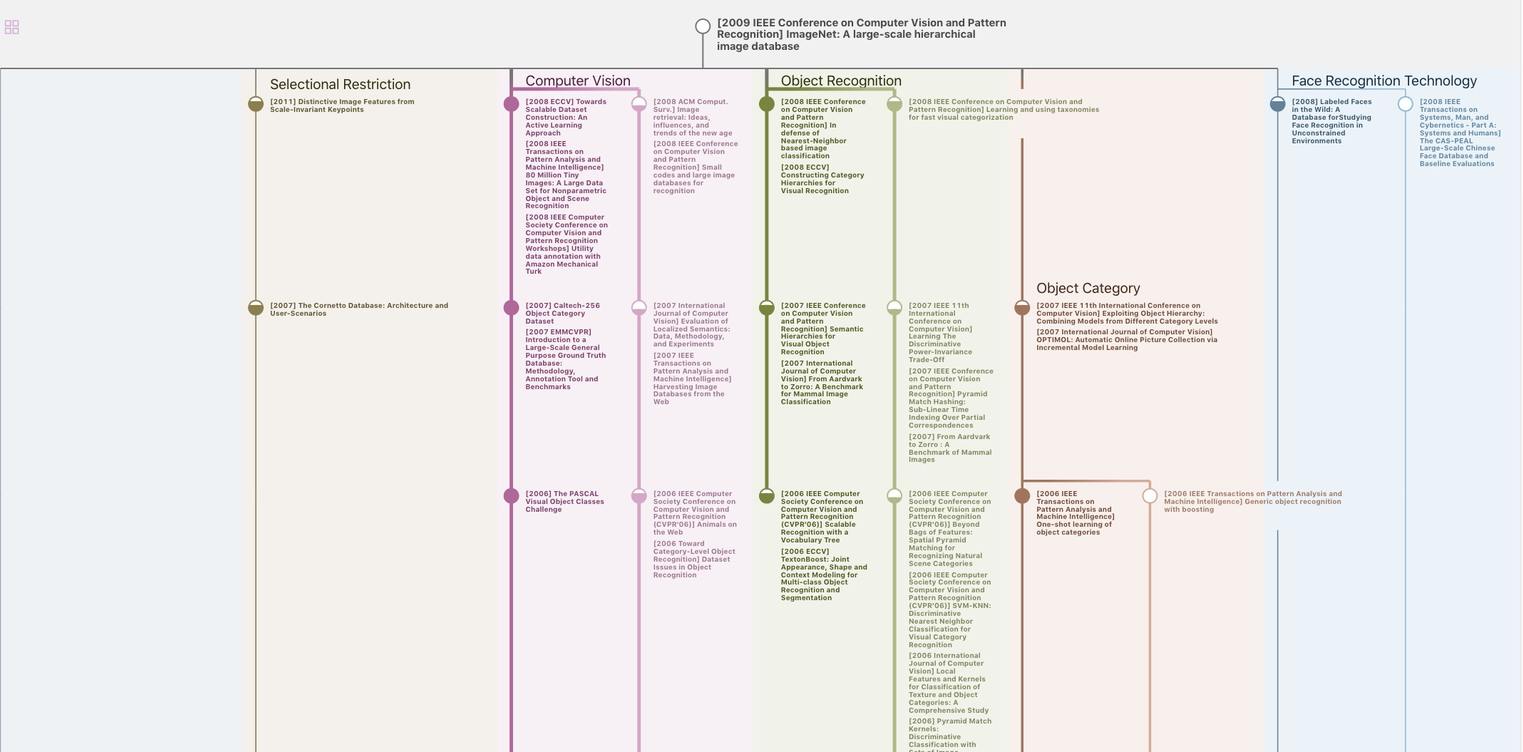
生成溯源树,研究论文发展脉络
Chat Paper
正在生成论文摘要