Discovering Frequent Closed Episodes from an event sequence
IJCNN(2012)
摘要
Serial episode mining is one of hot spots in temporal data mining with broad applications such as user-browsing behavior prediction, telecommunication alarm analysis, road traffic monitoring, and root cause diagnostics from faults log data in manufacturing. In this paper, as a step forward to analyzing patterns within an event sequence, we propose a novel algorithm FCEMiner (Frequent Closed Episodes Miner) for discovering all frequent closed episodes. To characterize the followed-by-closely relationships over event types well and avoid over-counting the support of long episodes, FCEMiner takes both minimal and non-overlapping occurrences of an episode into consideration. To perform iterative episode growth without generating any candidate, FCEMiner utilizes the depth-first search strategy with Apriori Property. To save the cost of post-processing on frequent episodes, FCEMiner checks the closures of some episodes during each valid episode growth. A set of performance studies on both synthetic and real-world datasets show that our algorithm is more efficient and effective.
更多查看译文
关键词
frequent closed episode,pattern recognition,apriori property,pattern analysis,followed-by-closely relationships,tree searching,frequent closed episodes discovery,temporal data mining,minimal,event sequence,depth first search strategy,iterative episode growth,data mining,serial episode mining,minimal occurrences,frequent closed episodes miner algorithm,nonoverlapping occurrences,fceminer algorithm,iterative methods,non-overlapping occurrences,communications technology,iron,algorithm design and analysis,indexes
AI 理解论文
溯源树
样例
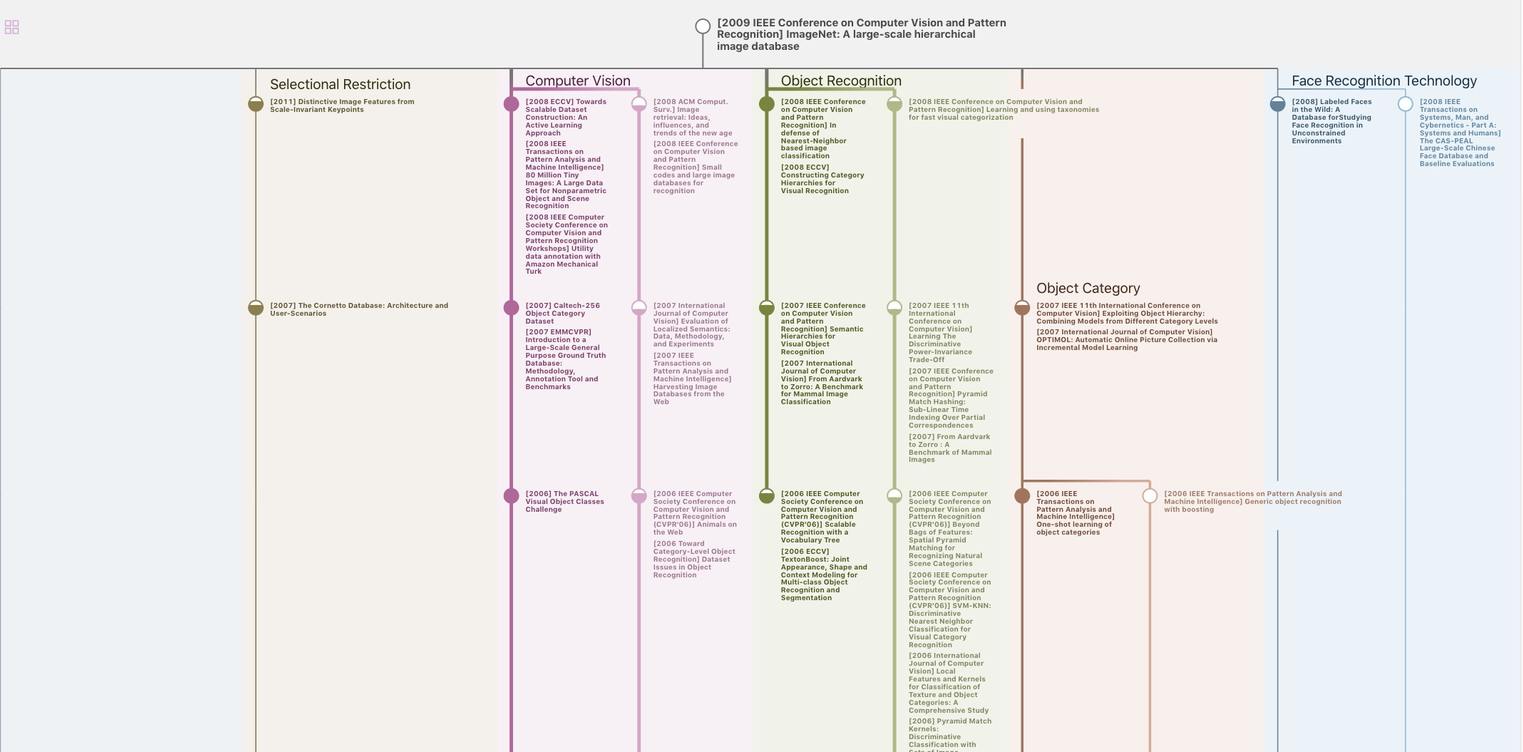
生成溯源树,研究论文发展脉络
Chat Paper
正在生成论文摘要