Does more uniformly distributed sampling generally lead to more accurate prediction in computer experiments?
Winter Simulation Conference(2005)
摘要
Sampling uniformity is one of the central issues for computer experiments or metamodeling. Is it generally true that more uniformly distributed sampling leads to more accurate prediction? A study was conducted to compare four designs for computer experiments, based on simulation tests and statistical analysis. Maximin Latin hypercube design (LHMm) nearly always generated more uniform sampling in two- and three- dimensional cases than does random sampling (Rd), Latin hypercube design (LHD), or Minimized centered L2 discrepancy Latin hypercube design (LHCL2). But often there was no significant difference among the means of the prediction errors by employing LHMm versus the other designs. Occasionally, even the opposite was seen. More uniform sampling did not generally lead to more accurate prediction unless sampling included extremely nonuniform cases, especially when the sample size was relatively small.
更多查看译文
关键词
central issue,accurate prediction,latin hypercube design,uniform sampling,maximin latin hypercube design,l2 discrepancy,random sampling,computer experiment,prediction error,sampling uniformity,metamodeling,three dimensional,sampling methods,statistical analysis,sample size,simulation,computer experiments
AI 理解论文
溯源树
样例
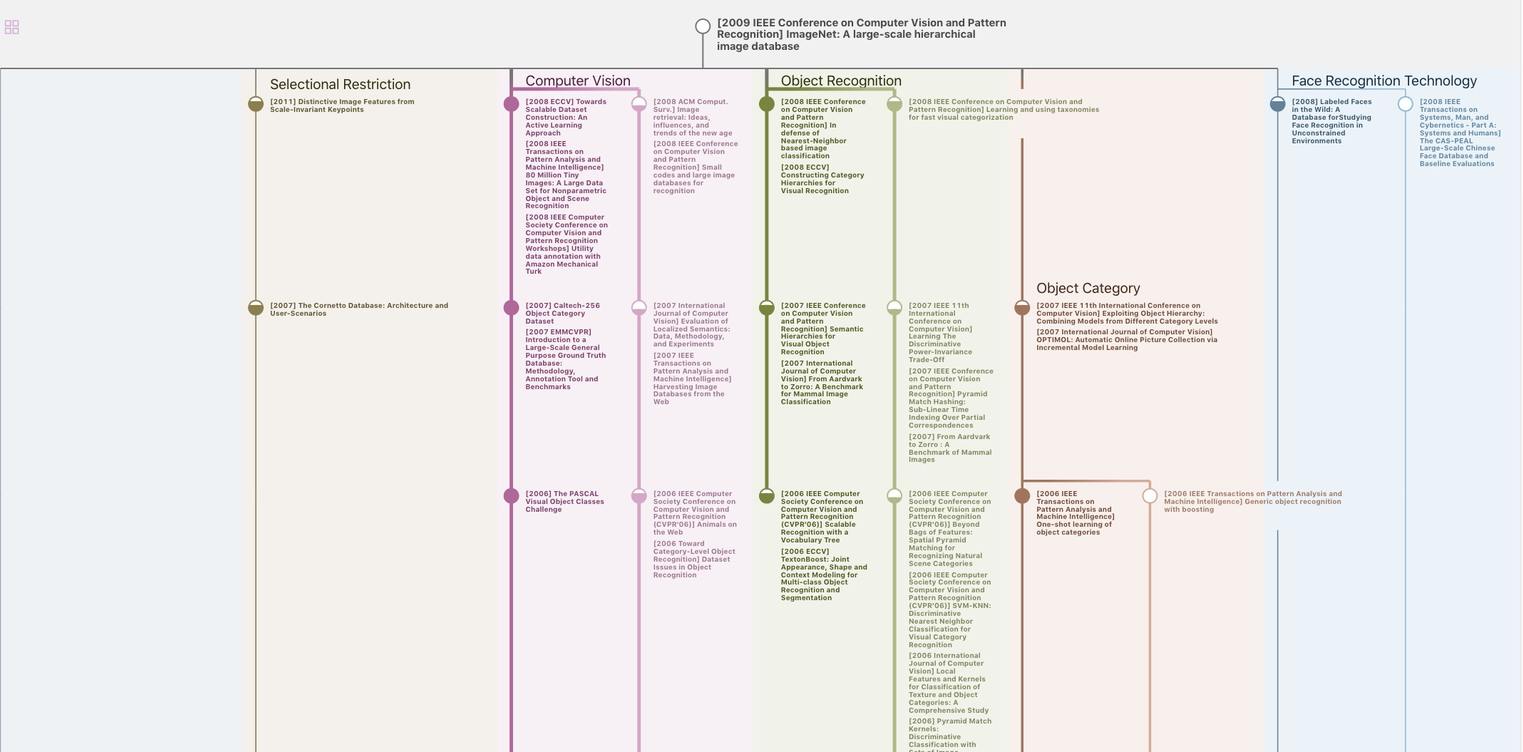
生成溯源树,研究论文发展脉络
Chat Paper
正在生成论文摘要