Learning Lie groups for invariant visual perception
NIPS(1998)
摘要
One of the most important problems in visual perception is that of visual invariance: how are objects perceived to be the same despite undergoing transformations such as translations, rotations or scaling? In this paper, we describe a Bayesian method for learning invariances based on Lie group theory. We show that previous approaches based on first-order Taylor series expansions of inputs can be regarded as special cases of the Lie group approach, the latter being capable of handling in principle arbitrarily large transformations. Using a matrix-exponential based generative model of images, we derive an unsupervised algorithm for learning Lie group operators from input data containing infinitesimal transformations. The on-line unsupervised learning algorithm maximizes the posterior probability of generating the training data. We provide experimental results suggesting that the proposed method can learn Lie group operators for handling reasonably large 1-D translations and 2-D rotations.
更多查看译文
关键词
image processing,first order,computer vision,unsupervised learning,lie group,posterior probability,visual perception,matrix exponential,taylor series expansion,bayesian method
AI 理解论文
溯源树
样例
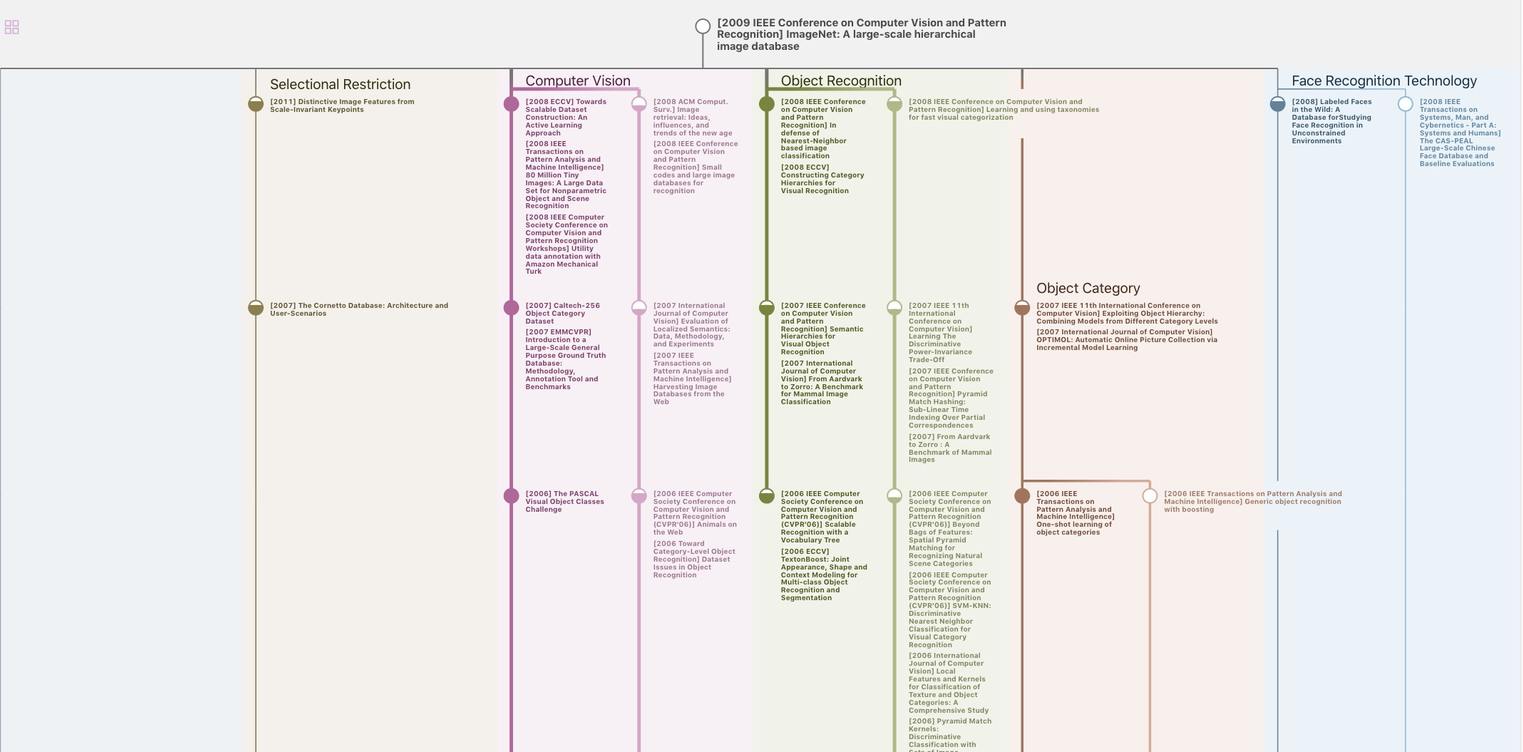
生成溯源树,研究论文发展脉络
Chat Paper
正在生成论文摘要