Learning vector quantization with adaptive metrics for online figure-ground segmentation
Frontiers in Computational Neuroscience(2009)
摘要
Event Abstract Back to Event Learning vector quantization with adaptive metrics for online figure-ground segmentation Alexander Denecke1, 2*, Heiko Wersing2, Jochen Steil1 and Edgar Körner2 1 Bielefeld University, CoR-Lab, P.O. Box 10 01 31, D-33501 Bielefeld, Germany 2 Honda Research Institute Europe, Carl-Legien-Str. 30, D-63073 Offenbach/Main, Germany One classical problem in visual object learning and recognition is figure-ground segmentation, namely the problem to separate the object related regions in the image data from the surrounding background clutter. Figure-ground segmentation is needed in particular for online learning and interaction where the restricted computation time and limited number of available training-data complicate a direct training of the object classifier and generalization to the object parts. To bootstrap the segmentation process we assume that an initial segmentation hypothesis derived from depth information is available. However, a direct usage of this hypothesis for high-performance object learning is not appropriate. Instead this hypothesis is used to build up models for the consistent colors of foreground and background. Using these models for a pixel-wise evaluation afterwards derives a foreground classification that is more consistent with the outline and appearance of the object. We investigate variants of Generalized Learning Vector Quantization (GLVQ) for this purpose. Since similarity based clustering and classification in prototype based networks depends on the underlying metrics, the emphasis lies on the metrics adaptation in this scenario. We model figure and ground by prototypical feature representatives and investigate several metrics extensions for GLVQ (P. Schneider et al., WSOM, 2007) to improve this approach. Comparing those extensions, we show that using prototype-specific linear projections of the feature-space enables an improved foreground generalization (A. Denecke et al., Neurocomputing, 2009). The proposed method can handle arbitrary background, is robust to changes in illumination and real-time capable which yields foreground segmentations that allow for a significant enhancement in object learning and recognition. Further the method is capable to outperform state of the art foreground segmentation methods in our online learning s cenario and achieves competitive results on common benchmark data. Finally, we show that the proposed method imposes fewer constraints (e.g. a priori assumptions about object position and size) on the training data than other methods and is less sensitive to the quality of the initial hypothesis. Vector quantization methods generally are confronted with a model selection problem, namely the number of prototypical feature representatives to model each class. In further work (A. Denecke et al., WSOM, 2009), we address this problem and allow for an adaptive number of prototypes where we have to cope with two problems. Firstly the local adaptive metrics complicates distance-based criteria to place new prototypes, where we use the confidence of the classification instead. Secondly the method has to cope with noisy supervised information, that is, the labels to adapt the networks are not fully consistent. In particular, we address the second problem by using a parallel evaluation method on the basis of a local utility function, which does not rely on global error optimization. On our real world benchmark dataset we show that the incremental network is capable to maintain an adaptive network size and yields a significant smaller variance of the results, and thus is more robust against the initialization of the network. Conference: Bernstein Conference on Computational Neuroscience, Frankfurt am Main, Germany, 30 Sep - 2 Oct, 2009. Presentation Type: Poster Presentation Topic: Abstracts Citation: Denecke A, Wersing H, Steil J and Körner E (2009). Learning vector quantization with adaptive metrics for online figure-ground segmentation. Front. Comput. Neurosci. Conference Abstract: Bernstein Conference on Computational Neuroscience. doi: 10.3389/conf.neuro.10.2009.14.009 Copyright: The abstracts in this collection have not been subject to any Frontiers peer review or checks, and are not endorsed by Frontiers. They are made available through the Frontiers publishing platform as a service to conference organizers and presenters. The copyright in the individual abstracts is owned by the author of each abstract or his/her employer unless otherwise stated. Each abstract, as well as the collection of abstracts, are published under a Creative Commons CC-BY 4.0 (attribution) licence (https://creativecommons.org/licenses/by/4.0/) and may thus be reproduced, translated, adapted and be the subject of derivative works provided the authors and Frontiers are attributed. For Frontiers’ terms and conditions please see https://www.frontiersin.org/legal/terms-and-conditions. Received: 25 Aug 2009; Published Online: 25 Aug 2009. * Correspondence: Alexander Denecke, Bielefeld University, CoR-Lab, P.O. Box 10 01 31, D-33501 Bielefeld, Bielefeld, Germany, adenecke@cor-lab.uni-bielefeld.de Login Required This action requires you to be registered with Frontiers and logged in. To register or login click here. Abstract Info Abstract The Authors in Frontiers Alexander Denecke Heiko Wersing Jochen Steil Edgar Körner Google Alexander Denecke Heiko Wersing Jochen Steil Edgar Körner Google Scholar Alexander Denecke Heiko Wersing Jochen Steil Edgar Körner PubMed Alexander Denecke Heiko Wersing Jochen Steil Edgar Körner Related Article in Frontiers Google Scholar PubMed Abstract Close Back to top Javascript is disabled. Please enable Javascript in your browser settings in order to see all the content on this page.
