Traffic Prediction Using A Generic Self-Evolving Takagi-Sugeno-Kang (Gsetsk) Fuzzy Neural Network
2012 INTERNATIONAL JOINT CONFERENCE ON NEURAL NETWORKS (IJCNN)(2012)
摘要
This paper analyses traffic prediction based on a Generic Self-Evolving Takagi-Sugeno-Kang (GSETSK) fuzzy neural network. Traffic prediction is a problem that requires online adaptive systems with high accuracy performance. The proposed GSETSK framework can learn incrementally with high accuracy without any prior assumption about the data sets. To keep an up-to-date fuzzy rule base, a novel 'gradual'-forgetting-based rule pruning approach is proposed to unlearn outdated data by deleting obsolete rules. Experiments conducted on real-life traffic data confirm the validity of the design and the accuracy performance of the GSETSK system.
更多查看译文
关键词
accuracy,pragmatics,fuzzy set theory,computational modeling,predictive models
AI 理解论文
溯源树
样例
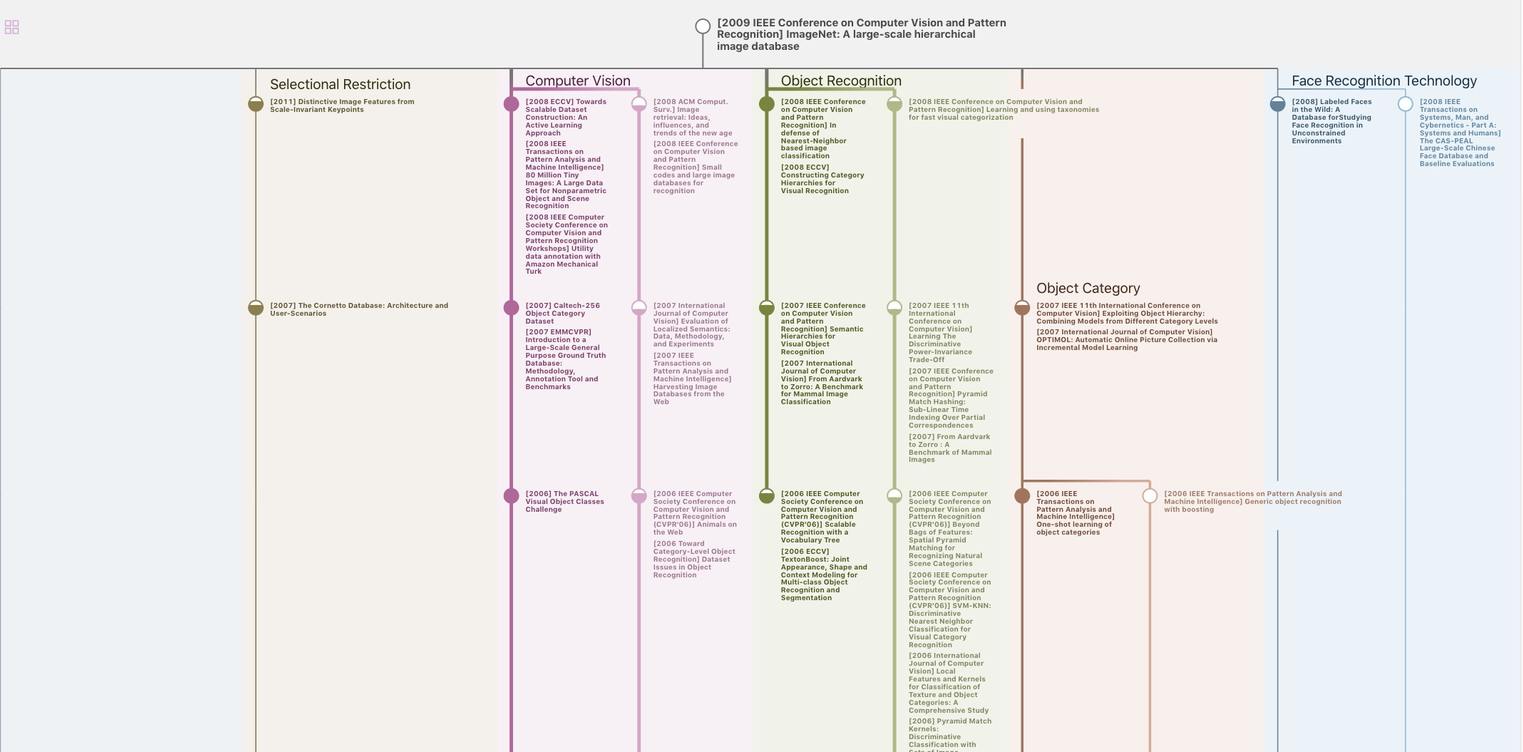
生成溯源树,研究论文发展脉络
Chat Paper
正在生成论文摘要