更多查看译文
关键词
learning vector quantization
AI 理解论文
溯源树
样例
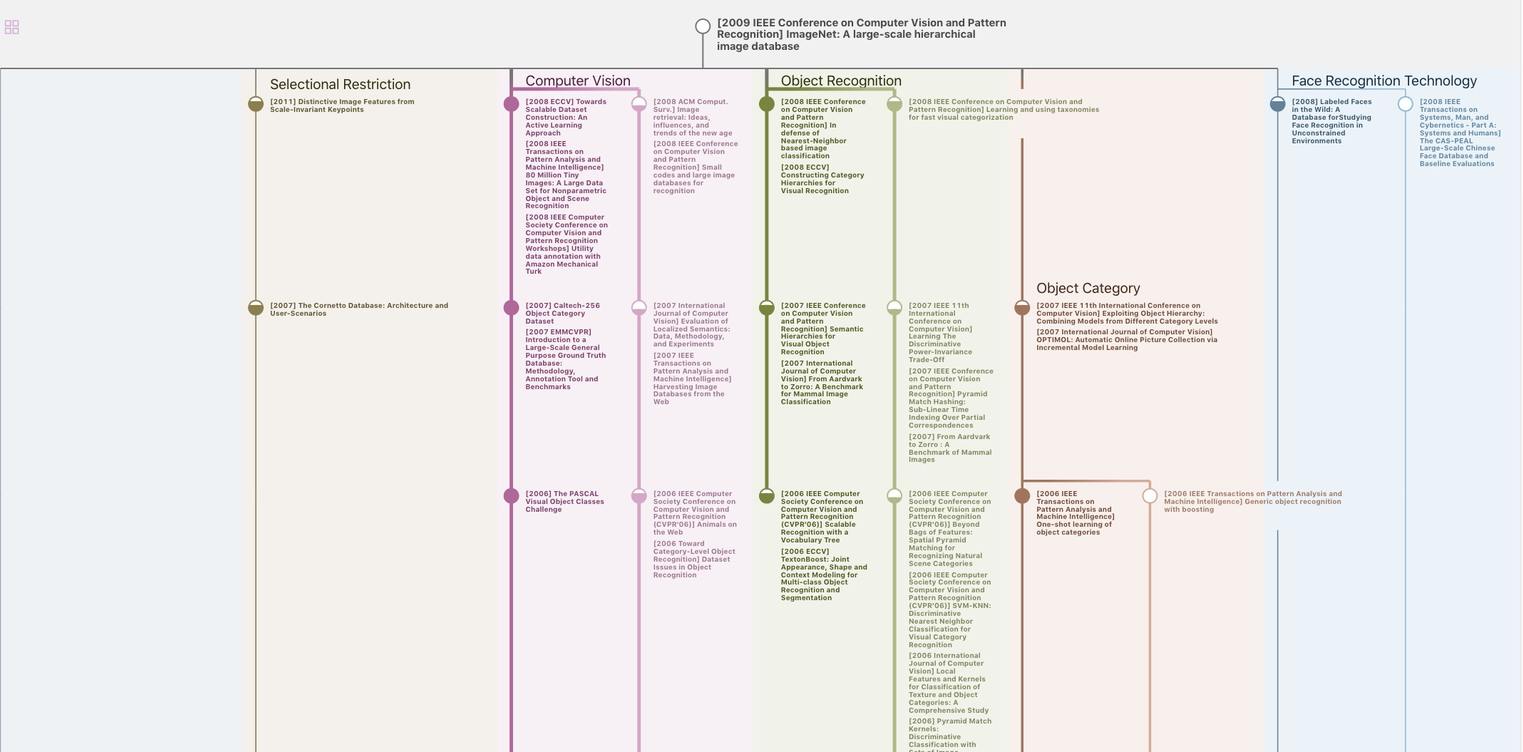
生成溯源树,研究论文发展脉络
Chat Paper
正在生成论文摘要